Imbalanced Gradients: A New Cause of Overestimated Adversarial Robustness
arXiv: Computer Vision and Pattern Recognition(2021)
Abstract
Evaluating the robustness of a defense model is a challenging task in adversarial robustness research. Obfuscated gradients, a type of gradient masking, have previously been found to exist in many defense methods and cause a false signal of robustness. In this paper, we identify a more subtle situation called \emph{Imbalanced Gradients} that can also cause overestimated adversarial robustness. The phenomenon of imbalanced gradients occurs when the gradient of one term of the margin loss dominates and pushes the attack towards to a suboptimal direction. To exploit imbalanced gradients, we formulate a \emph{Margin Decomposition (MD)} attack that decomposes a margin loss into individual terms and then explores the attackability of these terms separately via a two-stage process. We examine 12 state-of-the-art defense models, and find that models exploiting label smoothing easily cause imbalanced gradients, and on which our MD attacks can decrease their PGD robustness (evaluated by PGD attack) by over 23\%. For 6 out of the 12 defenses, our attack can reduce their PGD robustness by at least 9\%. The results suggest that imbalanced gradients need to be carefully addressed for more reliable adversarial robustness.
MoreTranslated text
Key words
robustness
AI Read Science
Must-Reading Tree
Example
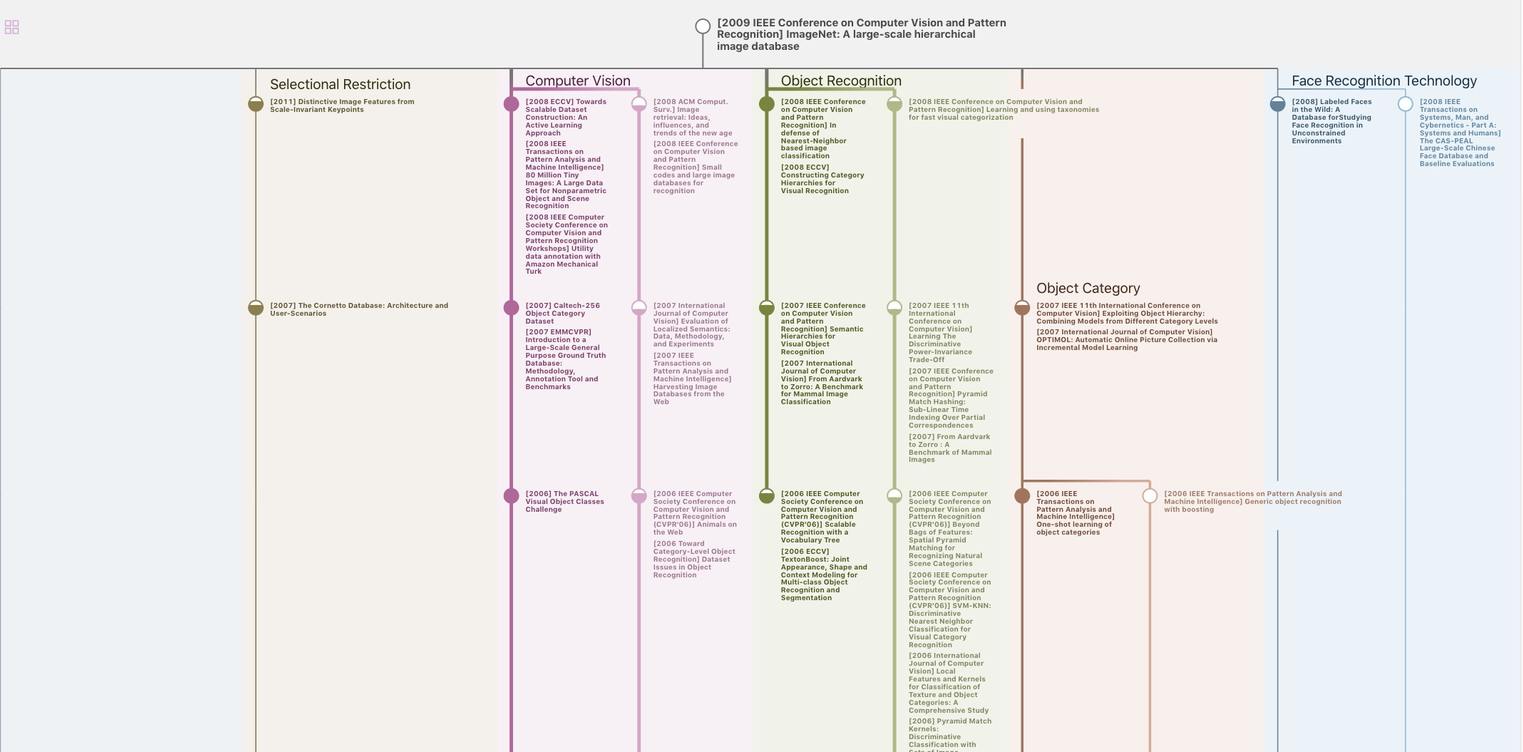
Generate MRT to find the research sequence of this paper
Chat Paper
Summary is being generated by the instructions you defined