Dynamic Template Selection Through Change Detection for Adaptive Siamese Tracking
IEEE International Joint Conference on Neural Network (IJCNN)(2022)
摘要
Deep Siamese trackers have recently gained much attention in recent years since they can track visual objects at high speeds. Additionally, adaptive tracking methods, where target samples collected by the tracker are employed for online learning, have achieved state-of-the-art accuracy. However, single object tracking (SOT) remains a challenging task in real-world application due to changes and deformations in a target object's appearance. Learning on all the collected samples may lead to catastrophic forgetting, and thereby corrupt the tracking model. In this paper, SOT is formulated as an online incremental learning problem. A new method is proposed for dynamic sample selection and memory replay, preventing template corruption. In particular, we propose a change detection mechanism to detect gradual changes in object appearance and select the corresponding samples for online adaption. In addition, an entropy-based sample selection strategy is introduced to maintain a diversified auxiliary buffer for memory replay. Our proposed method can be integrated into any object tracking algorithm that leverages online learning for model adaptation. Extensive experiments conducted on the OTB-100, LaSOT, UAV123, and TrackingNet datasets highlight the cost-effectiveness of our method, along with the contribution of its key components. Results indicate that integrating our proposed method into state-of-art adaptive Siamese trackers can increase the potential benefits of a template update strategy, and significantly improve performance.
更多查看译文
关键词
Visual Object Tracking,Deep Learning,Change Detection,Adaptive Siamese Tracking,Video Surveillance
AI 理解论文
溯源树
样例
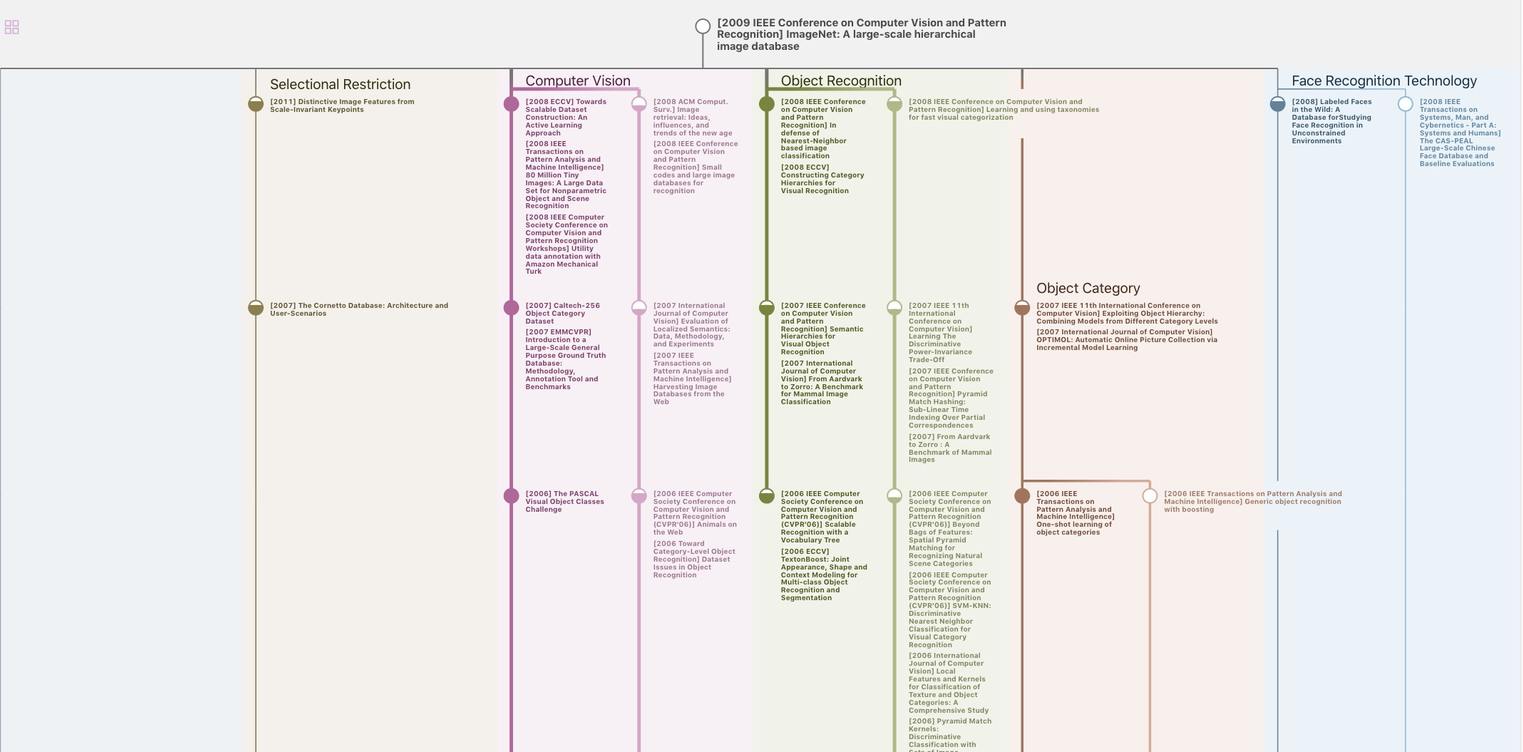
生成溯源树,研究论文发展脉络
Chat Paper
正在生成论文摘要