Feature Cloud: Improving Deep Visual Recognition With Probabilistic Feature Augmentation
IEEE Transactions on Circuits and Systems for Video Technology(2022)
摘要
This paper considers deep visual recognition on long-tailed data. Under the long-tailed distribution, a small portion of the classes (head classes) occupy most training samples and the most classes (tail classes) only occupy relatively few samples. We observe that such long-tailed distribution significantly distorts the deeply-learned feature space, which consequentially compromises the deep visual recognition. Specifically, during training, each head class is prone to a relatively wide spatial distribution in the deep feature space, while each tail class is prone to a relatively small spatial distribution. In another word, the tail classes usually have much smaller spatial distribution than the head classes, distorting the overall feature space. In response, we propose to explicitly inflate the distribution of each tail class in the deep feature space, so that the tail classes will have comparable distribution range as the head classes. To this end, we replace each tail feature vector with a set of feature vectors on the fly. These feature vectors follow a probabilistic distribution learned from the head classes and yield a “feature cloud” surrounding the original tail feature. We show that the feature cloud effectively transfers the within-class diversity from the head classes onto the tail classes, maintaining an effect of probabilistic feature augmentation. An important advantage of the proposed feature cloud is that it is capable to bring general improvement to long-tailed visual recognition on two fundamental tasks,
i.e.
, deep classification and deep representation learning, in spite of the significant differences between them. Extensive experiments on both deep metric learning benchmarks and deep image classification benchmarks validate the effectiveness of the proposed feature cloud.
更多查看译文
关键词
Long-tailed distribution,data augmentation,deep visual recognition
AI 理解论文
溯源树
样例
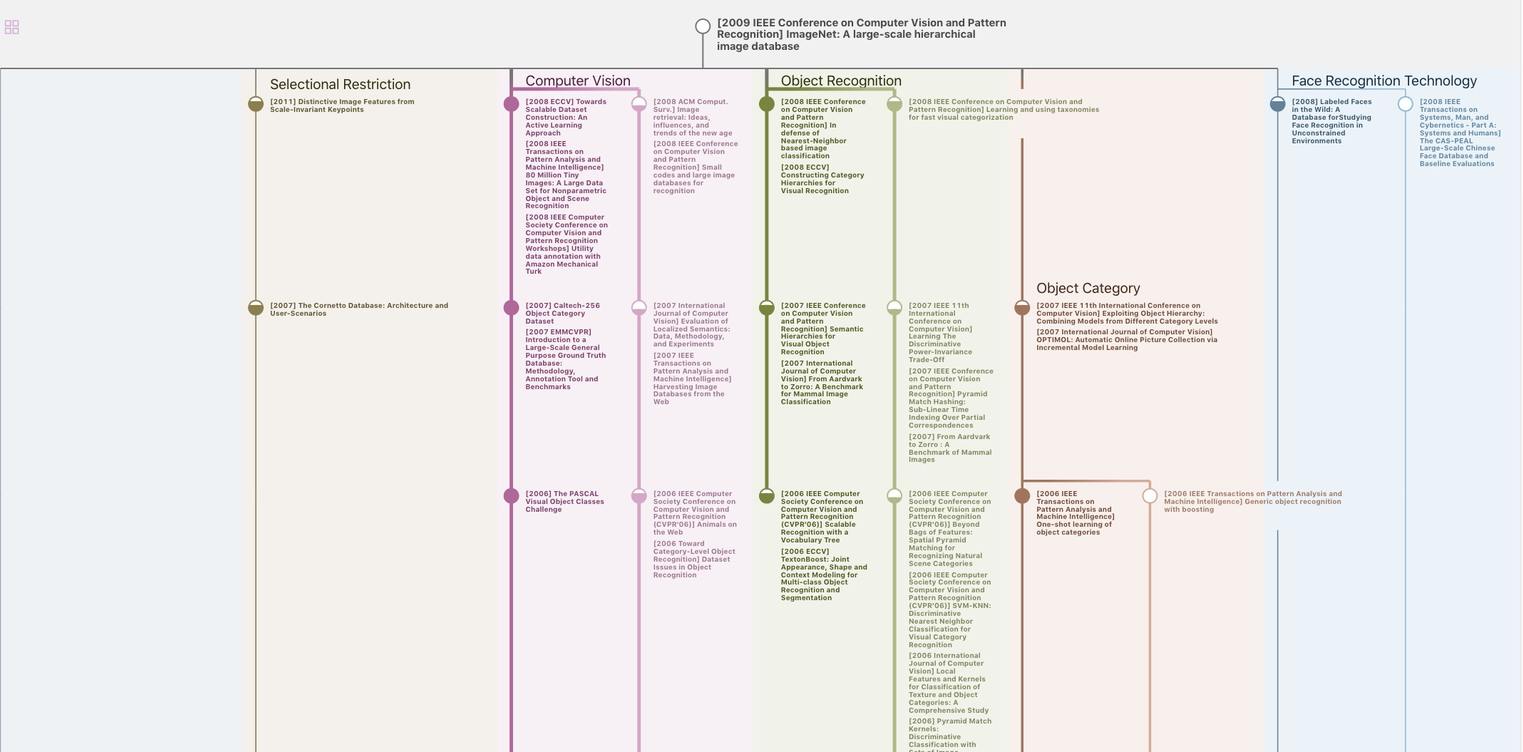
生成溯源树,研究论文发展脉络
Chat Paper
正在生成论文摘要