Simultaneous Past and Current Social Interaction-aware Trajectory Prediction for Multiple Intelligent Agents in Dynamic Scenes
ACM Transactions on Intelligent Systems and Technology(2022)
摘要
AbstractTrajectory prediction of multiple agents in a crowded scene is an essential component in many applications, including intelligent monitoring, autonomous robotics, and self-driving cars. Accurate agent trajectory prediction remains a significant challenge because of the complex dynamic interactions among the agents and between them and the surrounding scene. To address the challenge, we propose a decoupled attention-based spatial-temporal modeling strategy in the proposed trajectory prediction method. The past and current interactions among agents are dynamically and adaptively summarized by two separate attention-based networks and have proven powerful in improving the prediction accuracy. Moreover, it is optional in the proposed method to make use of the road map and the plan of the ego-agent for scene-compliant and accurate predictions. The road map feature is efficiently extracted by a convolutional neural network, and the features of the ego-agent’s plan is extracted by a gated recurrent network with an attention module based on the temporal characteristic. Experiments on benchmark trajectory prediction datasets demonstrate that the proposed method is effective when the ego-agent plan and the the surrounding scene information are provided and achieves state-of-the-art performance with only the observed trajectories.
更多查看译文
关键词
Trajectory data analysis, neural networks, autonomous robots
AI 理解论文
溯源树
样例
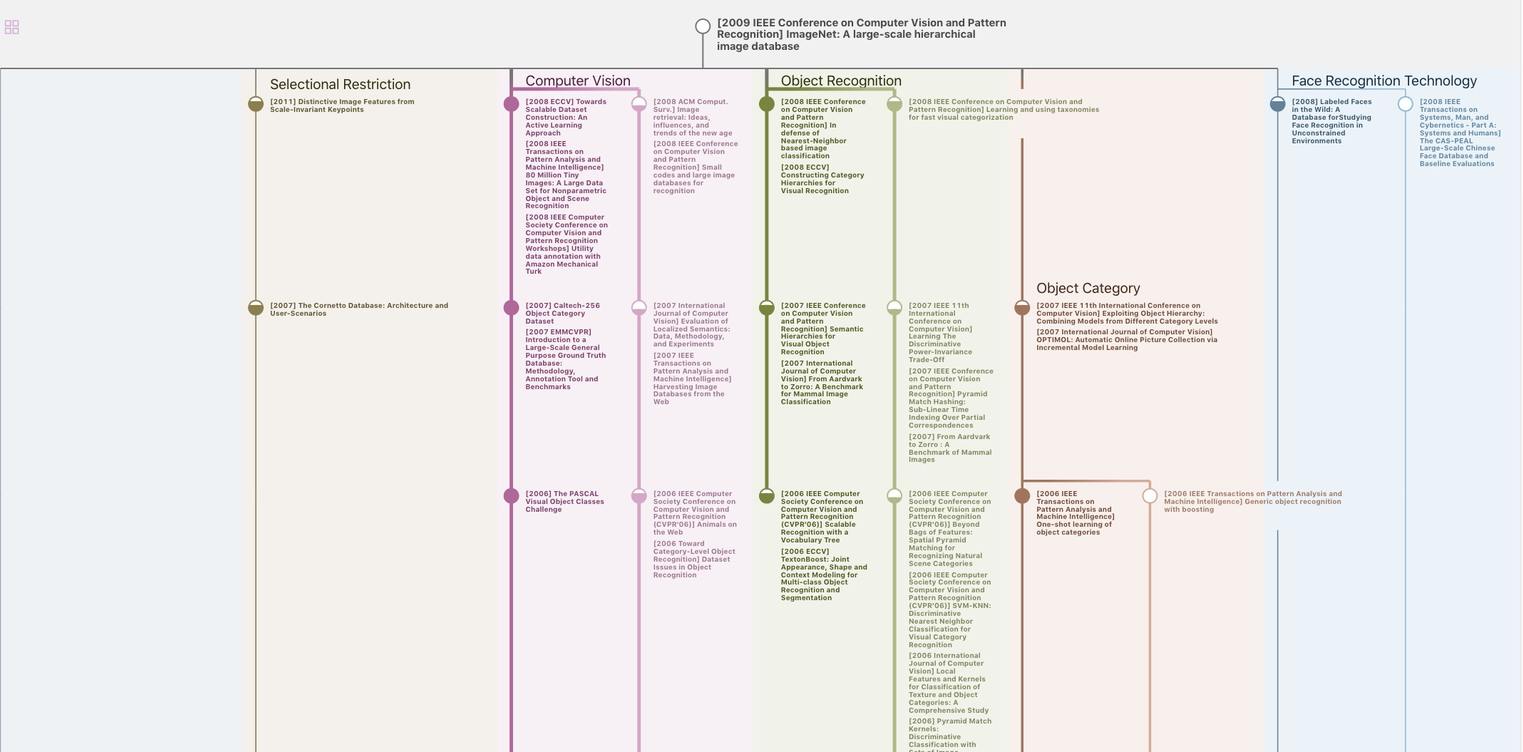
生成溯源树,研究论文发展脉络
Chat Paper
正在生成论文摘要