QaNER: Prompting Question Answering Models for Few-shot Named Entity Recognition
arxiv(2022)
摘要
Recently, prompt-based learning for pre-trained language models has succeeded in few-shot Named Entity Recognition (NER) by exploiting prompts as task guidance to increase label efficiency. However, previous prompt-based methods for few-shot NER have limitations such as a higher computational complexity, poor zero-shot ability, requiring manual prompt engineering, or lack of prompt robustness. In this work, we address these shortcomings by proposing a new prompt-based learning NER method with Question Answering (QA), called QaNER. Our approach includes 1) a refined strategy for converting NER problems into the QA formulation; 2) NER prompt generation for QA models; 3) prompt-based tuning with QA models on a few annotated NER examples; 4) zero-shot NER by prompting the QA model. Comparing the proposed approach with previous methods, QaNER is faster at inference, insensitive to the prompt quality, and robust to hyper-parameters, as well as demonstrating significantly better low-resource performance and zero-shot capability.
更多查看译文
关键词
prompting question answering models,question answering,entity
AI 理解论文
溯源树
样例
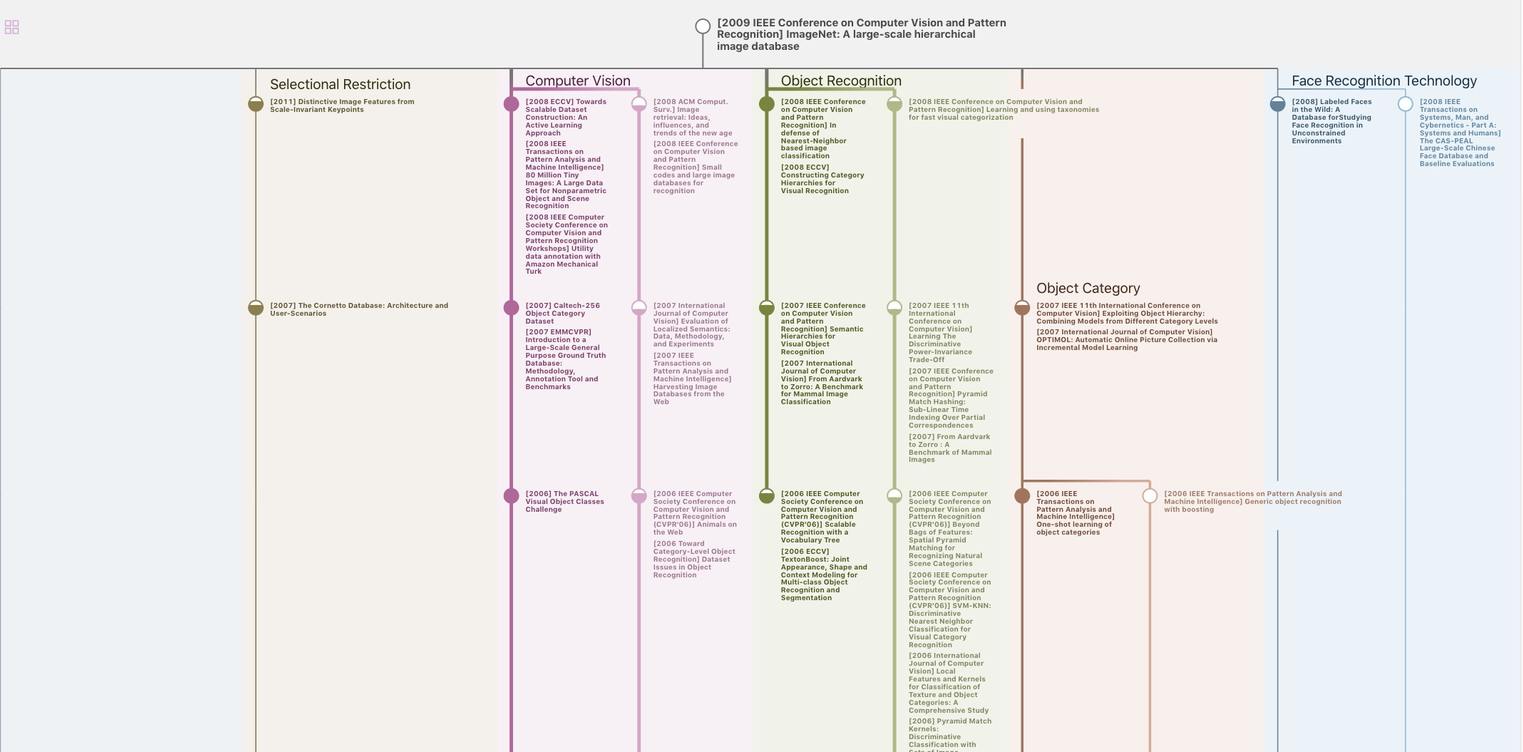
生成溯源树,研究论文发展脉络
Chat Paper
正在生成论文摘要