JobPruner
Periodicals(2018)
摘要
AbstractHigh Performance Computing (HPC) applications are essential for scientists and engineers to create and understand models and their properties. These professionals depend on the execution of large sets of computational jobs that explore combinations of parameter values. Avoiding the execution of unnecessary jobs brings not only speed to these experiments, but also reductions in infrastructure usageparticularly important due to the shift of these applications to HPC cloud platforms. Our hypothesis is that data generated by these experiments can help users in identifying such jobs. To address this hypothesis we need to understand the similarity levels among multiple experiments necessary for job elimination decisions and the steps required to automate this process. In this paper we present a study and a machine learning-based tool called JobPruner to support parameter exploration in HPC experiments. The tool was evaluated with three real-world use cases from different domains including seismic analysis and agronomy. We observed the tool reduced 93% of jobs in a single experiment, while improving quality in most scenarios. In addition, reduction in job executions was possible even considering past experiments with low correlations. A tool to reduce search spaces in HPC experiments.Search space reduction based on machine learning.Evaluation using real industry applications.
更多查看译文
关键词
HPC,Machine learning,Parametric applications,Search space exploration
AI 理解论文
溯源树
样例
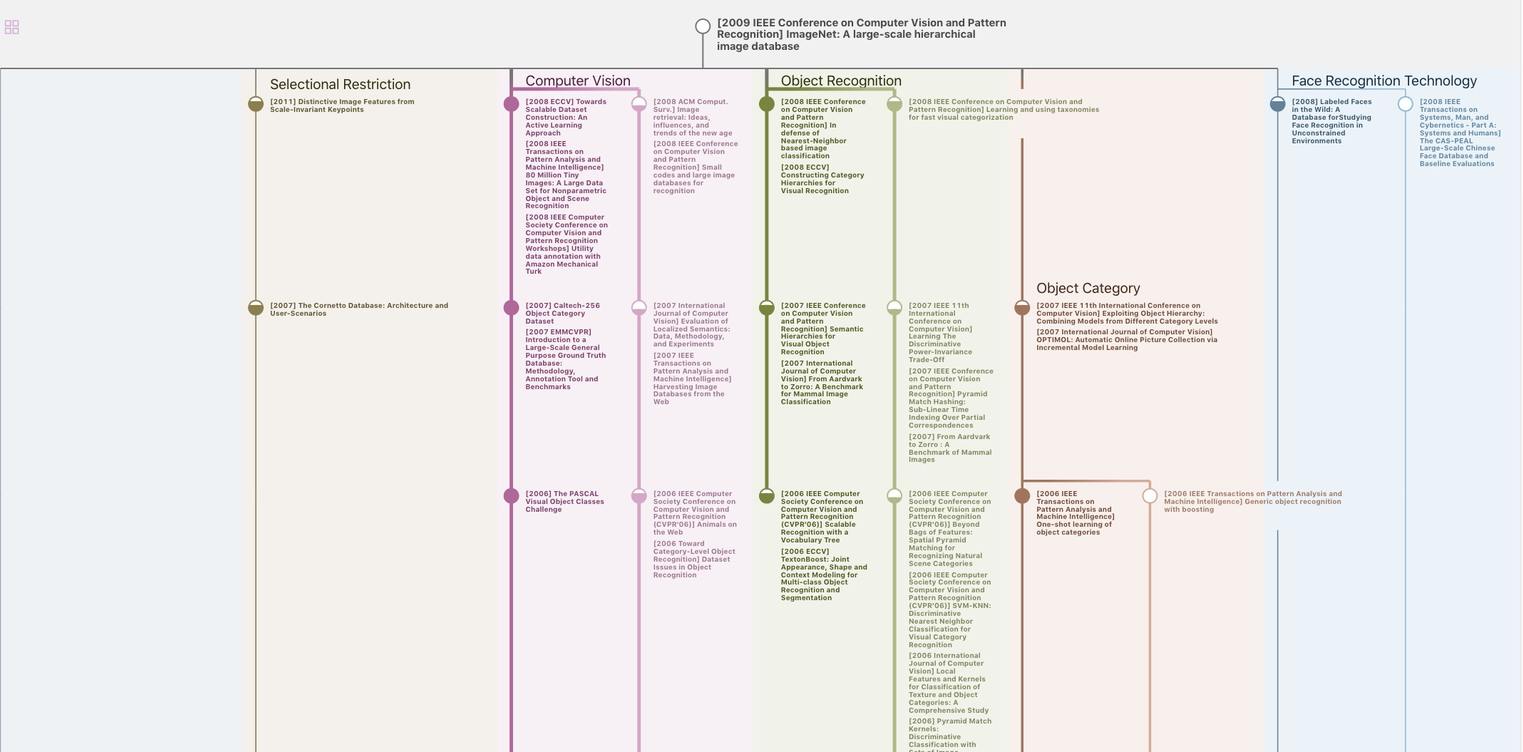
生成溯源树,研究论文发展脉络
Chat Paper
正在生成论文摘要