Typology, network features and damage response in worldwide urban road systems
PLOS ONE(2022)
摘要
We survey the network properties and response to damage sustained of road networks of cities worldwide, using OpenStreetMap (OSM) data. We find that our primary damage response variable (t(1:0)) over bar, which is the average shortest time needed to reach all nodes in a road network (which stand in for locations within a metropolitan area) from an initial node (which stands in for the location of a center for disaster relief operations), is strongly linearly-correlated with p(d), the fraction of the road network segments damaged. We find that this result, previously reported for a city's road network as opposed to grid and scale-free idealizations, is widely present across the road networks we have examined regardless of location. Furthermore, we identify three families of road networks according to their damage response, forming a typology by which we can classify city road networks. Using this typology, we identify the family of road networks which may be of most concern from a humanitarian standpoint. We also find that, of the properties of the road networks we examined, the average shortest path length, < l(min)> and the average node degree, < k >, proxies for city road network size and complexity respectively, are very significantly-correlated with damage susceptibility. In addition to forming a damage response typology by which city road networks could be classified, we consider five cities in detail, looking at risks and previous disaster events. Our results offer a generalizable framework in evaluating the feasibility of coursing relief efforts within disaster-affected areas using land-based transportation methods. They also provide, albeit in retrospect, a glimpse of the time difficulties which occurred, and the stakes of life involved in the humanitarian crisis which developed in the Kathmandu area due to the earthquakes of April and May 2015.
更多查看译文
关键词
damage response,network features,urban
AI 理解论文
溯源树
样例
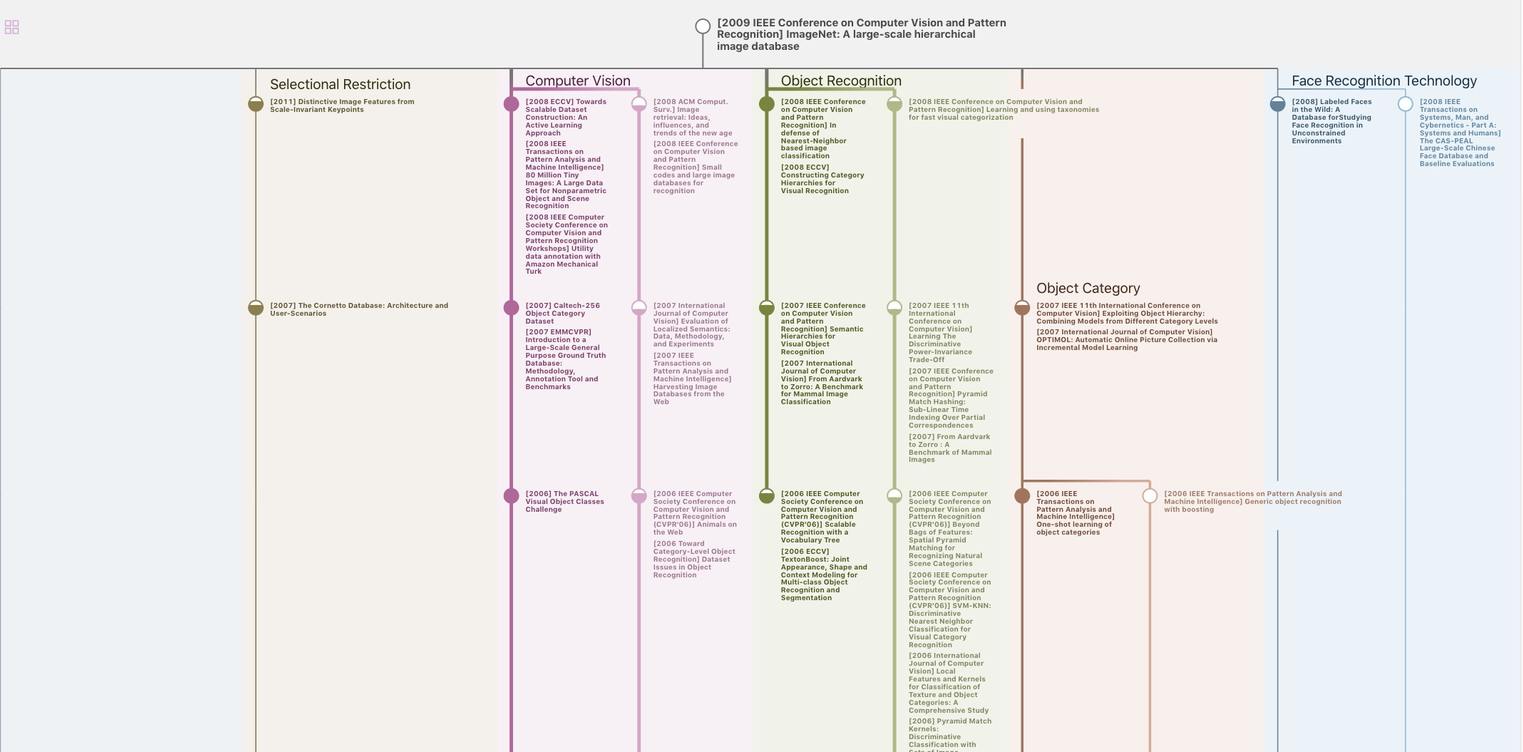
生成溯源树,研究论文发展脉络
Chat Paper
正在生成论文摘要