Do Common Educational Datasets contain Static Information? A Statistical Study
educational data mining(2021)
摘要
In Intelligent Tutoring Systems, methods to choose the next exercise for a student are inspired from generic recommender systems used for instance in online shopping or multimedia recommendation. As such, collaborative filtering and especially matrix factorization is often included as a part of recommendation algorithms in ITS.One notable difference in ITS is the rapid evolution of the users who are improving their performance as opposed to multimedia recommendation where preferences are more static.This raises the following question: how reliably can we use matrix factorization, a tool tried and tested in a static environment, in a context where timelines seem of importance.In this article we tried to quantify empirically how much information can be extracted statically from datasets in educations versus datasets in multimedia, as the quality of such information is critical to be able to accurately make predictions and recommendations.We found that educational datasets contain less static information compared to multimedia sets, to the point that vectors of higher dimensions only marginally increase the precision of the matrix factorization compared to a 1-dimensional characterization. These results show that educational datasets must be used with time information and warns about the dangers of trying to directly use existing algorithms developped for static datasets.
更多查看译文
AI 理解论文
溯源树
样例
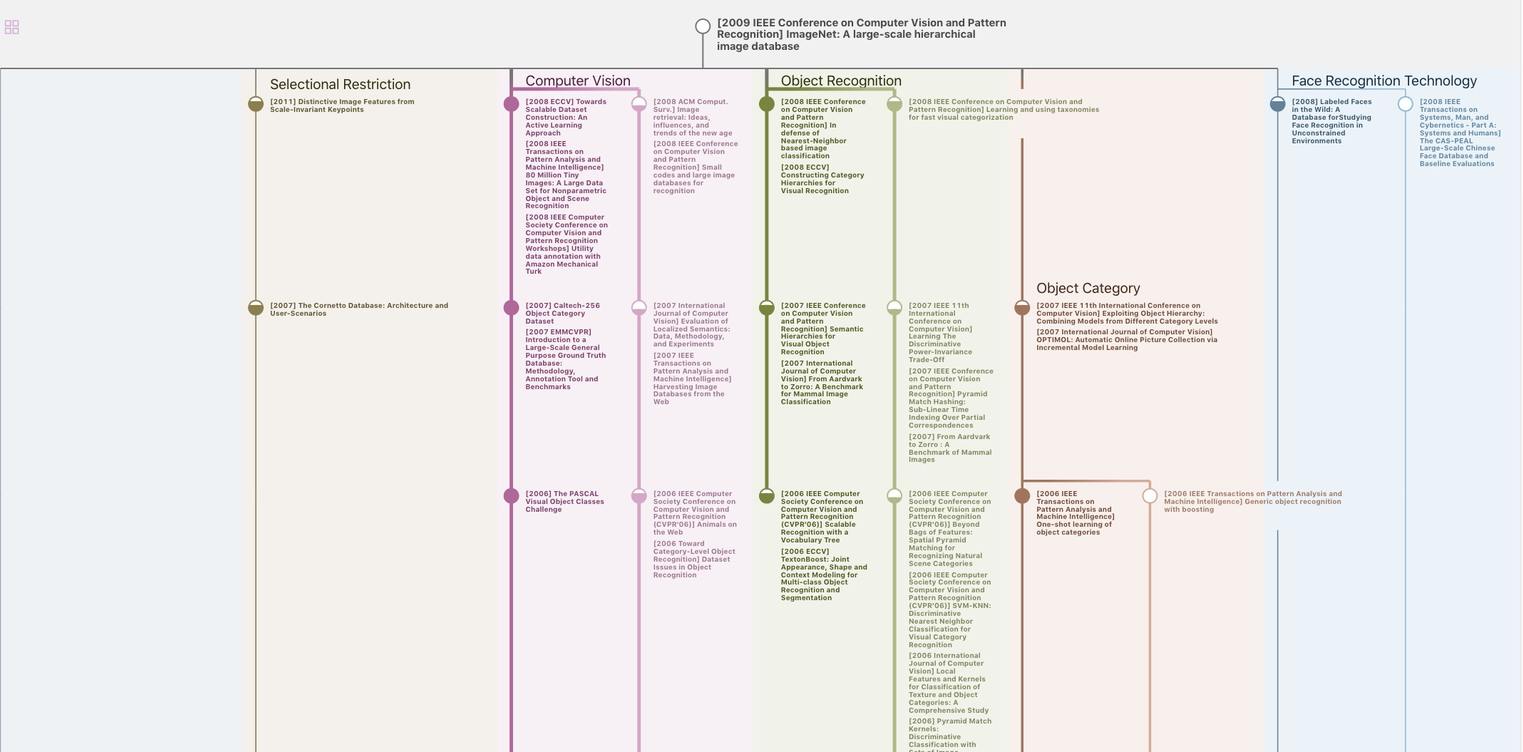
生成溯源树,研究论文发展脉络
Chat Paper
正在生成论文摘要