Glaucomanet: A Deep-Learning Algorithm for the Diagnosis of Primary Open-Angle Glaucoma from Fundus Photographs
Social Science Research Network(2021)
摘要
Background: Primary open-angle glaucoma (POAG) is one of the leading causes of irreversible blindness in the United States and worldwide. Although deep learning methods have been proposed to diagnose POAG, it remains challenging to develop a robust and explainable algorithm to facilitate the downstream diagnostic tasks automatically.
Methods: We proposed a deep learning model, GlaucomaNet, with joint fusion to identify POAG from fundus photographs. GlaucomaNet consists of two neural networks to simulate the human grading process: one performing preliminary grading and the other detailed grading. An ensemble of network architectures further improved diagnostic accuracy. GlaucomaNet was evaluated on two independent datasets. The performance was measured by AUC, accuracy, precision, sensitivity, specificity, and F1-score.
Findings: GlaucomaNet was trained and evaluated on two datasets: 37,339 fundus photographs from the Ocular Hypertension Treatment Study (OHTS), and 4,855 fundus photographs from the Large-scale Attention-based Glaucoma (LAG) dataset. GlaucomaNet achieved superior performance to six state-of-the-art neural networks on OHTS, with accuracy of 0.930 (standard deviation, 0.010), F1-score of 0.490 (0.033), and AUC of 0.904 (0.014). It also outperformed the baselines on the LAG dataset.
Interpretations: GlaucomaNet demonstrated high accuracy with increased transparency in POAG diagnosis. It is also applicable for two well-known challenges: image data diversity and POAG only due to visual fields abnormalities. These results highlight the potential of deep learning to assist and enhance clinical POAG diagnosis. GlaucomaNet is publicly available on https://github.com/bionlplab/GlaucomaNet. Funding: This project was supported by the National Library of Medicine (4R00LM013001), the National Eye Institute, the National Center on Minority Health and Health Disparities (EY09341, EY09307) at NIH, Horncrest Foundation, the Department of Ophthalmology and Visual Sciences at Washington University, the NIH Vision Core Grant (P30EY02687), Merck Research Laboratories, Pfizer, Inc., White House Station, New Jersey, and unrestricted grants from Research to Prevent Blindness, Inc., New York, NY.
Declaration of Interest: Ethical Approval: The first dataset is obtained from the Ocular Hypertension Treatment Study (OHTS). The OHTS comes from 22 centers in the United States. The study protocol was approved by an independent Institutional Review Board at each clinical center
更多查看译文
AI 理解论文
溯源树
样例
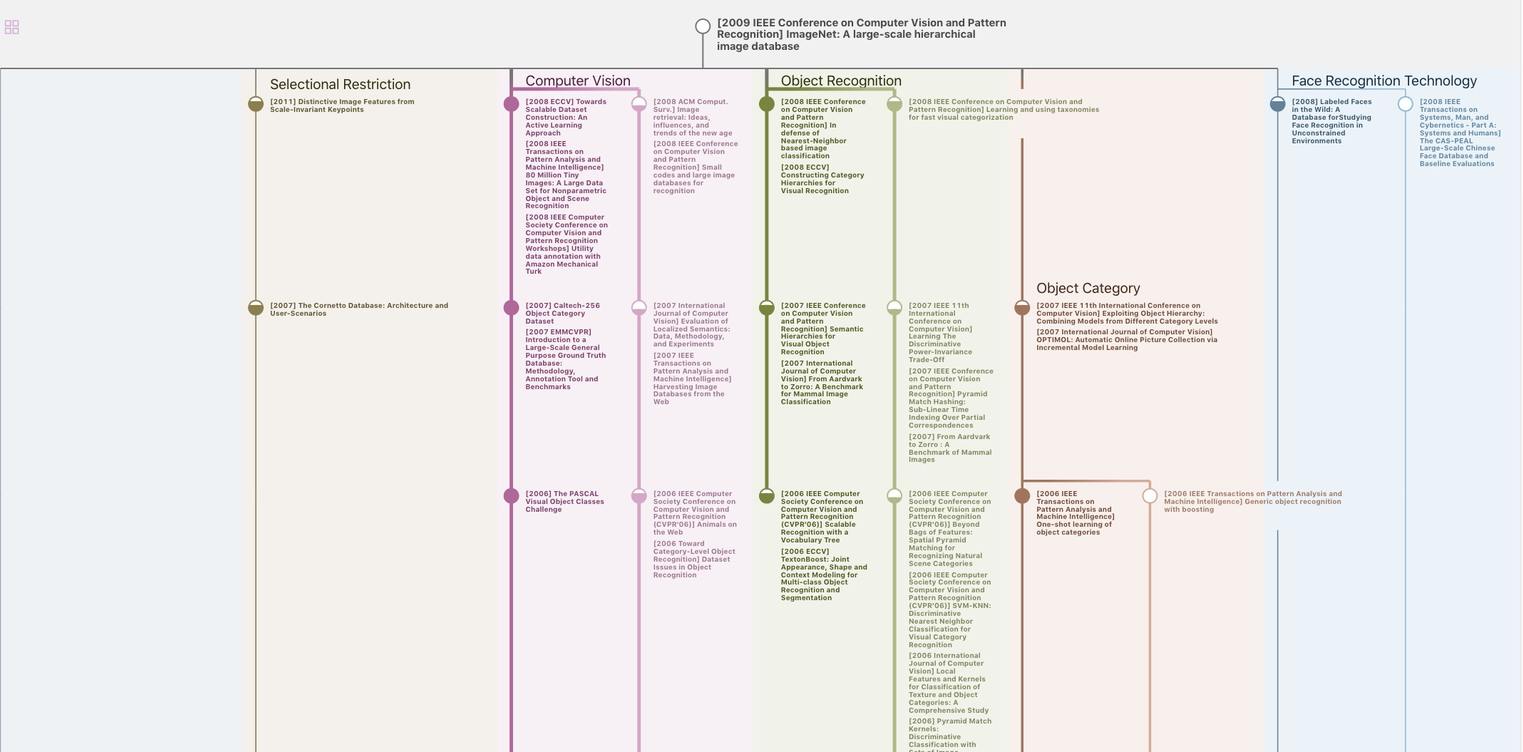
生成溯源树,研究论文发展脉络
Chat Paper
正在生成论文摘要