Transformations in Learned Image Compression from a Modulation Perspective
ArXiv(2022)
摘要
In this paper, a unified transformation method in learned image
compression(LIC) is proposed from the perspective of modulation. Firstly, the
quantization in LIC is considered as a generalized channel with additive
uniform noise. Moreover, the LIC is interpreted as a particular communication
system according to the consistency in structures and optimization objectives.
Thus, the technology of communication systems can be applied to guide the
design of modules in LIC. Furthermore, a unified transform method based on
signal modulation (TSM) is defined. In the view of TSM, the existing
transformation methods are mathematically reduced to a linear modulation. A
series of transformation methods, e.g. TPM and TJM, are obtained by extending
to nonlinear modulation. The experimental results on various datasets and
backbone architectures verify that the effectiveness and robustness of the
proposed method. More importantly, it further confirms the feasibility of
guiding LIC design from a communication perspective. For example, when backbone
architecture is hyperprior combining context model, our method achieves
3.52% BD-rate reduction over GDN on Kodak dataset without increasing
complexity.
更多查看译文
关键词
learned image compression,modulation
AI 理解论文
溯源树
样例
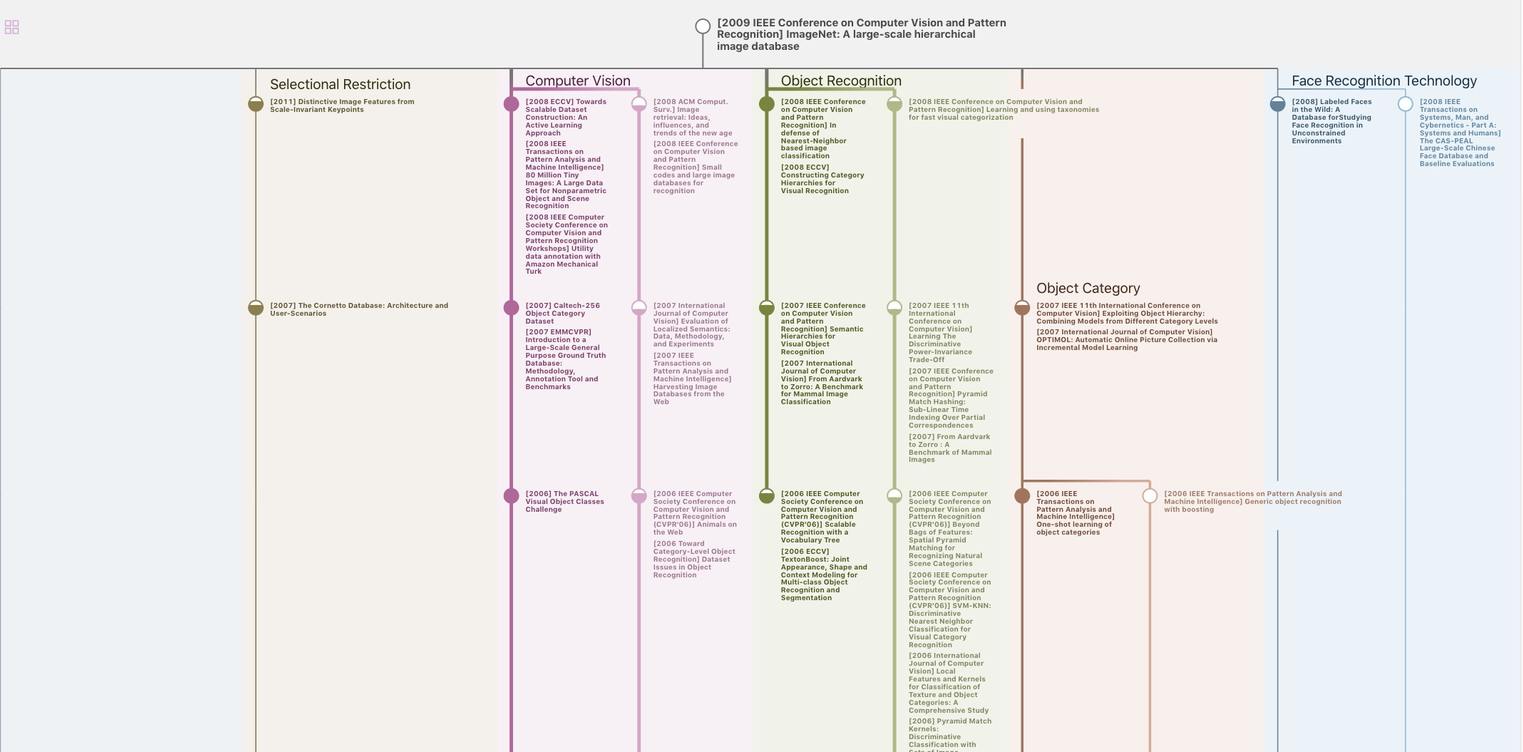
生成溯源树,研究论文发展脉络
Chat Paper
正在生成论文摘要