Exploiting Auxiliary Information for Improved Underwater Target Classification with Convolutional Neural Networks
Global Oceans 2020: Singapore – U.S. Gulf Coast(2020)
摘要
This work deals with the classification of objects as targets or clutter in synthetic aperture sonar (SAS) imagery using convolutional neural networks (CNNs). First, a new image-annotation tool is developed that allows extra auxiliary information (beyond the basic binary label) to be easily recorded about a given input image. The additional information consists of an estimate of the image quality; the local background environment; and for targets, the specific object shape, orientation, and length. The architecture of the CNNs - specifically the final dense layer and output layer - is then modified so that these extra quantities are additional outputs to be predicted simultaneously. As such, the task of the augmented CNNs becomes to provide a richer representation of an image beyond the binary label. This more complete operational picture can then better inform subsequent mine countermeasures (MCM) decisions. Experiments on a set of real, measured SAS data collected at sea demonstrate that tiny CNNs can accurately predict the additional auxiliary qualities without suffering a significant drop in binary classification performance.
更多查看译文
关键词
binary classification performance,target classification,convolutional neural networks,synthetic aperture sonar imagery,image-annotation tool,extra auxiliary information,basic binary label,input image,image quality,local background environment,specific object shape,output layer,augmented CNN
AI 理解论文
溯源树
样例
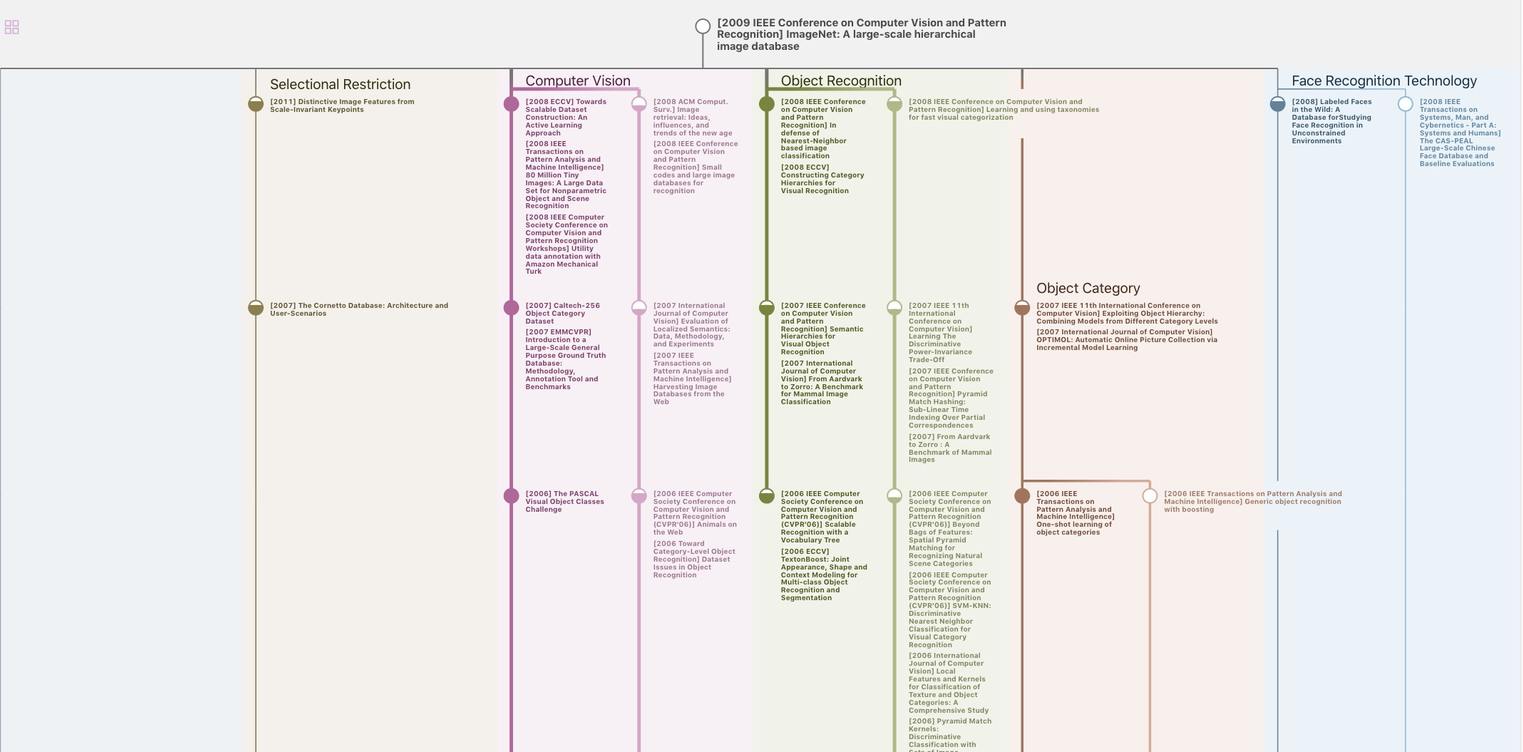
生成溯源树,研究论文发展脉络
Chat Paper
正在生成论文摘要