AgraSSt: Approximate Graph Stein Statistics for Interpretable Assessment of Implicit Graph Generators
NeurIPS 2022(2022)
摘要
We propose and analyse a novel statistical procedure, coined AgraSSt, to assess the quality of graph generators that may not be available in explicit form. In particular, AgraSSt can be used to determine whether a learnt graph generating process is capable of generating graphs that resemble a given input graph. Inspired by Stein operators for random graphs, the key idea of AgraSSt is the construction of a kernel discrepancy based on an operator obtained from the graph generator. AgraSSt can provide interpretable criticisms for a graph generator training procedure and help identify reliable sample batches for downstream tasks. Using Stein`s method we give theoretical guarantees for a broad class of random graph models. We provide empirical results on both synthetic input graphs with known graph generation procedures, and real-world input graphs that the state-of-the-art (deep) generative models for graphs are trained on.
更多查看译文
关键词
Stein's method,kernel method,synthetic graph generator
AI 理解论文
溯源树
样例
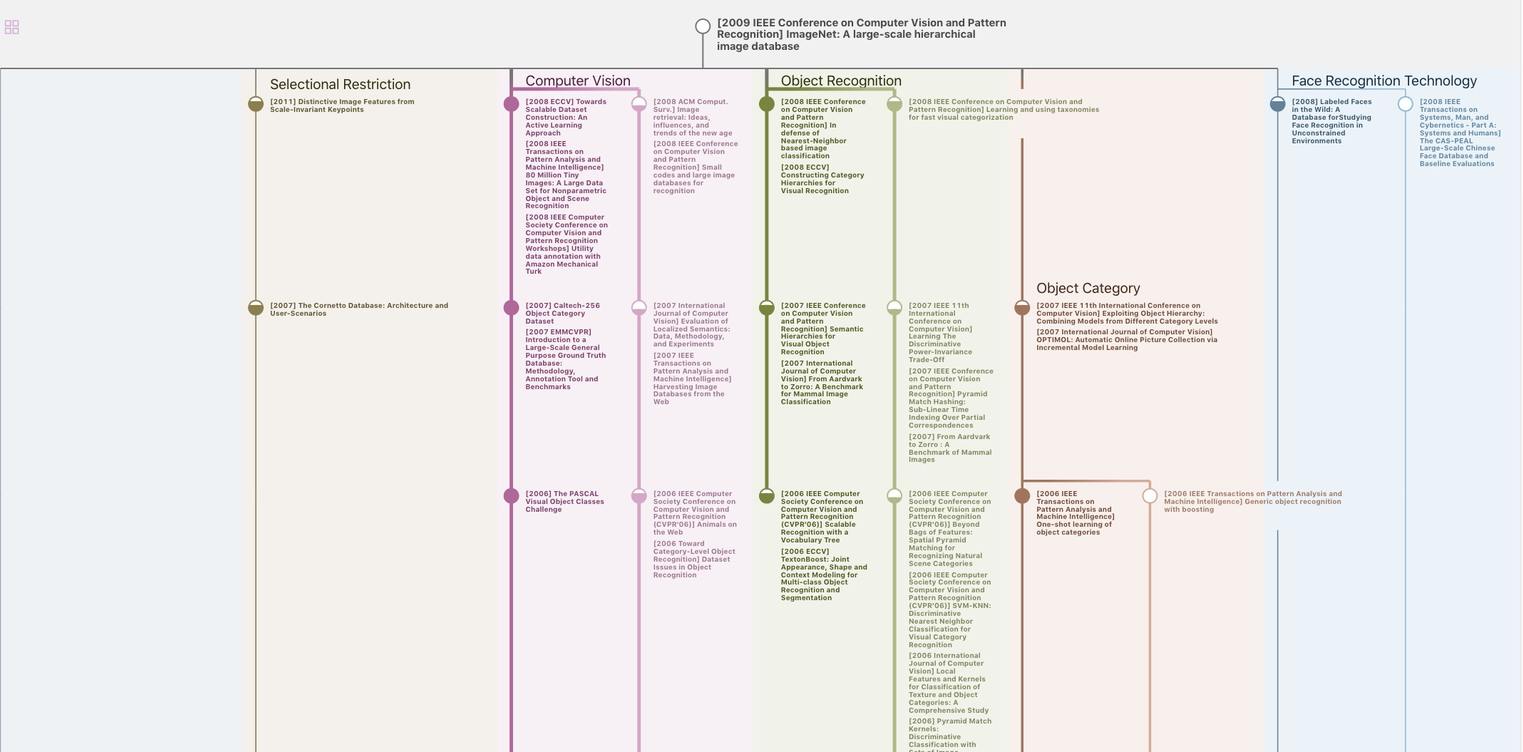
生成溯源树,研究论文发展脉络
Chat Paper
正在生成论文摘要