Classification of IoT Binaries in Resource Constrained Environments
2020 International Conference on Convergence to Digital World - Quo Vadis (ICCDW)(2020)
摘要
An overwhelming majority of the devices in the IoT ecosystem are severely constrained in terms of computing power and security, the former being one of the causes of numerous security concerns. This paper provides an efficient light-weight Convolutional Neural Network (CNN) based architecture for classification of IoT binary executables as malware or benign taking into account the severely constrained computing capabilities of the targeted devices. The proposed architecture facilitates faster classification of IoT binaries as benign or malignant using a reasonable number of parameters. The results of the experiment show that the proposed solution achieves an accuracy of around 95% using approximately 360,000 parameters. The number of parameters used in the proposed work is much less compared to what other neural network based models would use.
更多查看译文
关键词
CNN,IoT,malware,deep learning
AI 理解论文
溯源树
样例
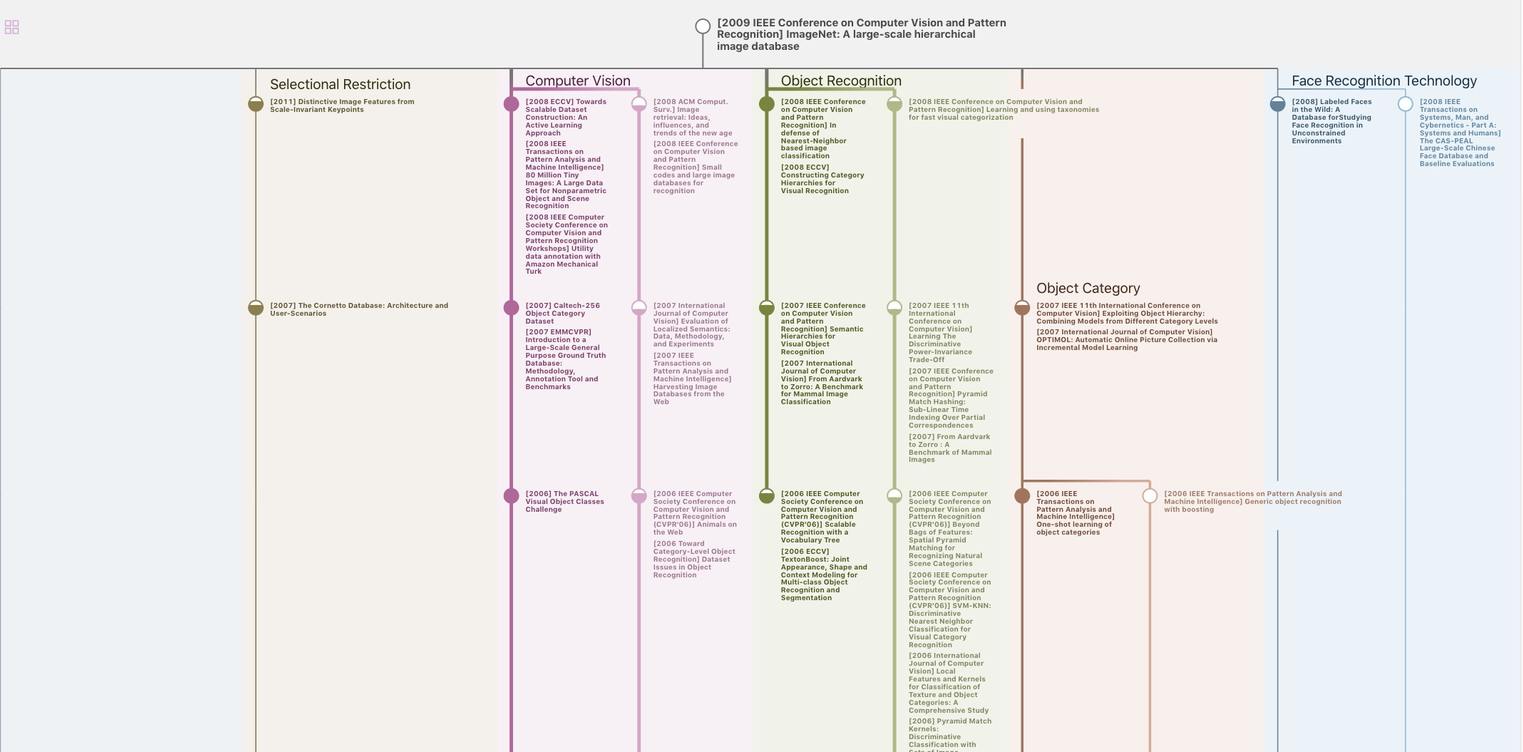
生成溯源树,研究论文发展脉络
Chat Paper
正在生成论文摘要