Geodesic Multi-Modal Mixup for Robust Fine-Tuning
arxiv(2022)
摘要
Pre-trained large-scale models provide a transferable embedding, and they show promising performance on diverse downstream tasks. However, the analysis of learned embedding has not been explored well, and the transferability for cross-modal tasks can be improved. This paper provides a perspective to understand multi-modal embedding in terms of uniformity and alignment. We newly find that the representation learned by multi-modal learning models such as CLIP has two separated embedding spaces for each heterogeneous dataset with less alignment. Besides, there are unexplored large intermediate areas between the two modalities with less uniformity. As a result, lack of alignment and uniformity might restrict the robustness and transferability of the representation for the downstream task. To this end, we provide a new end-to-end fine-tuning method for robust representation that encourages better uniformity and alignment score. First, we propose a \textit{Geodesic Multi-Modal Mixup} that mixes the representation of image and text to generate the hard negative samples on the hyperspherical embedding space. Second, we fine-tune the multi-modal model on hard negative samples as well as normal negatives and positive samples with contrastive loss. Through extensive experiments on retrieval, classification, and structure-awareness task, we demonstrate that our geodesic multi-modal Mixup learns a robust representation and provides improved performance on various downstream tasks.
更多查看译文
AI 理解论文
溯源树
样例
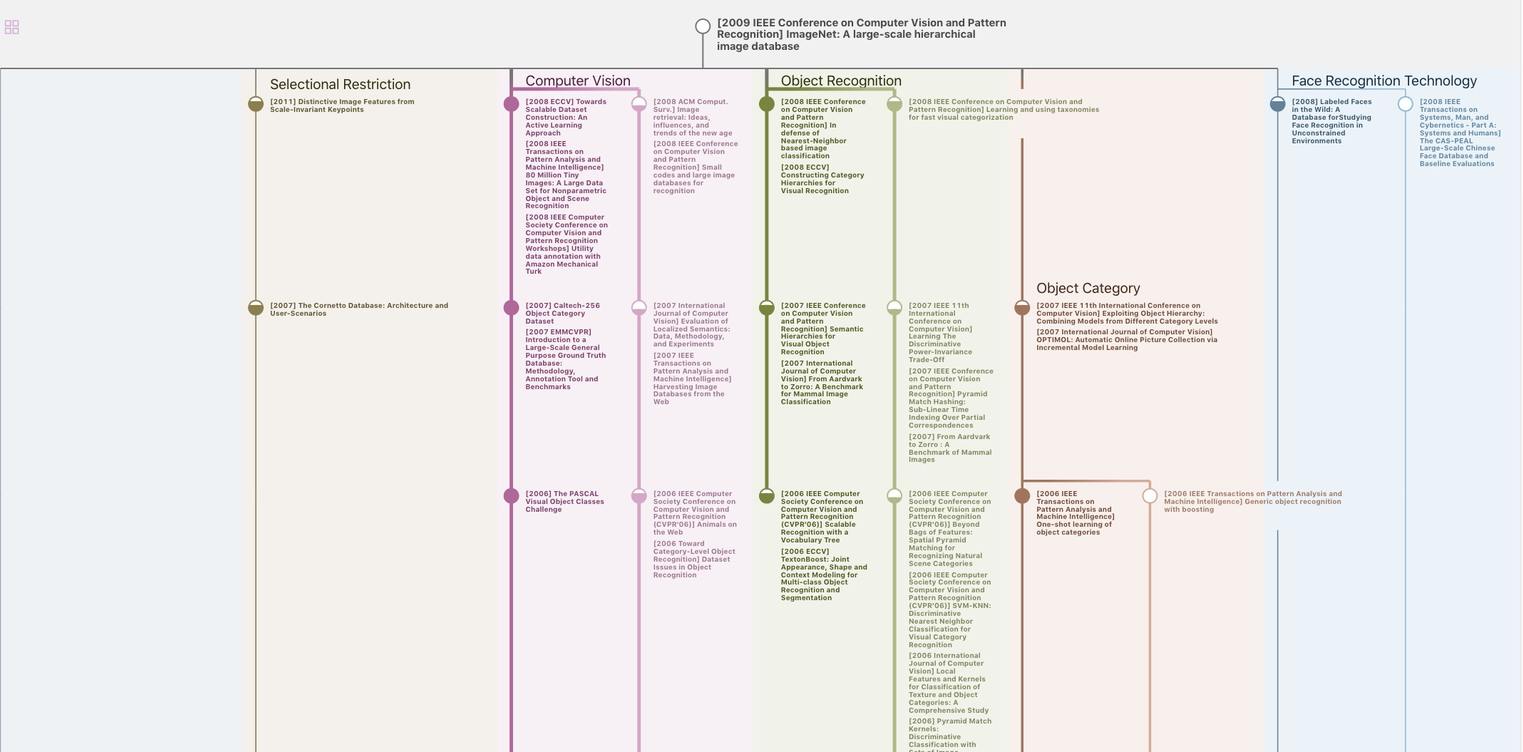
生成溯源树,研究论文发展脉络
Chat Paper
正在生成论文摘要