Towards Robust Part-aware Instance Segmentation for Industrial Bin Picking
2022 International Conference on Robotics and Automation (ICRA)(2022)
摘要
Industrial bin picking is a challenging task that requires accurate and robust segmentation of individual object instances. Particularly, industrial objects can have irregular shapes, that is, thin and concave, whereas in bin-picking scenarios, objects are often closely packed with strong occlusion. To address these challenges, we formulate a novel part-aware instance segmentation pipeline. The key idea is to decompose industrial objects into correlated approximate convex parts and enhance the object-level segmentation with part-level segmentation. We design a part-aware network to predict part masks and part-to-part offsets, followed by a part aggregation module to assemble the recognized parts into instances. To guide the network learning, we also propose an automatic label decoupling scheme to generate ground-truth part-level labels from instance-level labels. Finally, we contribute the first instance segmentation dataset, which contains a variety of industrial objects that are thin and have non-trivial shapes. Extensive experimental results on various industrial objects demonstrate that our method can achieve the best segmentation results compared with the state-of-the-art approaches.
更多查看译文
关键词
industrial bin
AI 理解论文
溯源树
样例
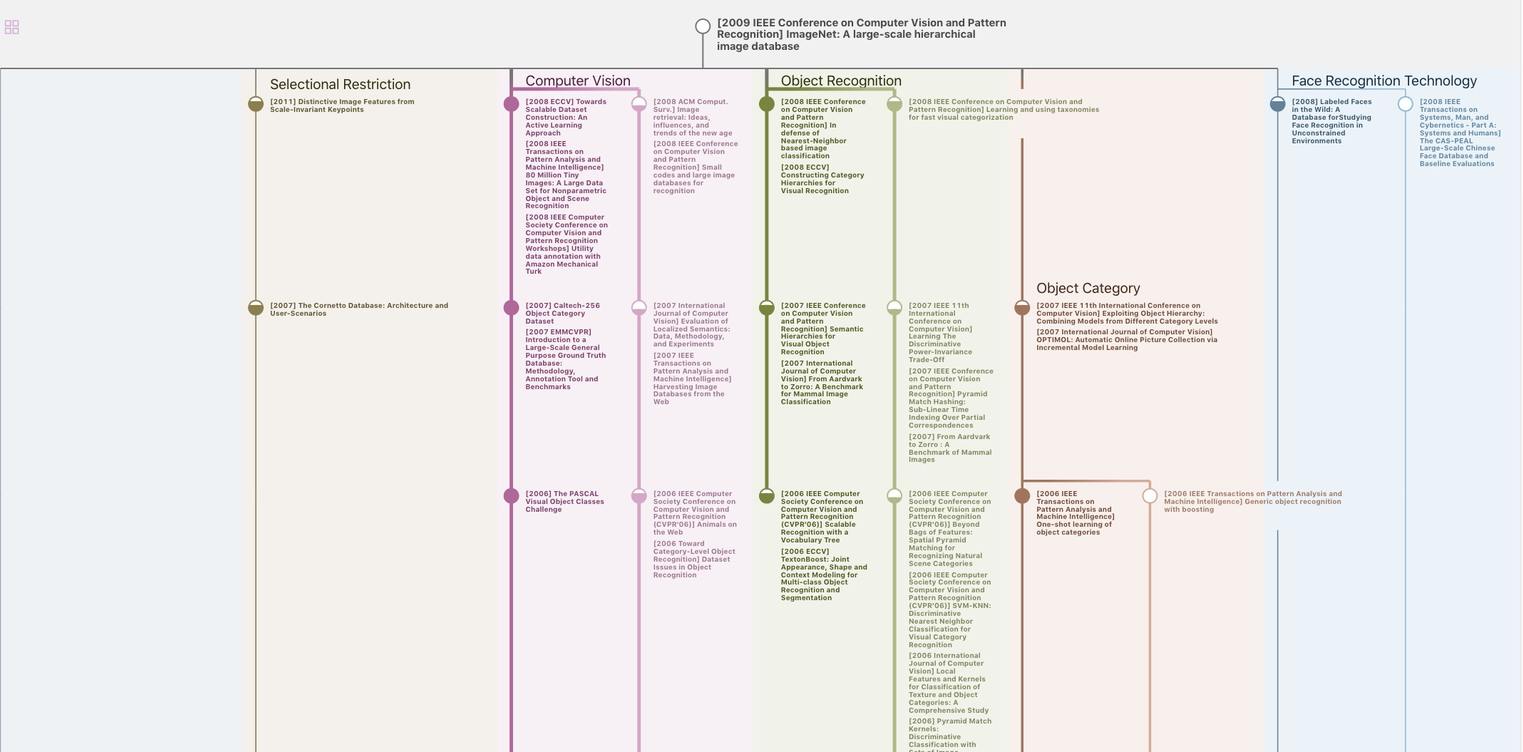
生成溯源树,研究论文发展脉络
Chat Paper
正在生成论文摘要