Early Time-Series Classification Algorithms: An Empirical Comparison
arxiv(2022)
摘要
Early Time-Series Classification (ETSC) is the task of predicting the class of incoming time-series by observing as few measurements as possible. Such methods can be employed to obtain classification forecasts in many time-critical applications. However, available techniques are not equally suitable for every problem, since differentiations in the data characteristics can impact algorithm performance in terms of earliness, accuracy, F1-score, and training time. We evaluate six existing ETSC algorithms on publicly available data, as well as on two newly introduced datasets originating from the life sciences and maritime domains. Our goal is to provide a framework for the evaluation and comparison of ETSC algorithms and to obtain intuition on how such approaches perform on real-life applications. The presented framework may also serve as a benchmark for new related techniques.
更多查看译文
关键词
classification,algorithms,time-series
AI 理解论文
溯源树
样例
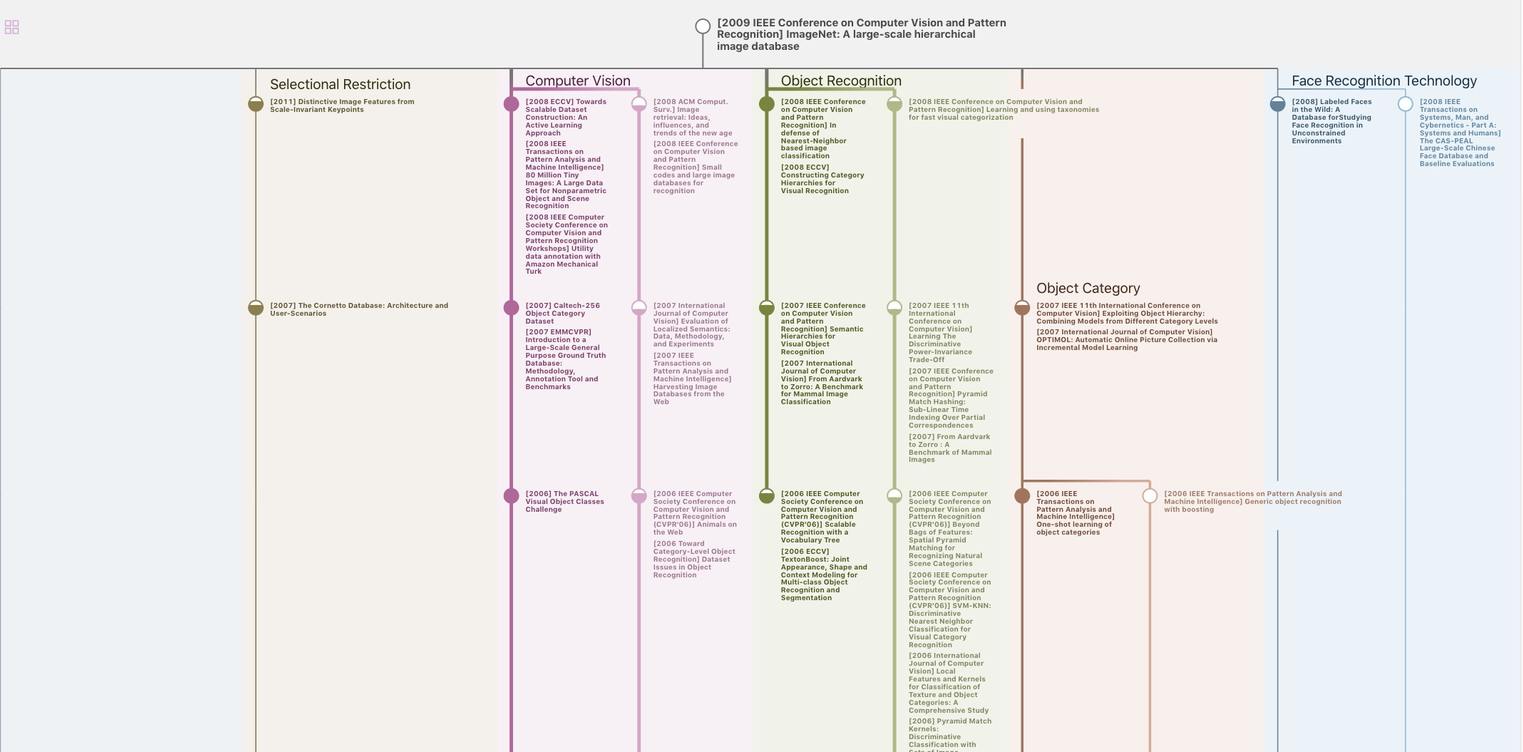
生成溯源树,研究论文发展脉络
Chat Paper
正在生成论文摘要