Unlocking the black box: Non-parametric option pricing before and during COVID-19
Annals of Operations Research(2022)
摘要
This paper addresses the interpretability problem of non-parametric option pricing models by using the explainable artificial intelligence (XAI) approach. We study call options written on the S&P 500 stock market index across three market regimes: pre-COVID-19, COVID-19 market crash, and post-COVID-19 recovery. Our comparative option pricing exercise demonstrates the superiority of the random forest and extreme gradient boosting models for each market regime. We also show that the model’s pricing accuracy has worsened from the pre-COVID-19 to the recovery period. Moneyness was the most important price determinants across the market regimes, while the implied volatility and time-to-maturity inputs contributed intermittently to a lesser extent. During the COVID-19 crash, open interest gained more economic importance due to the increased behavioral tendencies of traders consistent with market distress.
更多查看译文
关键词
Option pricing,COVID-19,Random forest,Extreme gradient boosting,Explainable artificial intelligence,Interpretability
AI 理解论文
溯源树
样例
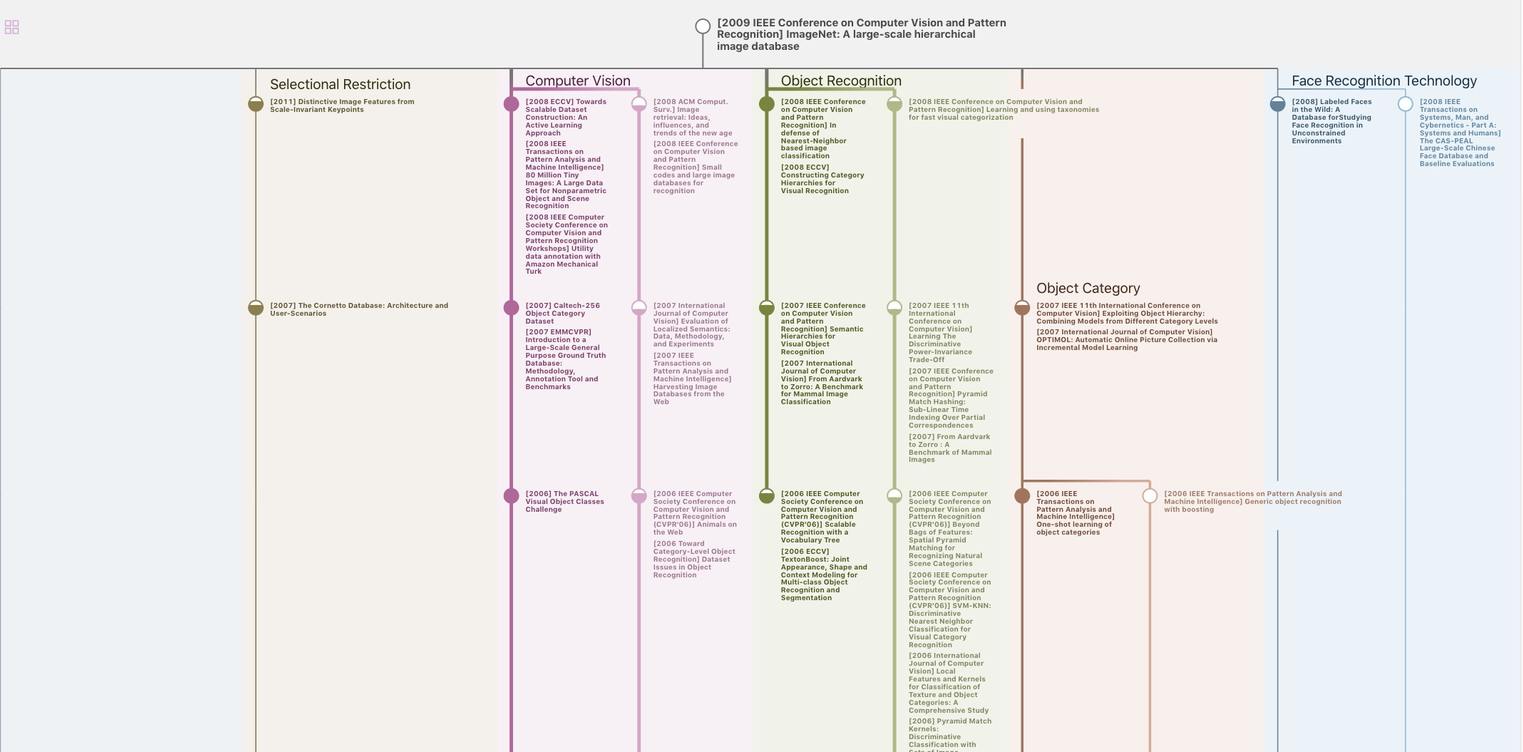
生成溯源树,研究论文发展脉络
Chat Paper
正在生成论文摘要