Artificial intelligence-based fault prediction framework for WBAN
Journal of King Saud University - Computer and Information Sciences(2022)
摘要
Wireless Body Area Networks (WBAN) can provide continuous monitoring of patients’ health. Such monitoring can be a decisive factor in health and death situations. Fault management in WBANs is a key reliability component to make it socially acceptable and to overcome pertained challenges such as unpredicted faults, massive data streaming, and detection accuracy. Failures in fault detection due to hardware, software, and network issues may put human lives at risk. This paper focuses on detecting and predicting faults in sensors in the context of a WBAN. A framework is proposed to manage AI-based prediction models and fault detection using thresholds where four Machine learning techniques: Artificial Neural Networks (ANN), Deep Neural Networks (DNN), Support Vector Machines (SVM), and Decision Trees (DT), are used. The framework also provides alarm notifications, prediction model deployment, version control, and sensing node profiling. As a proof of concept, a fault management prototype is implemented and validated. The prototype classifies faults, manages automation of sensing node profiling, training, and validation of new models. The obtained experimental results show an accuracy greater than 96% for detecting faults with an inferior false alarm rate.
更多查看译文
关键词
WBAN,Machine learning,Fault management,Fault prediction,Sensor health packets
AI 理解论文
溯源树
样例
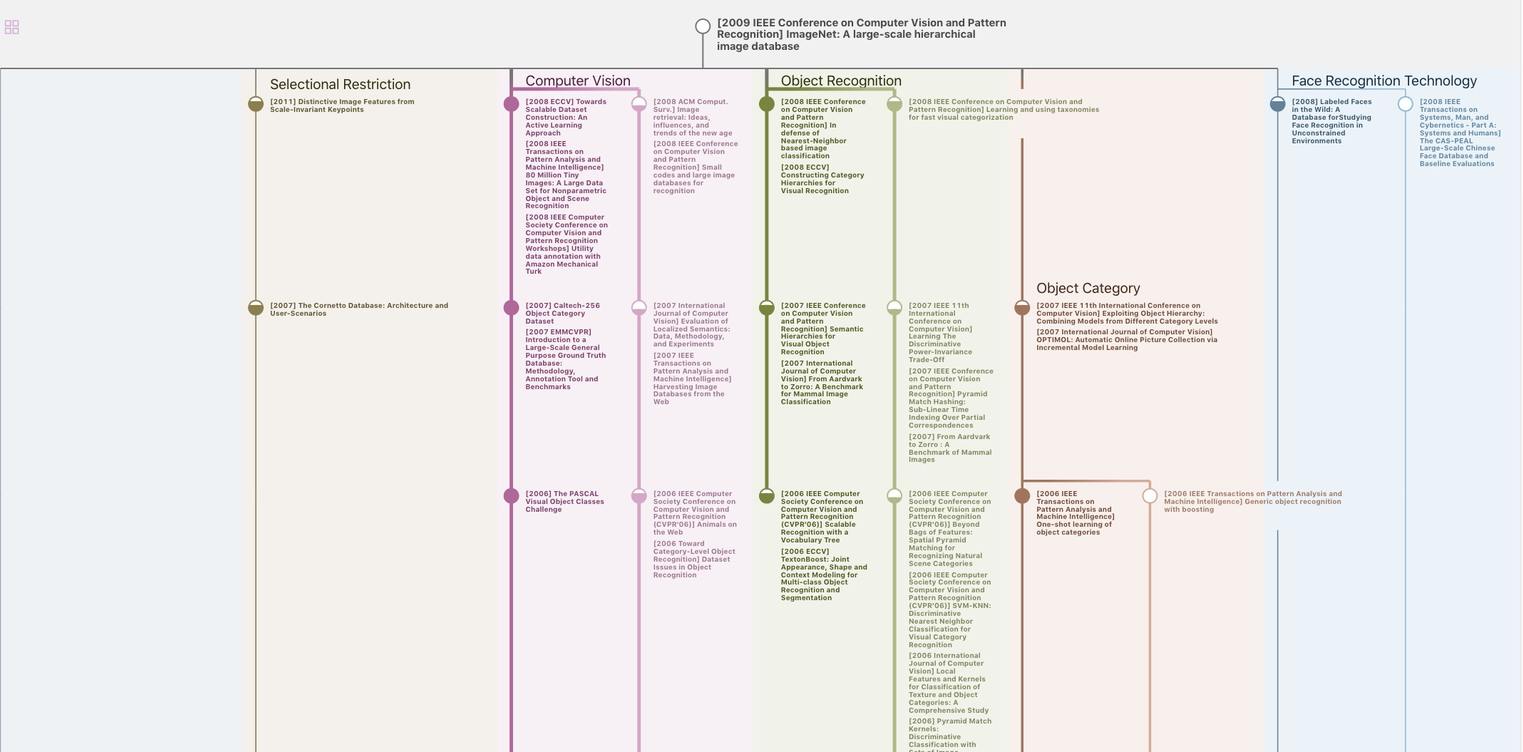
生成溯源树,研究论文发展脉络
Chat Paper
正在生成论文摘要