Neural Enhancement of Factor Graph-based Symbol Detection
2022 IEEE 23rd International Workshop on Signal Processing Advances in Wireless Communication (SPAWC)(2022)
摘要
We study the application of the factor graph framework for symbol detection on linear inter-symbol interference channels. Cyclic factor graphs have the potential to yield low-complexity symbol detectors, but are suboptimal if the ubiquitous sum-product algorithm is applied. In this paper, we present and evaluate strategies to improve the performance of cyclic factor graph-based symbol detection algorithms by means of neural enhancement. In particular, we apply neural belief propagation as an effective way to counteract the effect of cycles within the factor graph. We further propose the application and optimization of a linear preprocessor of the channel output. By modifying the observation model, the preprocessing can effectively change the underlying factor graph, thereby significantly improving the detection performance as well as reducing the complexity.
更多查看译文
关键词
Factor graphs,neural belief propagation,symbol detection,channels with memory
AI 理解论文
溯源树
样例
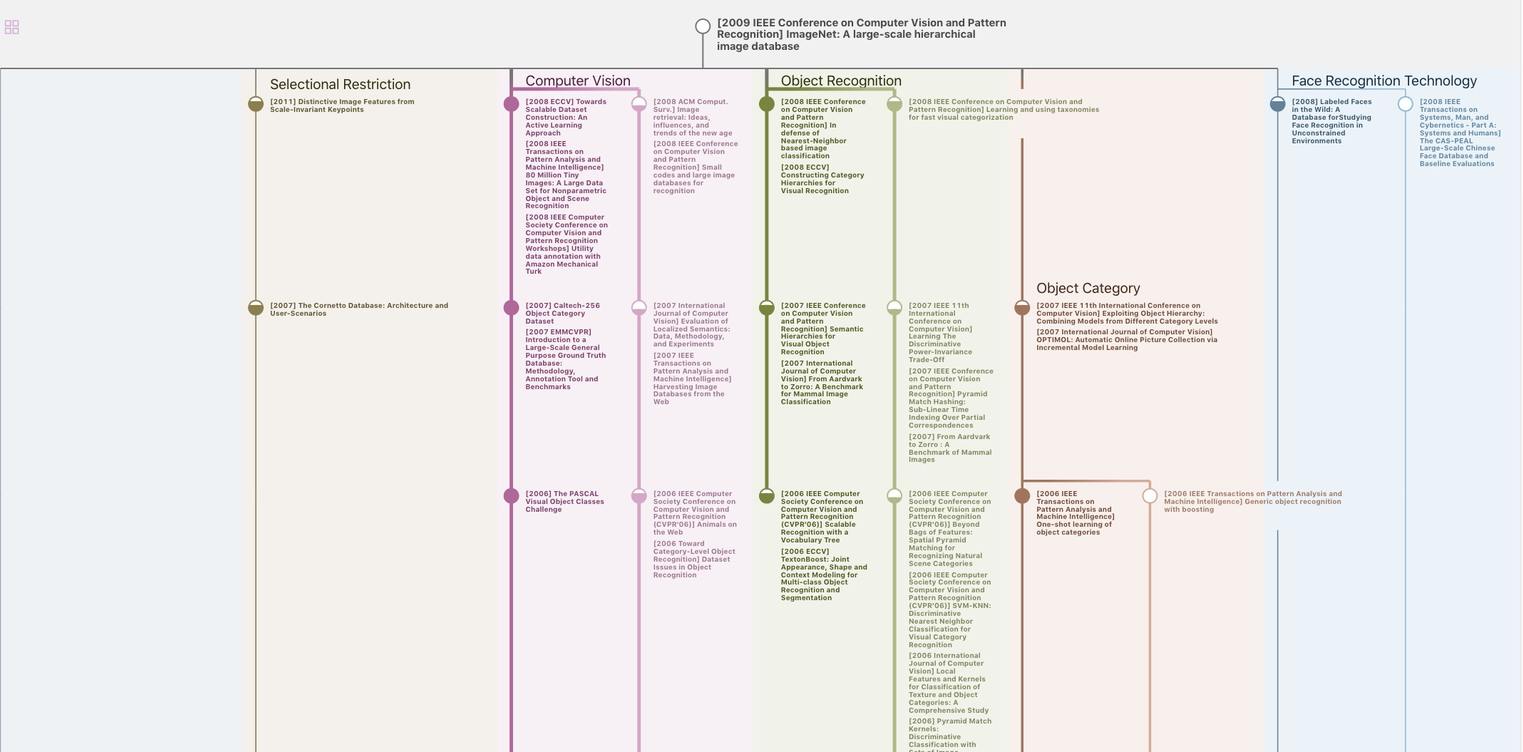
生成溯源树,研究论文发展脉络
Chat Paper
正在生成论文摘要