FLOPS: efficient on-chip learning for optical neural networks through stochastic zeroth-order optimization
DAC(2020)
摘要
ABSTRACTOptical neural networks (ONNs) have attracted extensive attention due to its ultra-high execution speed and low energy consumption. The traditional software-based ONN training, however, suffers the problems of expensive hardware mapping and inaccurate variation modeling while the current on-chip training methods fail to leverage the self-learning capability of ONNs due to algorithmic inefficiency and poor variation-robustness. In this work, we propose an on-chip learning method to resolve the aforementioned problems that impede ONNs' full potential for ultra-fast forward acceleration. We directly optimize optical components using stochastic zeroth-order optimization on-chip, avoiding the traditional high-overhead back-propagation, matrix decomposition, or in situ device-level intensity measurements. Experimental results demonstrate that the proposed on-chip learning framework provides an efficient solution to train integrated ONNs with 3~4x fewer ONN forward, higher inference accuracy, and better variation-robustness than previous works.
更多查看译文
AI 理解论文
溯源树
样例
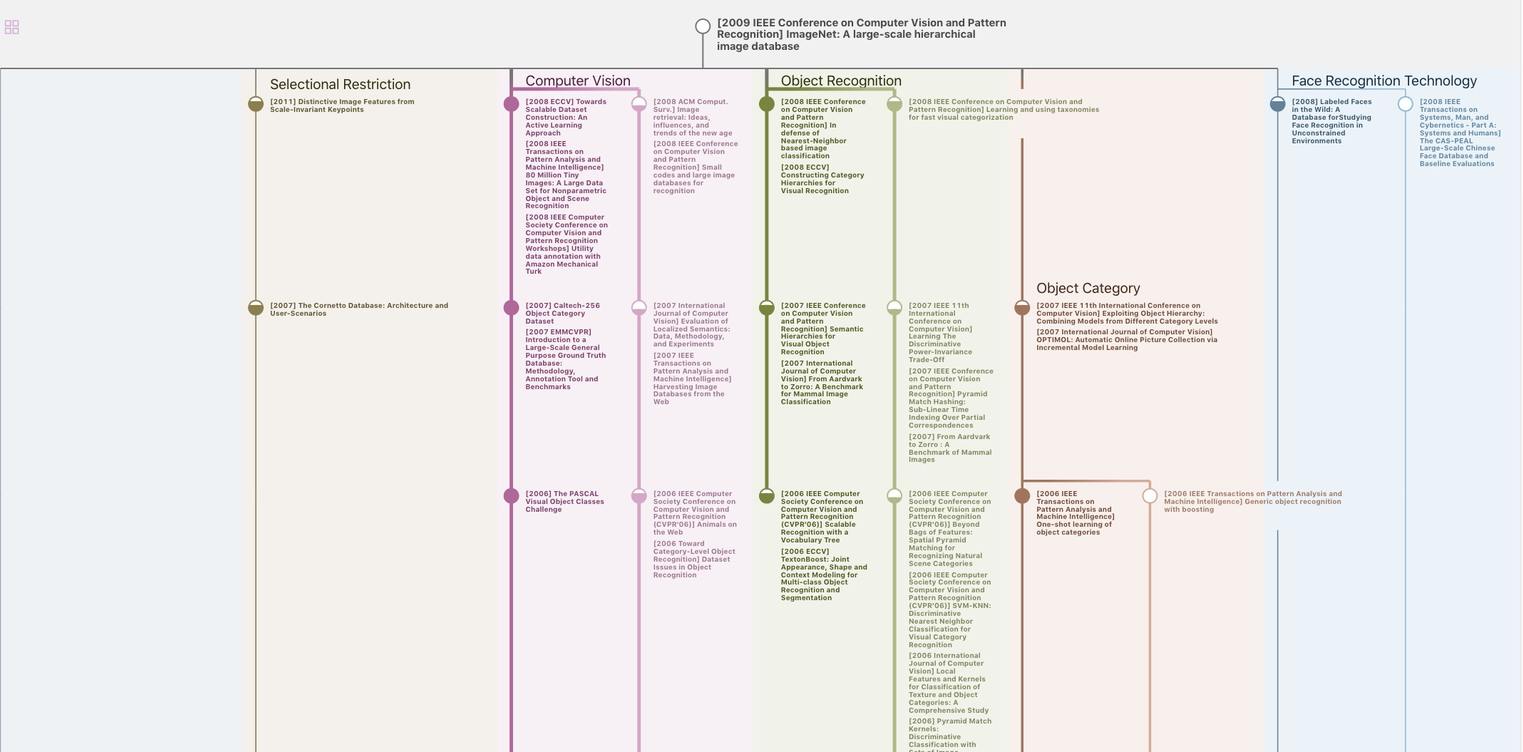
生成溯源树,研究论文发展脉络
Chat Paper
正在生成论文摘要