Adversarial Dual-Student With Differentiable Spatial Warping for Semi-Supervised Semantic Segmentation
IEEE Transactions on Circuits and Systems for Video Technology(2023)
摘要
A common challenge posed to robust semantic segmentation is the expensive data annotation cost. Existing semi-supervised solutions show great potential for solving this problem. Their key idea is constructing consistency regularization with unsupervised data augmentation from unlabeled data for model training. The perturbations for unlabeled data enable the consistency training loss, which benefits semi-supervised semantic segmentation. However, these perturbations destroy image context and introduce unnatural boundaries, which is harmful for semantic segmentation. Besides, the widely adopted semi-supervised learning framework, i.e. mean-teacher, suffers performance limitation since the student model finally converges to the teacher model. In this paper, first of all, we propose a context friendly differentiable geometric warping to conduct unsupervised data augmentation; secondly, a novel adversarial dual-student framework is proposed to improve the Mean-Teacher from the following two aspects: (1) dual student models are learned independently except for a stabilization constraint to encourage exploiting model diversities; (2) adversarial training scheme is applied to both students and the discriminators are resorted to distinguish reliable pseudo-label of unlabeled data for self-training. Effectiveness is validated via extensive experiments on PASCAL VOC2012 and Cityscapes. Our solution significantly improves the performance and state-of-the-art results are achieved on both datasets. Remarkably, compared with fully supervision, our solution achieves comparable mIoU of 73.4% using only 12.5% annotated data on PASCAL VOC2012. Our codes and models are available at
https://github.com/cao-cong/ADS-SemiSeg
.
更多查看译文
关键词
Semi-supervised semantic segmentation,dual-student,differentiable spatial warping
AI 理解论文
溯源树
样例
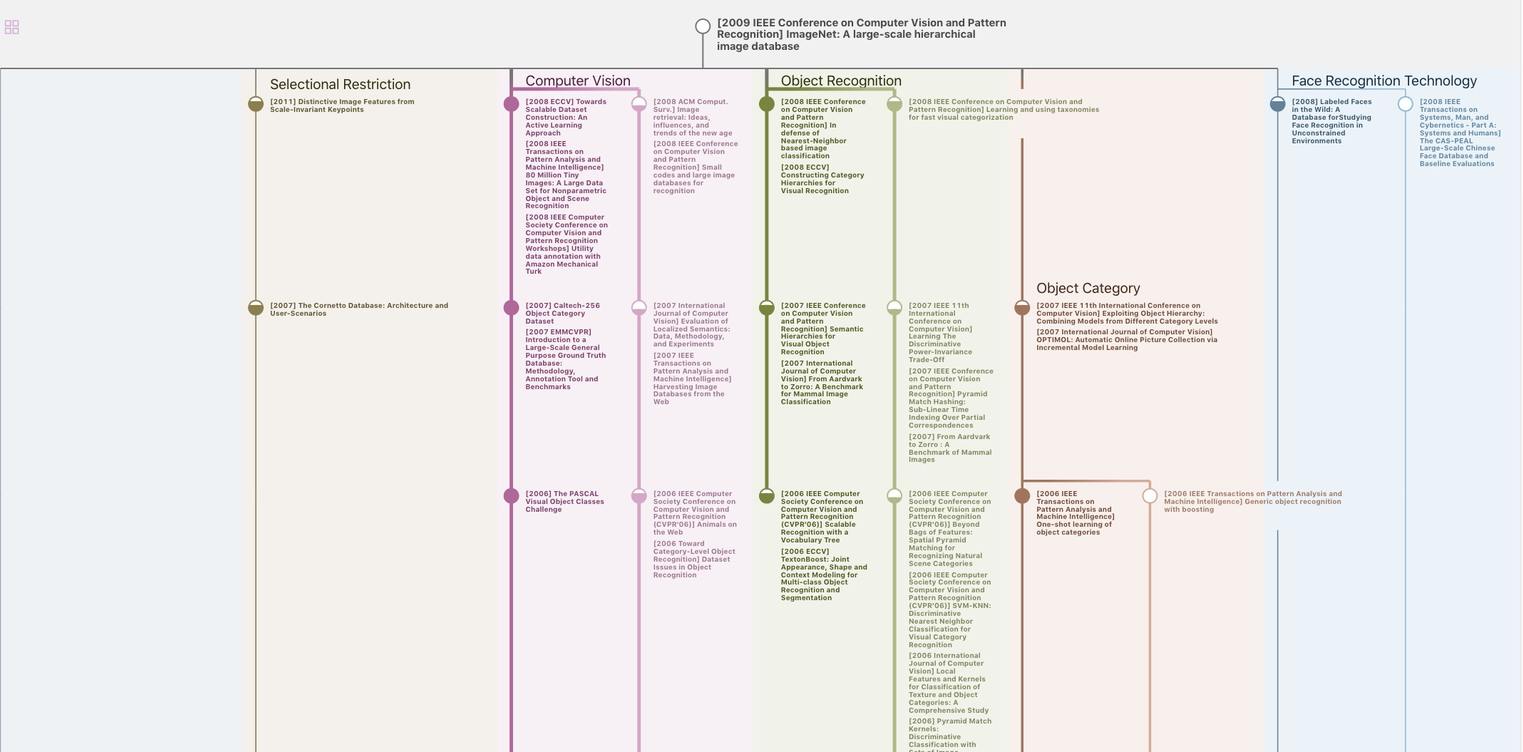
生成溯源树,研究论文发展脉络
Chat Paper
正在生成论文摘要