Target Network and Truncation Overcome the Deadly Triad in Q-Learning
SIAM JOURNAL ON MATHEMATICS OF DATA SCIENCE(2023)
摘要
Q-learning with function approximation is one of the most empirically successful while theoretically mysterious reinforcement learning (RL) algorithms and was identified in [R. S. Sutton, in European Conference on Computational Learning Theory, Springer, New York, 1999, pp. 11-17] as one of the most important theoretical open problems in the RL community. Even in the basic setting where linear function approximation is used, there are well-known divergent examples. In this work, we propose a stable online variant of Q-learning with linear function approximation that uses target network and truncation and is driven by a single trajectory of Markovian samples. We present the finite-sample guarantees of the algorithm, which imply a sample complexity of (O) over tilde (epsilon(-2)) up to a function approximation error. Importantly, we establish the results under minimal assumptions and do not modify the problem parameters to achieve stability.
更多查看译文
关键词
reinforcement learning,Q-learning,linear function approximation,finite-sample analysis
AI 理解论文
溯源树
样例
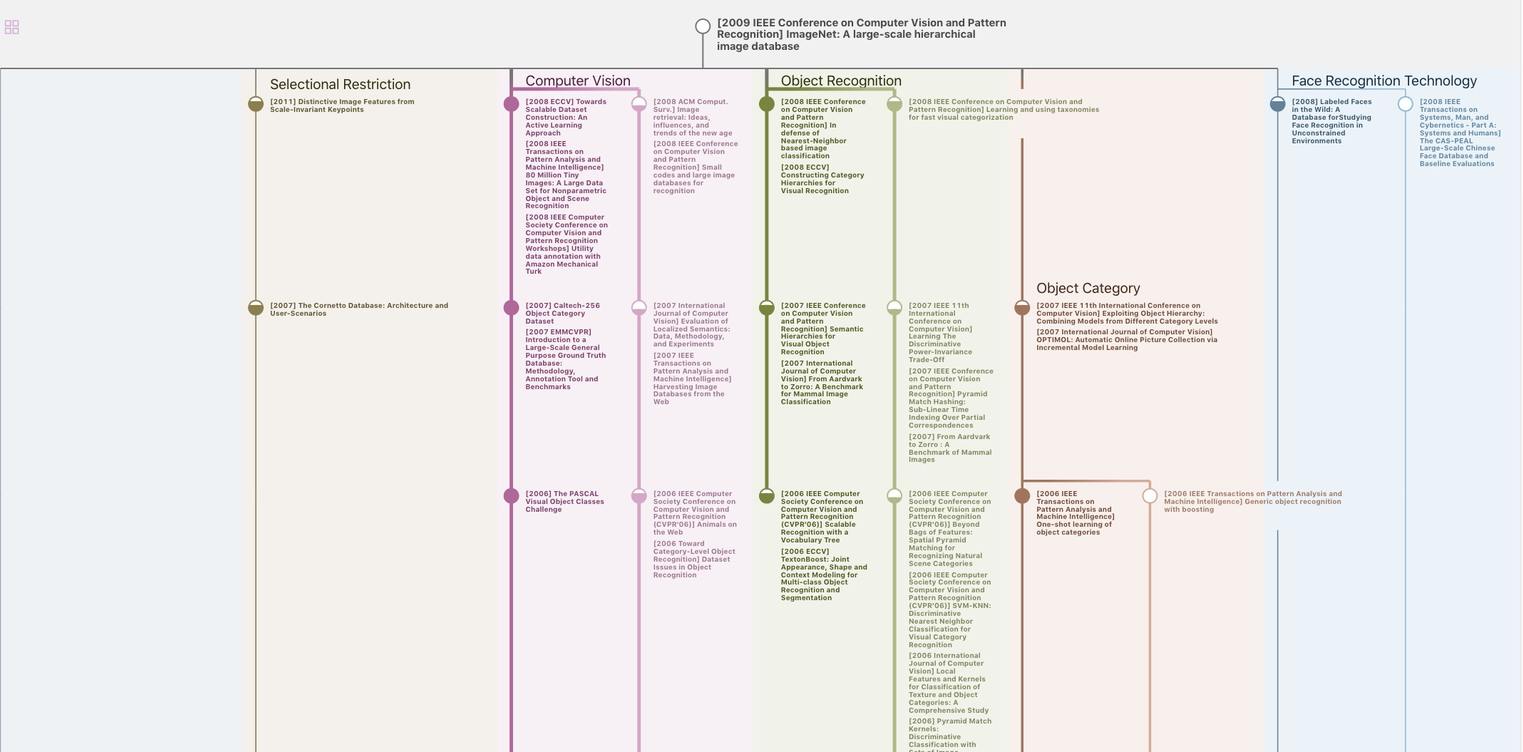
生成溯源树,研究论文发展脉络
Chat Paper
正在生成论文摘要