Robust Estimation of Covariance Matrices: Adversarial Contamination and Beyond
arxiv(2022)
摘要
We consider the problem of estimating the covariance structure of a random vector $Y\in \mathbb R^d$ from a sample $Y_1,\ldots,Y_n$. We are interested in the situation when $d$ is large compared to $n$ but the covariance matrix $\Sigma$ of interest has (exactly or approximately) low rank. We assume that the given sample is (a) $\epsilon$-adversarially corrupted, meaning that $\epsilon$ fraction of the observations could have been replaced by arbitrary vectors, or that (b) the sample is i.i.d. but the underlying distribution is heavy-tailed, meaning that the norm of $Y$ possesses only finite fourth moments. We propose an estimator that is adaptive to the potential low-rank structure of the covariance matrix as well as to the proportion of contaminated data, and admits tight deviation guarantees despite rather weak assumptions on the underlying distribution. Finally, we discuss the algorithms that allow to approximate the proposed estimator in a numerically efficient way.
更多查看译文
关键词
adversarial contamination,robust estimation,covariance matrices
AI 理解论文
溯源树
样例
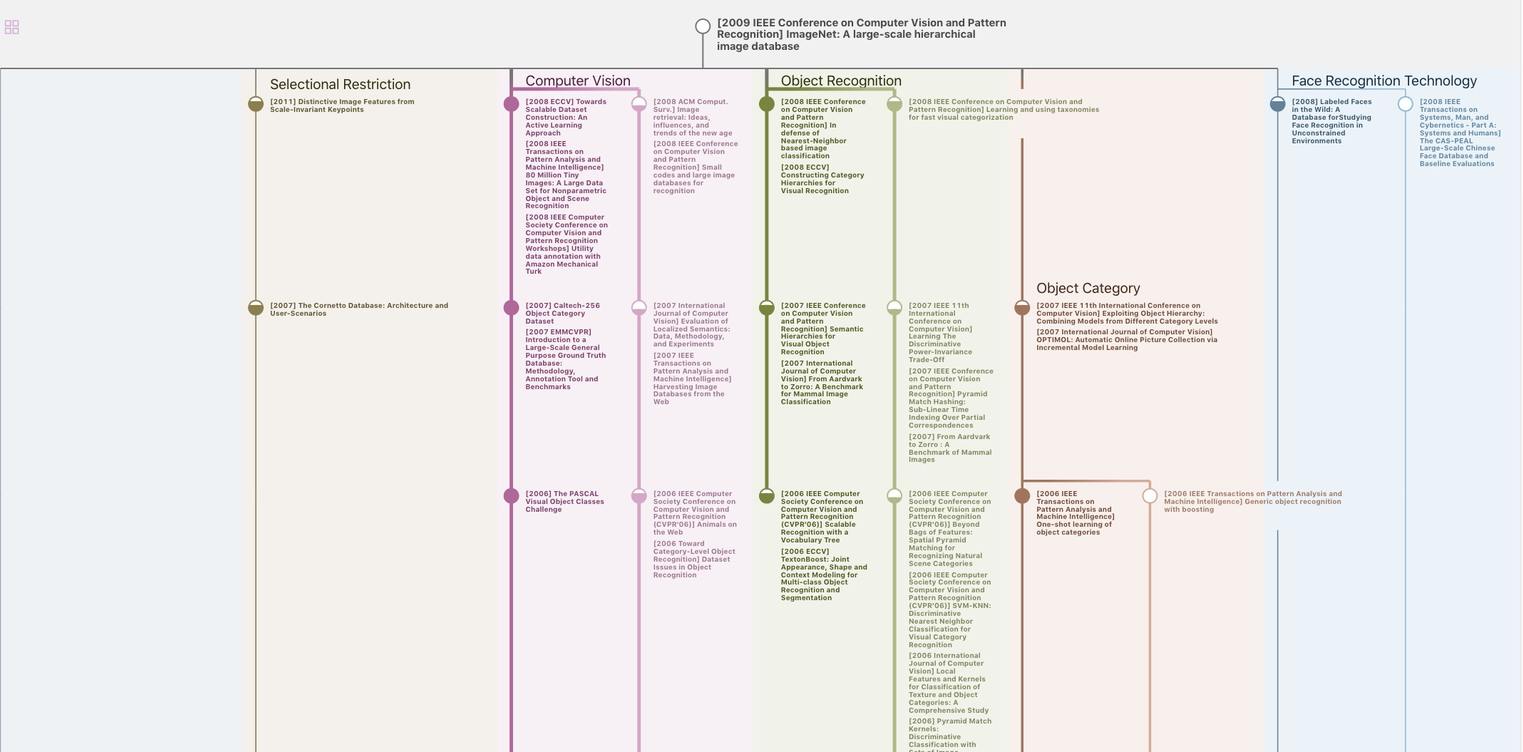
生成溯源树,研究论文发展脉络
Chat Paper
正在生成论文摘要