Estimation of downhole cuttings concentration from experimental data – Comparison of empirical and fuzzy logic models
Journal of Petroleum Science and Engineering(2022)
摘要
Proper selection of drilling parameters during planning phase to ensure an adequately clean well is the key to avoid costly drilling operational problems such as mechanical stuck pipe and high drillstring torque and drag resulting in not reaching the drilling target for highly deviated/ERD wells. Cuttings transport is a complex phenomenon influenced by different parameters such as flow rate, drillstring rotation, rate of penetration, eccentricity, well configuration, drilling fluid properties and cuttings properties. The complexity of the cuttings transport process has led to the use of alternative modeling approaches. In this paper, a computational intelligence method, fuzzy logic (FL), is chosen to develop a model to estimate downhole cuttings concentration. FL, resembling human reasoning, is capable of modeling non-linear functions of arbitrary complexity and dealing with imprecision in data. The FL model developed in the current work is based on 509 experimental observations involving 11 independent test parameters each. 25% of the collected dataset (excluding the test dataset) is used as cross-validation dataset and the rest is used as training dataset to avoid overfitting. The test dataset comprised of nine observations is used for comparing FL model estimates for downhole cuttings concentration to those made by two published empirical models which are based on dimensional analysis and critical drilling fluid velocity concept respectively. Comparison of the models on the test dataset shows that the developed FL model performs better than the two empirical models in all the three statistical measurements namely coefficient of determination (R2), root mean square error (RMSE) and mean absolute error (MAE).
更多查看译文
关键词
Downhole cuttings concentration,Computational intelligence,Fuzzy logic modeling,Empirical models,Experimental data
AI 理解论文
溯源树
样例
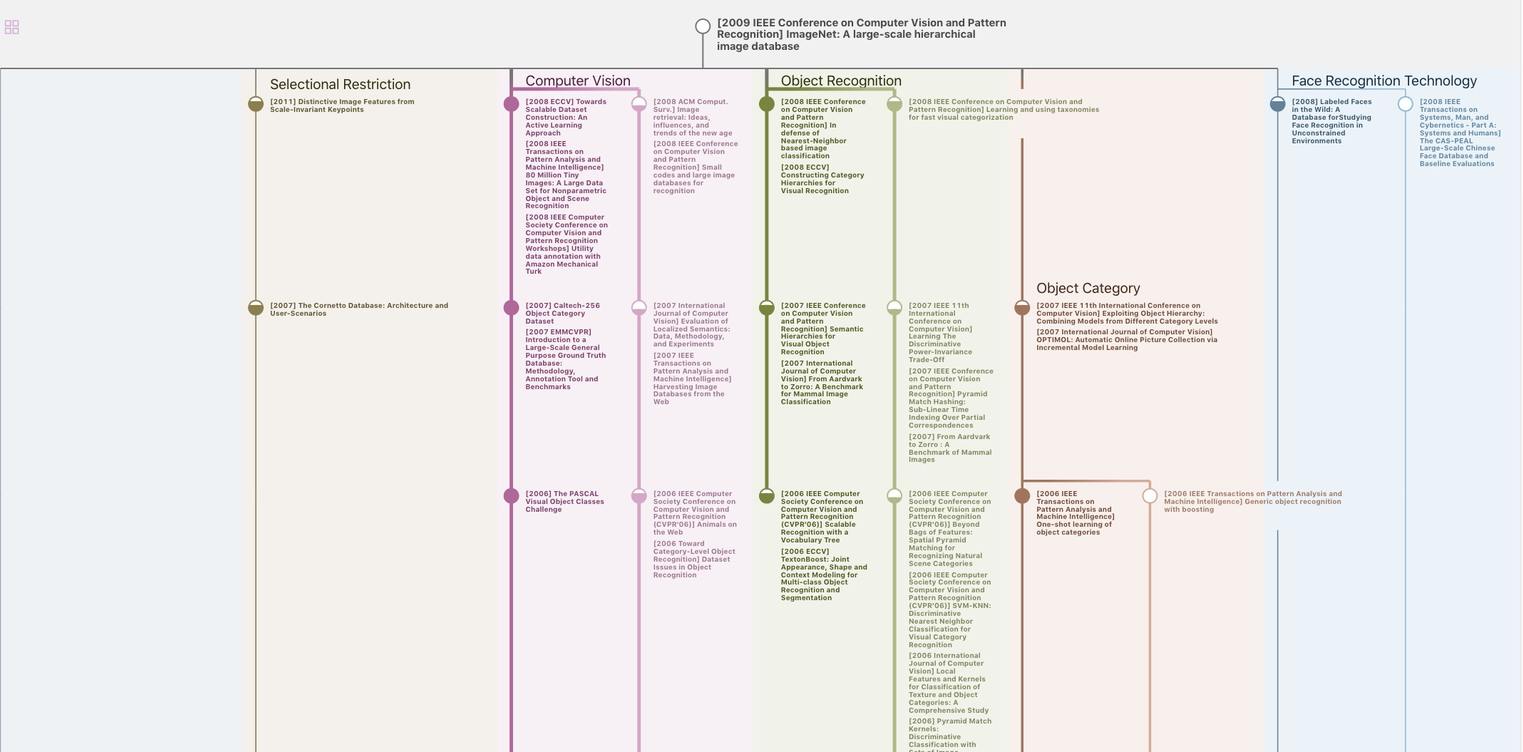
生成溯源树,研究论文发展脉络
Chat Paper
正在生成论文摘要