Shallow or Deep? Detecting Anomalous Flows in the Canadian Automated Clearing and Settlement System using an Autoencoder
Social Science Research Network(2020)
摘要
Financial market infrastructures and their participants play a crucial role in the economy. Financial or operational challenges faced by one participant can have contagion effects and pose risks to the broader financial system. Our paper applies (deep) neural networks (autoencoder) to detect anomalous flows from payments data in the Canadian Automated Clearing and Settlement System (ACSS) similar to Triepels et al. (2018). We evaluate several neural network architecture setups based on the size and number of hidden layers, as well as differing activation functions dependent on how the input data was normalized. As the Canadian financial system has not faced bank runs in recent memory, we train the models on “normal” data and evaluate out-of-sample using test data based on historical anomalies as well as simulated bank runs. Our out-of-sample simulations demonstrate the autoencoder’s performance in different scenarios, and results suggest that the autoencoder detects anomalous payment flows reasonably well. Our work highlights the challenges and trade-offs in employing a workhorse deep-learning model in an operational context and raises policy questions around how such outlier signals can be used by the system operator in complying with the prominent payment systems guidelines and by financial stability experts in assessing the impact on the financial system of a financial institution that shows extreme behaviour.
更多查看译文
关键词
canadian automated clearing,autoencoder,settlement system,anomalous flows
AI 理解论文
溯源树
样例
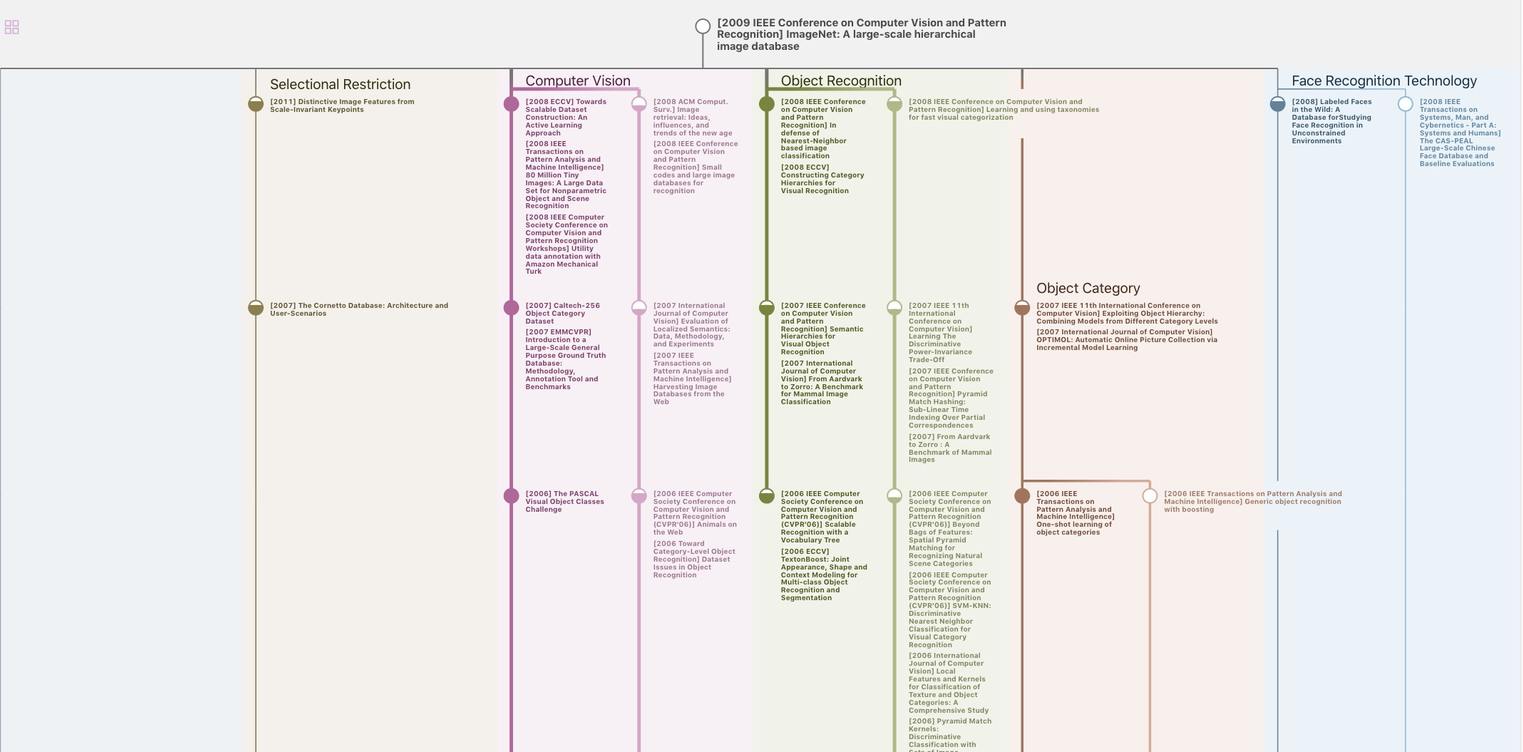
生成溯源树,研究论文发展脉络
Chat Paper
正在生成论文摘要