Concept-based Explanations for Out-Of-Distribution Detectors
ICLR 2023(2023)
摘要
Out-of-distribution (OOD) detection plays a crucial role in ensuring the safe deployment of deep neural network (DNN) classifiers. While a myriad of methods have focused on improving the performance of OOD detectors, a critical gap remains in interpreting their decisions. We help bridge this gap by providing explanations for OOD detectors based on learned high-level concepts. We first propose two new metrics for assessing the effectiveness of a particular set of concepts for explaining OOD detectors: 1) detection completeness, which quantifies the sufficiency of concepts for explaining an OOD-detector's decisions, and 2) concept separability, which captures the distributional separation between in-distribution and OOD data in the concept space. Based on these metrics, we propose a framework for learning a set of concepts that satisfy the desired properties of detection completeness and concept separability and demonstrate the framework's effectiveness in providing concept-based explanations for diverse OOD techniques. We also show how to identify prominent concepts that contribute to the detection results via a modified Shapley value-based importance score.
更多查看译文
关键词
out-of-distribution detection,interpretability,concept-based explanations
AI 理解论文
溯源树
样例
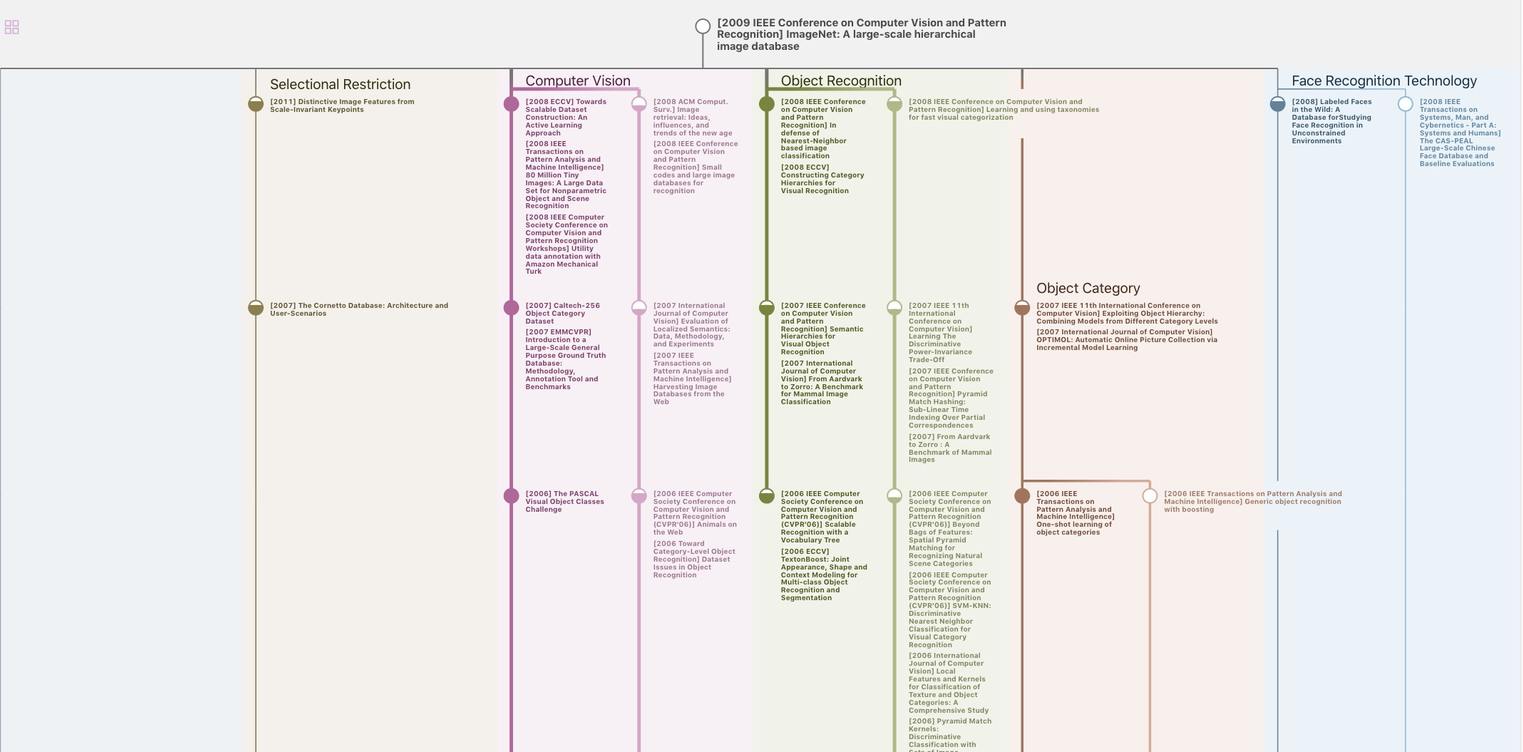
生成溯源树,研究论文发展脉络
Chat Paper
正在生成论文摘要