GNN-based end-to-end reconstruction in the CMS Phase 2 High-Granularity Calorimeter
arxiv(2023)
摘要
We present the current stage of research progress towards a one-pass, completely Machine Learning (ML) based imaging calorimeter reconstruction. The model used is based on Graph Neural Networks (GNNs) and directly analyzes the hits in each HGCAL endcap. The ML algorithm is trained to predict clusters of hits originating from the same incident particle by labeling the hits with the same cluster index. We impose simple criteria to assess whether the hits associated as a cluster by the prediction are matched to those hits resulting from any particular individual incident particles. The algorithm is studied by simulating two tau leptons in each of the two HGCAL endcaps, where each tau may decay according to its measured standard model branching probabilities. The simulation includes the material interaction of the tau decay products which may create additional particles incident upon the calorimeter. Using this varied multiparticle environment we can investigate the application of this reconstruction technique and begin to characterize energy containment and performance.
更多查看译文
关键词
cms phase,gnn-based,end-to-end,high-granularity
AI 理解论文
溯源树
样例
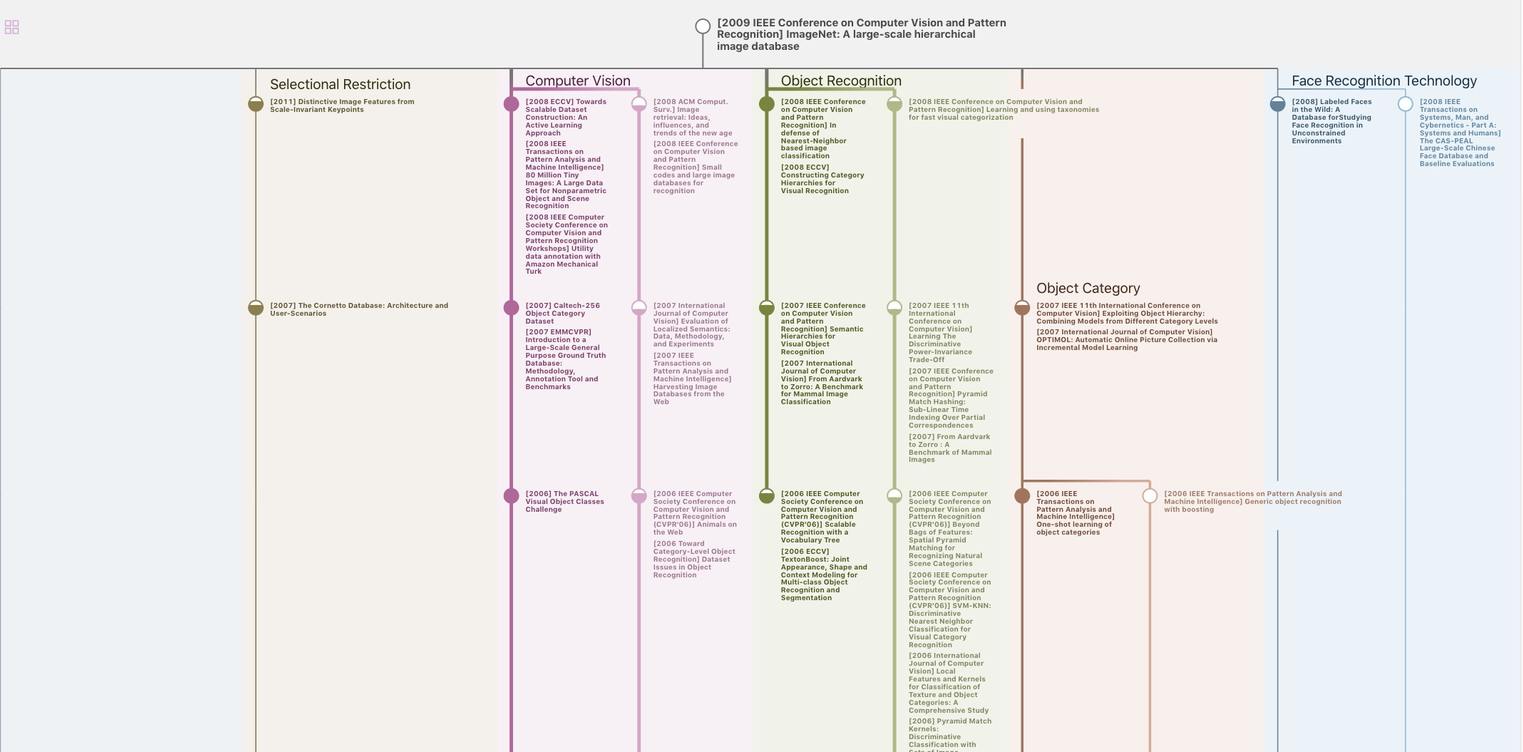
生成溯源树,研究论文发展脉络
Chat Paper
正在生成论文摘要