Maximizing conditional independence for unsupervised domain adaptation
Science China Information Sciences(2024)
摘要
Unsupervised domain adaptation (UDA) studies how to transfer a learner from a labeled source domain to an unlabeled target domain with different distributions. Existing methods mainly focus on matching marginal distributions of the source and target domains, which probably leads to a misalignment of samples from the same class but different domains. In this paper, we tackle this misalignment issue by achieving the class-conditioned transferring from a new perspective. Specifically, we propose a method named maximizing conditional independence (MCI) for UDA, which maximizes the conditional independence of feature and domain given class in the reproducing kernel Hilbert spaces. The optimization of conditional independence can be viewed as a surrogate for minimizing class-wise mutual information between feature and domain. An interpretable empirical estimation of the conditional dependence measure is deduced and connected with the unconditional case. Besides, we provide an upper bound on the target error by taking the class-conditional distribution into account, which provides a new theoretical insight for class-conditioned transferring. Extensive experiments on six benchmark datasets and various ablation studies validate the effectiveness of the proposed model in dealing with UDA.
更多查看译文
关键词
conditional independence,kernel method,domain adaptation,class-conditioned transferring
AI 理解论文
溯源树
样例
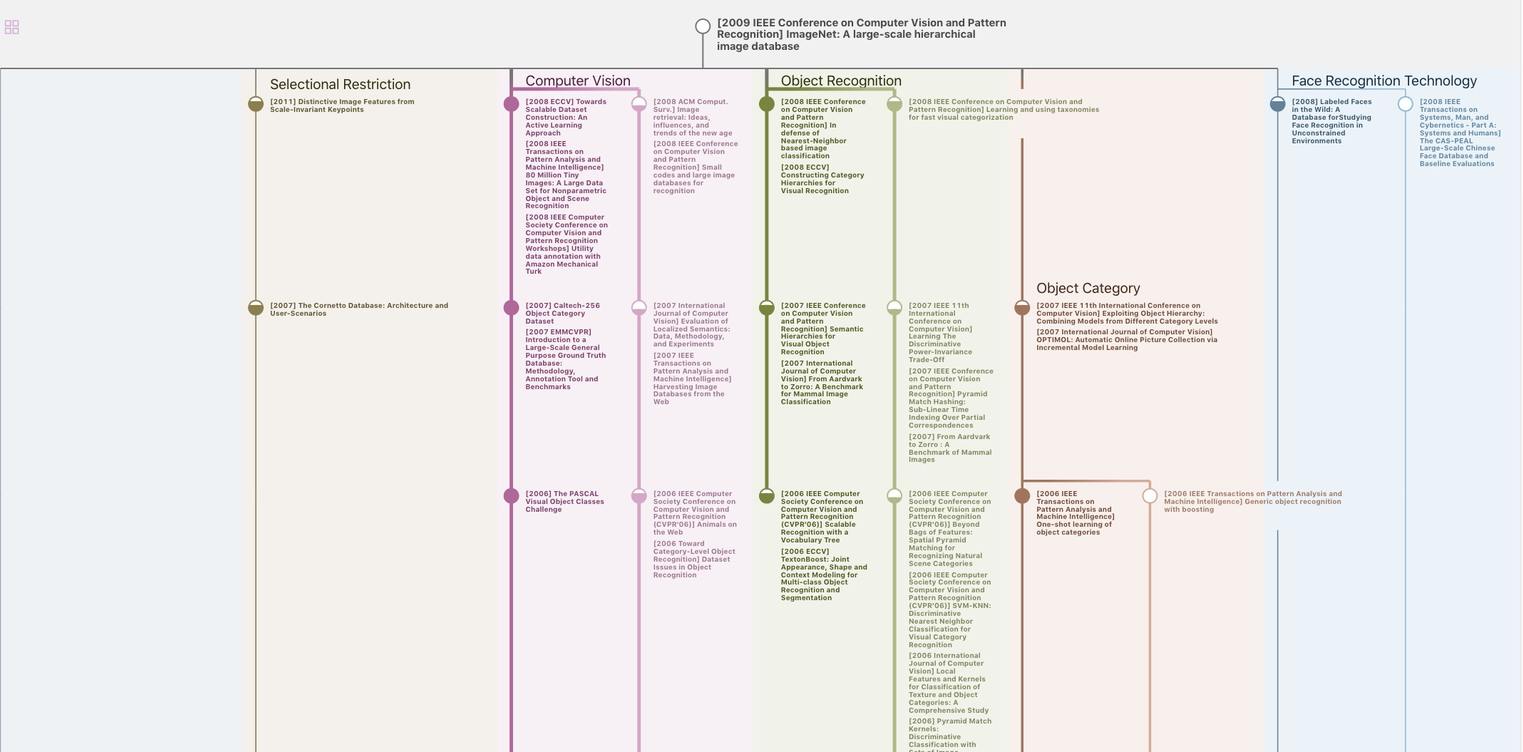
生成溯源树,研究论文发展脉络
Chat Paper
正在生成论文摘要