Follow your Nose: Using General Value Functions for Directed Exploration in Reinforcement Learning
arxiv(2023)
摘要
Improving sample efficiency is a key challenge in reinforcement learning, especially in environments with large state spaces and sparse rewards. In literature, this is resolved either through the use of auxiliary tasks (subgoals) or through clever exploration strategies. Exploration methods have been used to sample better trajectories in large environments while auxiliary tasks have been incorporated where the reward is sparse. However, few studies have attempted to tackle both large scale and reward sparsity at the same time. This paper explores the idea of combining exploration with auxiliary task learning using General Value Functions (GVFs) and a directed exploration strategy. We present a way to learn value functions which can be used to sample actions and provide directed exploration. Experiments on navigation tasks with varying grid sizes demonstrate the performance advantages over several competitive baselines.
更多查看译文
关键词
directed exploration
AI 理解论文
溯源树
样例
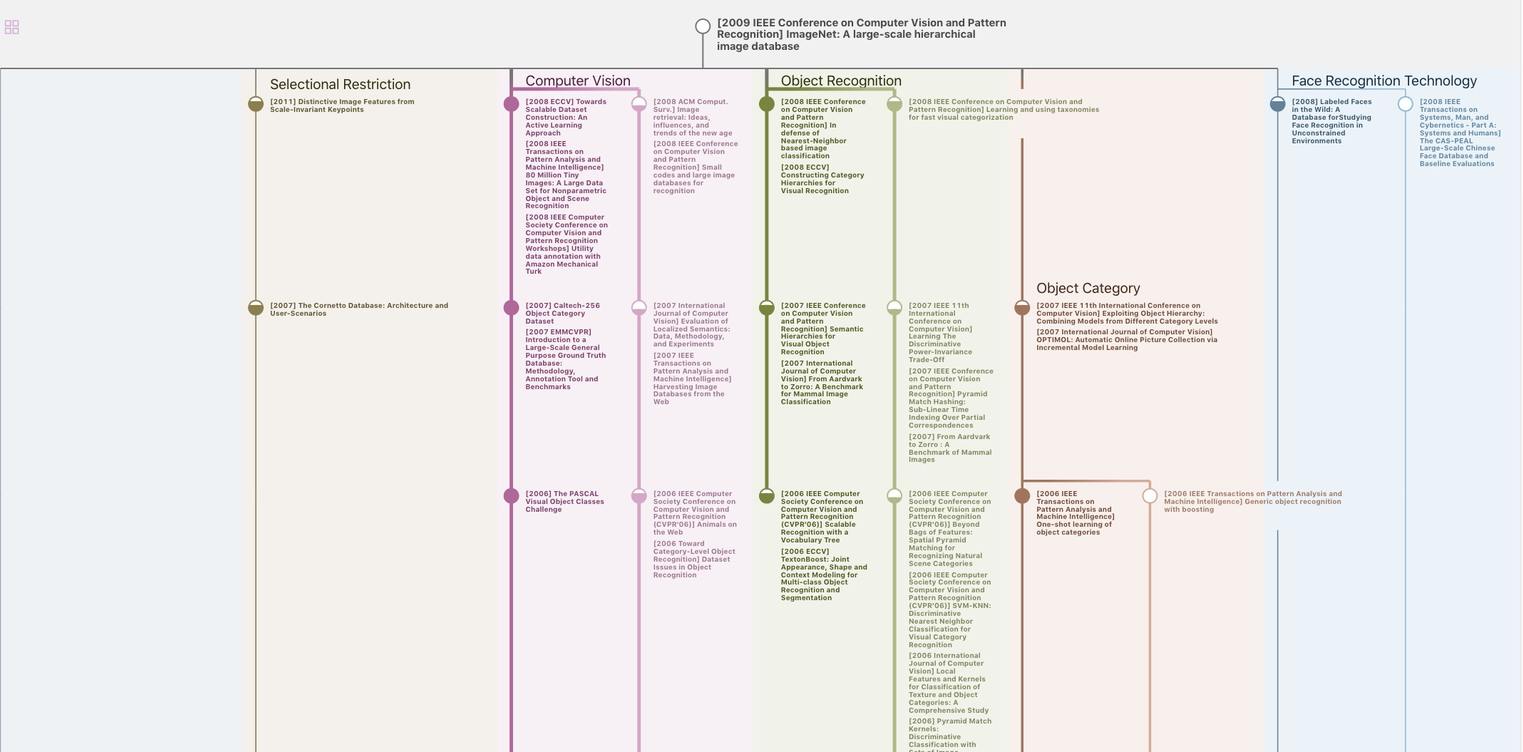
生成溯源树,研究论文发展脉络
Chat Paper
正在生成论文摘要