Label Enhancement by Maintaining Positive and Negative Label Relation
IEEE Transactions on Knowledge and Data Engineering(2021)
摘要
Label distribution learning (LDL) is a novel machine learning paradigm that gives the description degree of each label to a particular instance. However, many existing datasets contain only simple logical labels since it is difficult and time-consuming to directly obtain the label distributions. Therefore, label enhancement (LE) is proposed to convert multi-label datasets consisting of logical labels into label distribution datasets. Recently, many LE algorithms have been proposed and most of them concentrate on the fitting degree, but ignore the ordering relation between positive and negative labels. Therefore, in this paper, we propose an LE algorithm based on maintaining positive and negative label relation, which contains a novel ranking loss that can generate different penalties according to different ranking errors. Our algorithm achieves a good balance between the degree of fitting and the ordering relation. The experimental results on several real-world datasets validate the effectiveness of our method.
更多查看译文
关键词
Label enhancement,label distribution learning
AI 理解论文
溯源树
样例
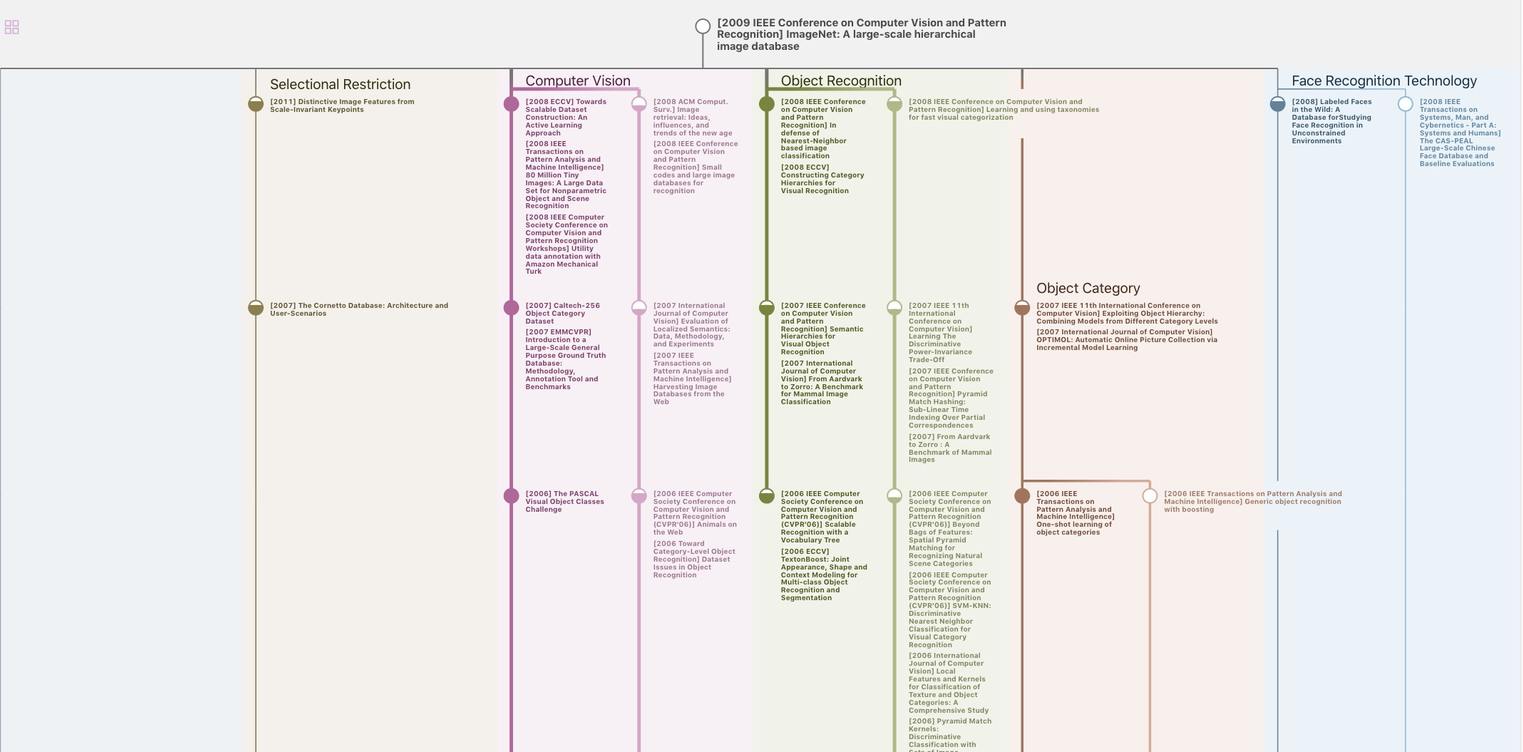
生成溯源树,研究论文发展脉络
Chat Paper
正在生成论文摘要