Data Anonymization with Diversity Constraints
IEEE Transactions on Knowledge and Data Engineering(2021)
摘要
Recent privacy legislation has aimed to restrict and control the amount of personal data published by companies and shared with third parties. Much of this real data is not only sensitive requiring anonymization but also contains characteristic details from a variety of individuals. This diversity is desirable in many applications ranging from Web search to drug and product development. Unfortunately, data anonymization techniques have largely ignored diversity in its published result. This inadvertently propagates underlying bias in subsequent data analysis. We study the problem of finding a diverse anonymized data instance where diversity is measured via a set of diversity constraints. We formalize diversity constraints, and study their fundamental problems of satisfiability, implication, and validation. We show that determining the existence of a diverse, anonymized instance can be done in PTIME, and we present a clustering-based algorithm, along with optimizations to improve performance. We conduct extensive experiments using real and synthetic data showing the effectiveness of our techniques, and improvement over existing baselines. Our work aligns with recent trends towards responsible data science by coupling diversity with privacy-preserving data publishing.
更多查看译文
关键词
Data anonymization,data diversity,diversity constraints
AI 理解论文
溯源树
样例
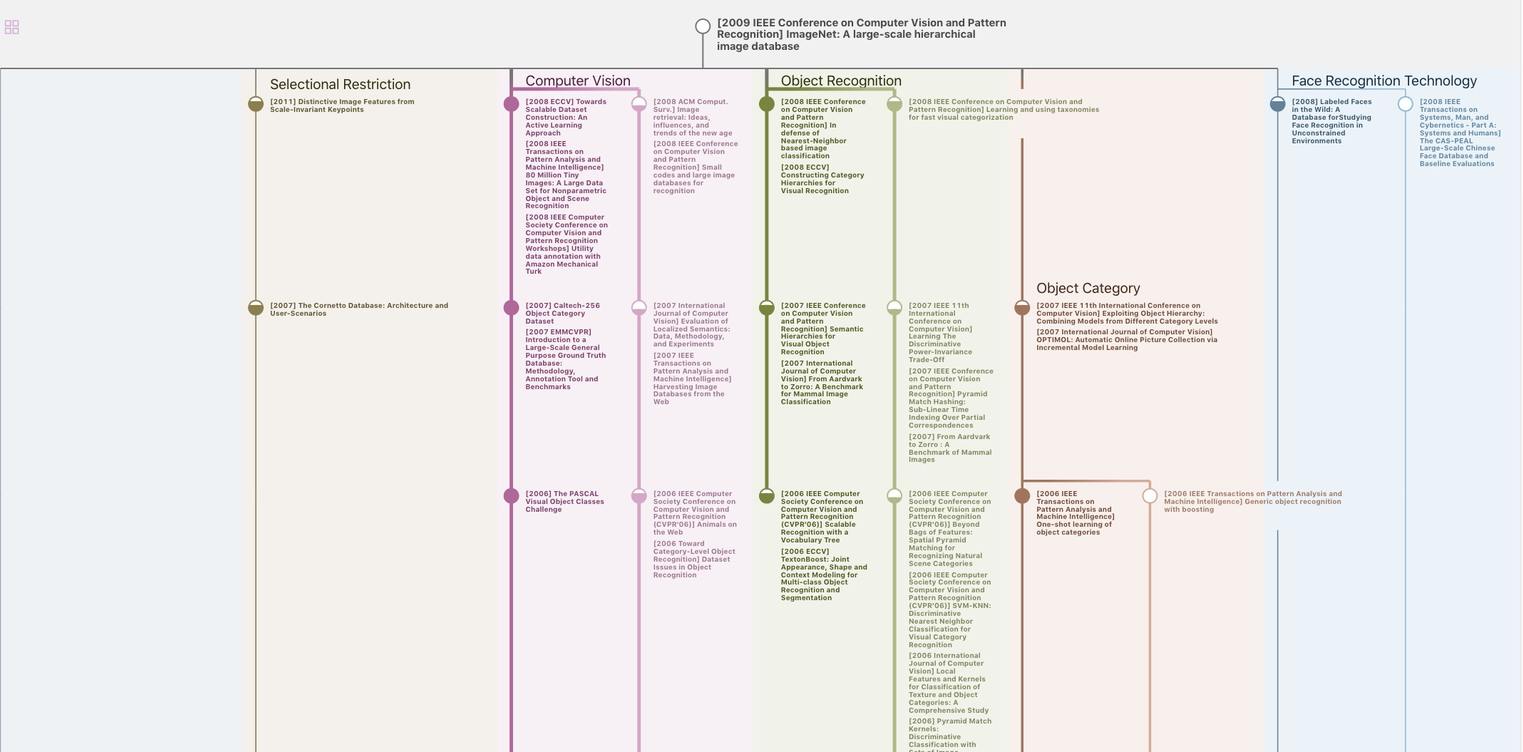
生成溯源树,研究论文发展脉络
Chat Paper
正在生成论文摘要