SalesExplorer
Periodicals(2016)
摘要
AbstractIn commercial sales and services, recommender systems have been widely adopted to predict customers' purchase interests using their prior purchasing behaviors. Cold-start is a known challenge to existing recommendation techniques, e.g., the popular collaborative filtering method is not applicable to predict the interests of "white-space" customers since they have no prior purchasing history in the targeted product categories.This paper presents SalesExplorer, a new recommendation algorithm to address "white-space" customer issue in the commercial sales and services segment. To predict the interests of customers who are new to a product category, we propose a statistical inference method using customers' existing purchase records from other product categories, a Probabilistic Latent Semantic Analysis (PLSA)-based transfer learning method using customers' business profile content, and a kernel logistic regression-based model which combines these two recommendations to produce the final results with higher accuracy. Experimental study using real-world enterprise sales data demonstrates that, comparing with a baseline and two state-of-the-art methods, the proposed combinatorial algorithm improves recommendation accuracy by 32.14%, 13.13% and 9.85%, respectively.
更多查看译文
关键词
Collaborative filtering,Logistic regression,Recommender system,White-space customer
AI 理解论文
溯源树
样例
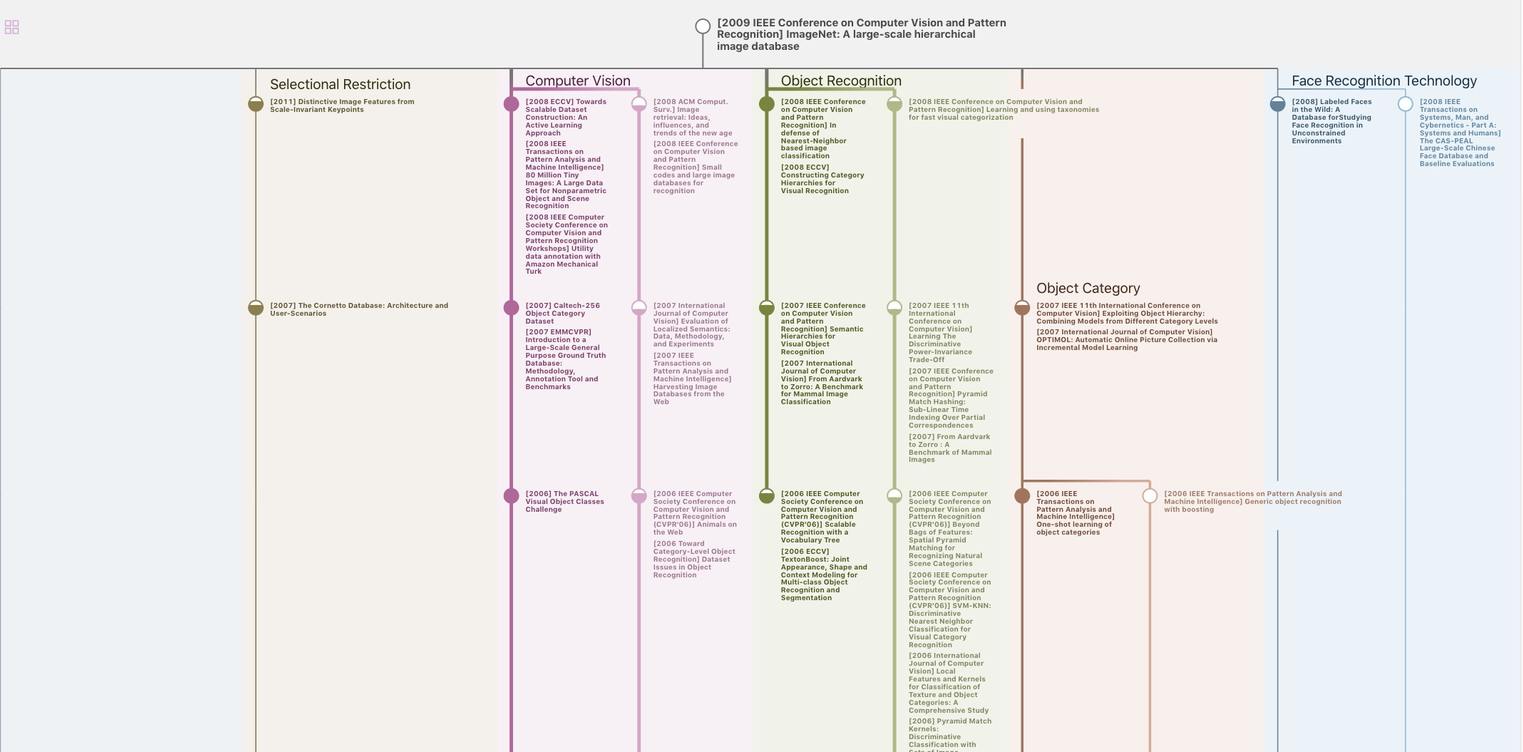
生成溯源树,研究论文发展脉络
Chat Paper
正在生成论文摘要