Structure-Aware Positional Transformer for Visible-Infrared Person Re-Identification
IEEE TRANSACTIONS ON IMAGE PROCESSING(2022)
摘要
Visible-infrared person re-identification (VI-ReID) is a cross-modality retrieval problem, which aims at matching the same pedestrian between the visible and infrared cameras. Due to the existence of pose variation, occlusion, and huge visual differences between the two modalities, previous studies mainly focus on learning image-level shared features. Since they usually learn a global representation or extract uniformly divided part features, these methods are sensitive to misalignments. In this paper, we propose a structure-aware positional transformer (SPOT) network to learn semantic-aware sharable modality features by utilizing the structural and positional information. It consists of two main components: attended structure representation (ASR) and transformer-based part interaction (TPI). Specifically, ASR models the modality-invariant structure feature for each modality and dynamically selects the discriminative appearance regions under the guidance of the structure information. TPI mines the part-level appearance and position relations with a transformer to learn discriminative part-level modality features. With a weighted combination of ASR and TPI, the proposed SPOT explores the rich contextual and structural information, effectively reducing cross-modality difference and enhancing the robustness against misalignments. Extensive experiments indicate that SPOT is superior to the state-of-the-art methods on two cross-modal datasets. Notably, the Rank-1/mAP value on the SYSU-MM01 dataset has improved by 8.43%/6.80%.
更多查看译文
关键词
Transformers, Feature extraction, Robustness, Background noise, Task analysis, Visualization, Heating systems, Visible-infrared person re-identification, transformer, structure information, interaction learning
AI 理解论文
溯源树
样例
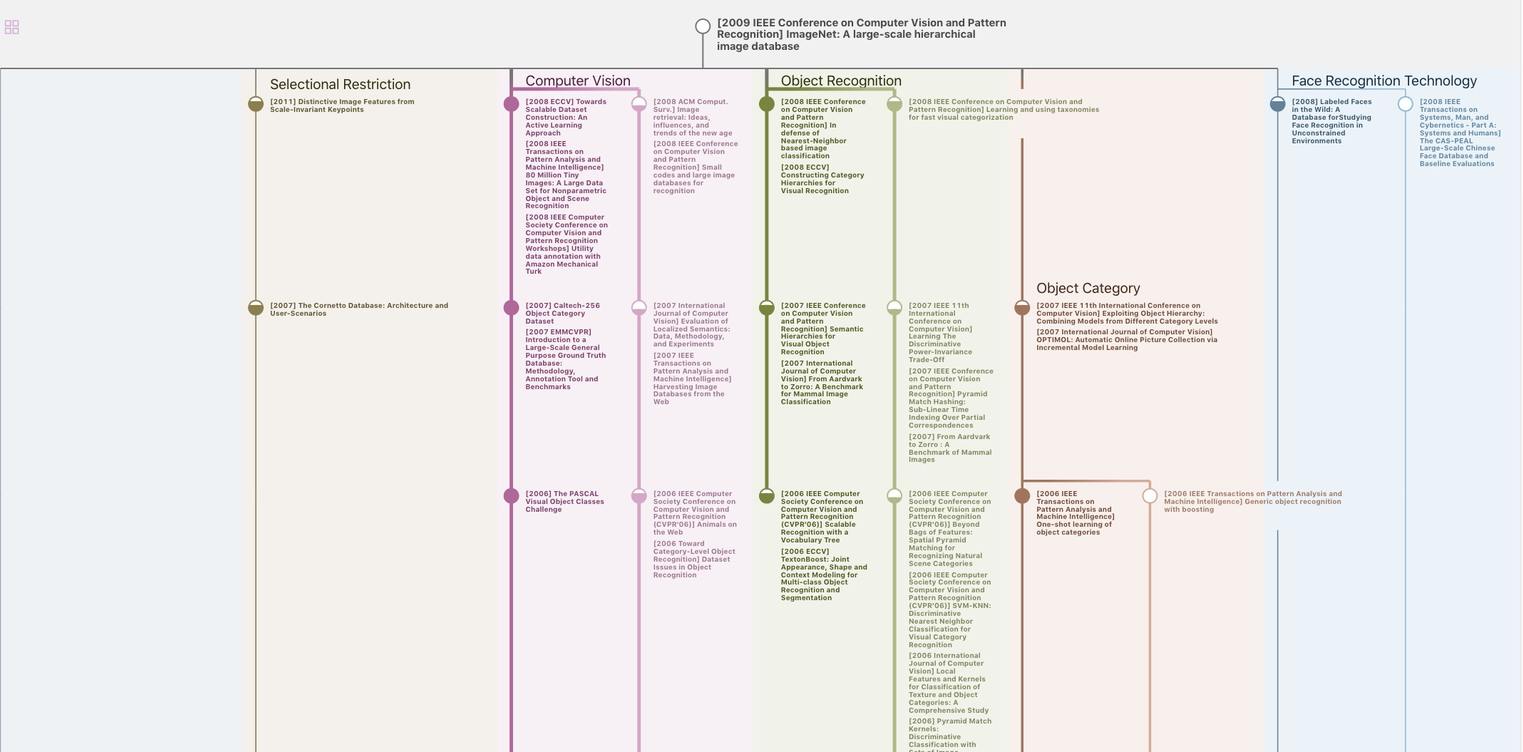
生成溯源树,研究论文发展脉络
Chat Paper
正在生成论文摘要