SAR Imaging and Despeckling Based on Sparse, Low-Rank, and Deep CNN Priors
IEEE GEOSCIENCE AND REMOTE SENSING LETTERS(2022)
摘要
Synthetic aperture radar (SAR) generally suffers from enormous strains from large quantities of sampling data and serious interferences from the speckle noise. This letter proposes a novel deep network to address these problems. By utilizing the prior knowledge in a more reasonable way, the proposed network could realize SAR imaging and despeckling with down-sampled data simultaneously. Specifically, we decompose the SAR image in the SAR imaging-despeckling observation model into a sparse matrix and a low-rank matrix, and then establish an optimization problem with the corresponding sparse and low-rank priors. Moreover, the deep convolutional neural networks (CNN) denoiser prior is also introduced to further improve the speckle reduction capability. Then, we devise a deep network called SLRCP-Net to solve this problem. Experiments conducted on real Radarsat-1 down-sampled data demonstrate the validity of SLRCP-Net in SAR imaging and speckle suppression.
更多查看译文
关键词
Radar polarimetry,Speckle,Synthetic aperture radar,Optimization,Sparse matrices,Imaging,Convolutional neural networks,Deep learning,down-sampled raw data,image despeckling,sparse imaging,synthetic aperture radar
AI 理解论文
溯源树
样例
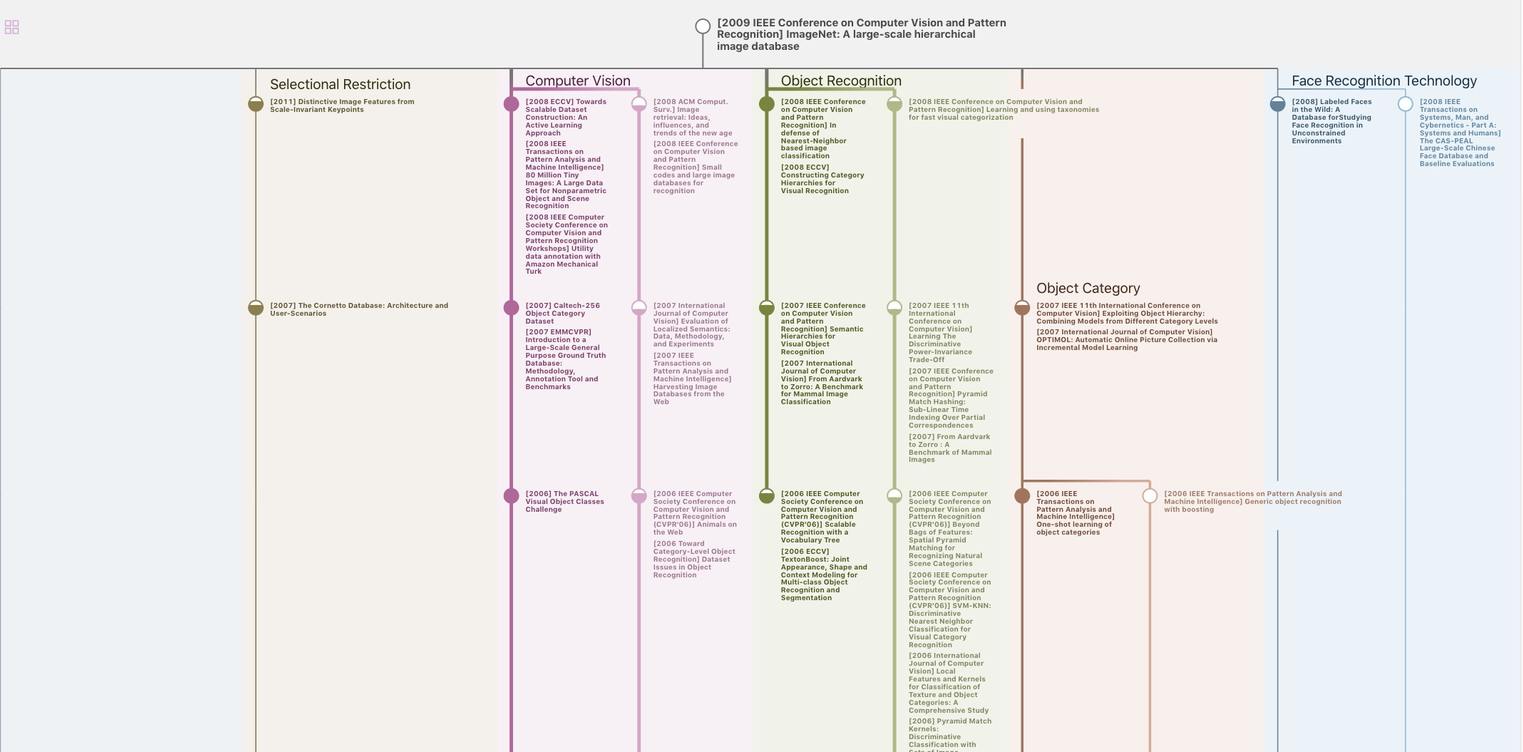
生成溯源树,研究论文发展脉络
Chat Paper
正在生成论文摘要