Spatial Reliability Enhanced Correlation Filter: An Efficient Approach for Real-Time UAV Tracking
IEEE Transactions on Multimedia(2021)
摘要
Traditional discriminative correlation filter (DCF) has received great popularity due to its high computational efficiency. However, the lightweight framework of DCF cannot promise robust performance when the tracker faces appearance variations within the background. These unpredictable appearance variations always distract the filter. Most existing DCF-based trackers either utilize deep convolutional features or incorporate additional constraints to elevate tracking robustness. Despite some improvements, both of them hamper the tracking speed and can only roughly alleviate the distractions of appearance variations. In this paper, a novel spatial reliability enhanced learning strategy is proposed to handle the problems aforementioned. By monitoring the variation of response produced in detection phase, a dynamic reliability map is generated to indicate the reliability of each background subregion. Then, label adjustment is conducted to repress the distractions of these unreliable areas. Compared with the conventional way of constraint where a new term is always added to realize the desired goal, label adjustment is simultaneously more efficient and effective. Moreover, to promise the accuracy and dependability of the reliability map, an adaptively updated response pool recording reliable historical response values is proposed. Extensive and exhaustive experiments on three challenging unmanned aerial vehicle (UAV) benchmarks, i.e., UAV123@10fps, DTB70 and UAVDT, which totally include 243 video sequences, validate the superiority of the proposed method against other state-of-the-art trackers and exhibit a remarkable generality in a variety of scenarios. Meanwhile, the tracking speed of 65.2FPS on a cheap CPU makes it suitable for real-time UAV applications.
更多查看译文
关键词
UAV tracking,spatial reliability map,response pool,label adjustment,correlation filter,appearance variation
AI 理解论文
溯源树
样例
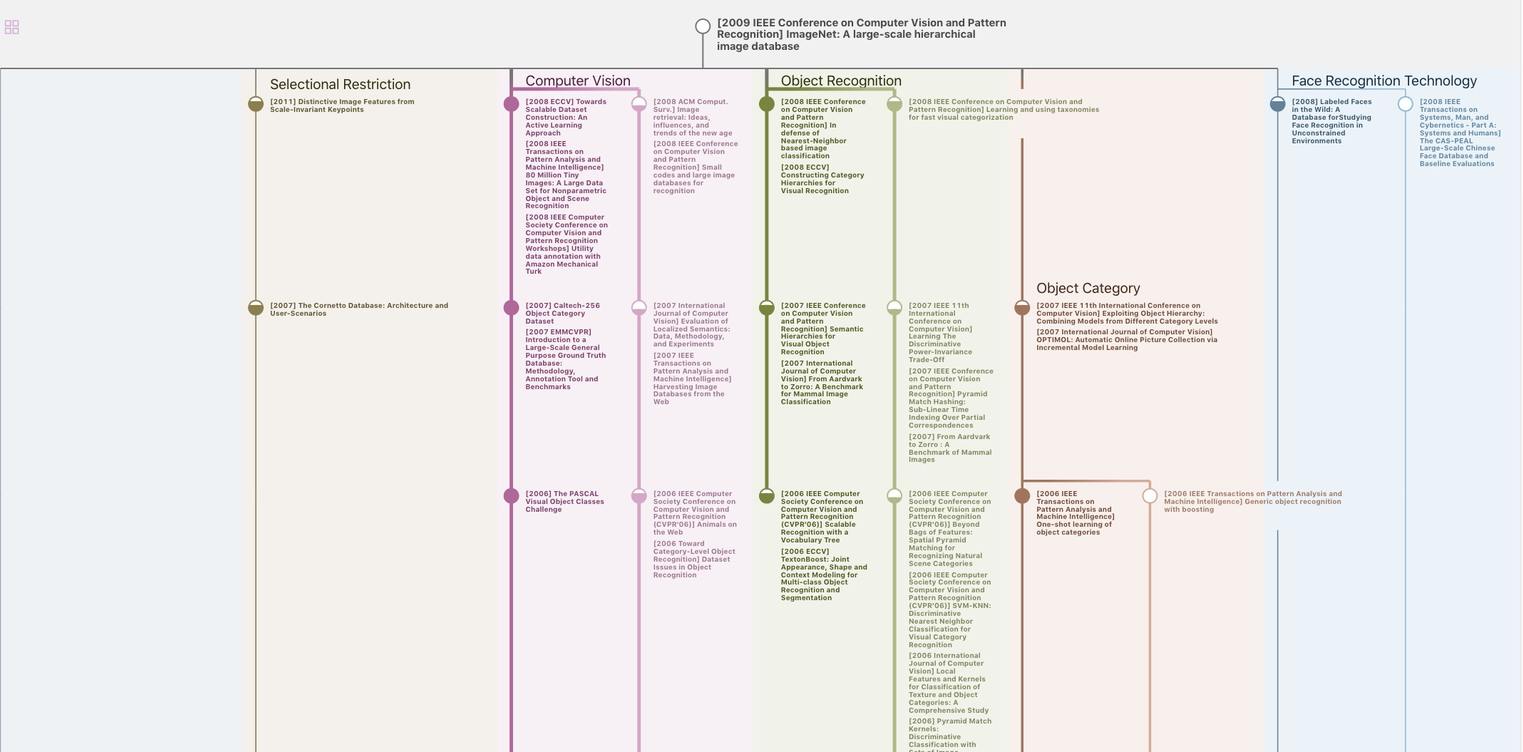
生成溯源树,研究论文发展脉络
Chat Paper
正在生成论文摘要