Rationalizing Translation Elongation by Reinforcement Learning
Social Science Research Network(2020)
摘要
Translation elongation is a crucial phase during protein biosynthesis. In this study, we develop a novel deep reinforcement learning based framework, named Riboexp, to model the uneven distribution of ribosomes on mRNA transcripts during translation elongation. In particular, Riboexp employs a policy network to perform a context-dependent feature selection for the prediction of ribosome density. Our extensive tests demonstrate that Riboexp can outperform other state-of-the-art methods in predicting ribosome density by up to 5.9% in per-gene Pearson correlation coefficient. Enabled by the reinforcement learning strategy, Riboexp can identify more relevant features for the prediction task than other commonly used attribution methods in deep learning. In-depth analyses also reveal the meaningful biological insights generated by the Riboexp framework. Moreover, the application of Riboexp in codon optimization results in an increase of protein production by around 31% over the previous state-of-the-art method that models ribosome density. These results have established Riboexp as a powerful and useful computational tool in the studies of translation dynamics and protein synthesis.
更多查看译文
关键词
translation elongation,reinforcement learning
AI 理解论文
溯源树
样例
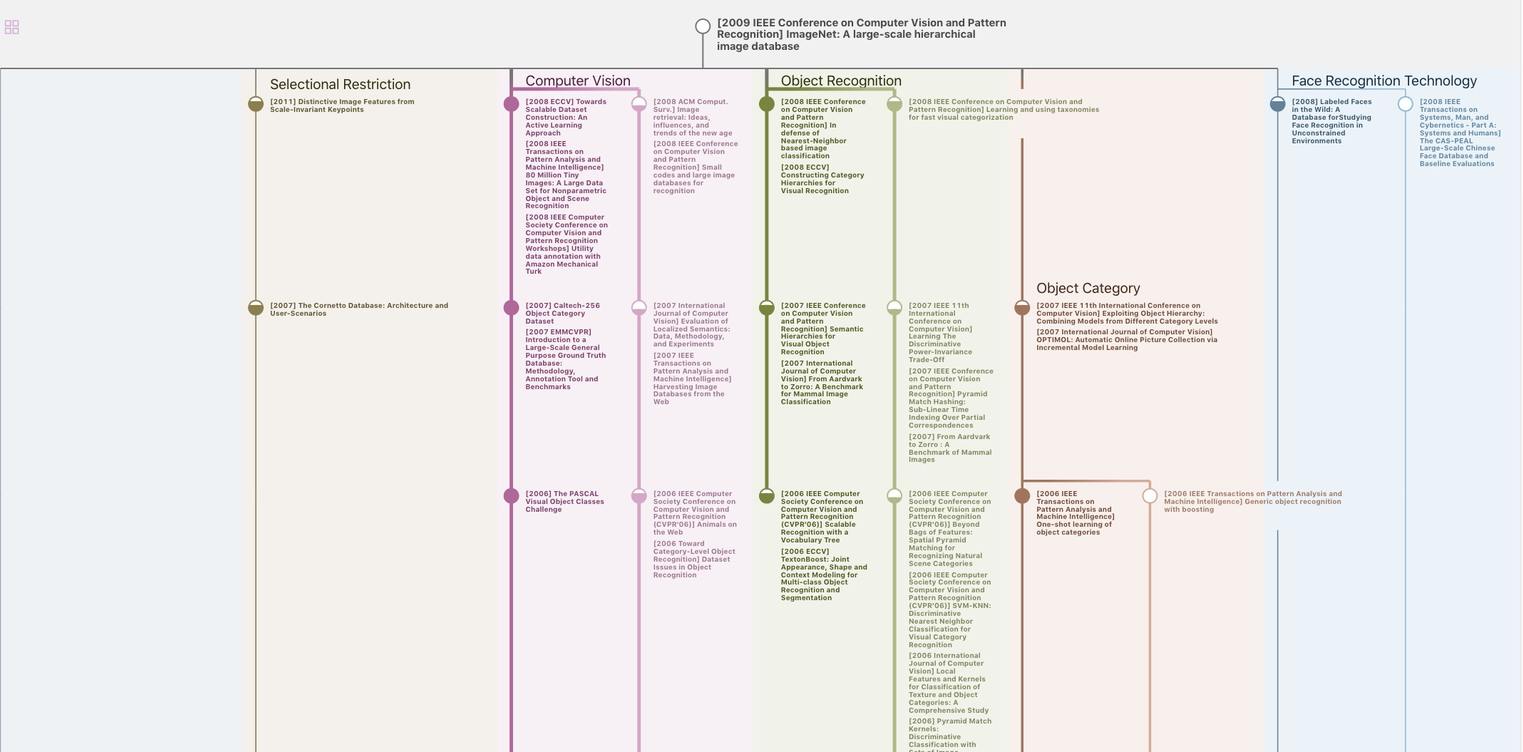
生成溯源树,研究论文发展脉络
Chat Paper
正在生成论文摘要