Scan-specific Self-supervised Bayesian Deep Non-linear Inversion for Undersampled MRI Reconstruction.
arxiv(2024)
摘要
Magnetic resonance imaging is subject to slow acquisition times due to the inherent limitations in data sampling. Recently, supervised deep learning has emerged as a promising technique for reconstructing sub-sampled MRI. However, supervised deep learning requires a large dataset of fully-sampled data. Although unsupervised or self-supervised deep learning methods have emerged to address the limitations of supervised deep learning approaches, they still require a database of images. In contrast, scan-specific deep learning methods learn and reconstruct using only the sub-sampled data from a single scan. Here, we introduce Scan-Specific Self-Supervised Bayesian Deep Non-Linear Inversion (DNLINV) that does not require an auto calibration scan region. DNLINV utilizes a Deep Image Prior-type generative modeling approach and relies on approximate Bayesian inference to regularize the deep convolutional neural network. We demonstrate our approach on several anatomies, contrasts, and sampling patterns and show improved performance over existing approaches in scan-specific calibrationless parallel imaging and compressed sensing.
更多查看译文
关键词
Bayesian deep learning,self-supervised deep learning,deep image prior,undersampled MRI,calibrationless MRI
AI 理解论文
溯源树
样例
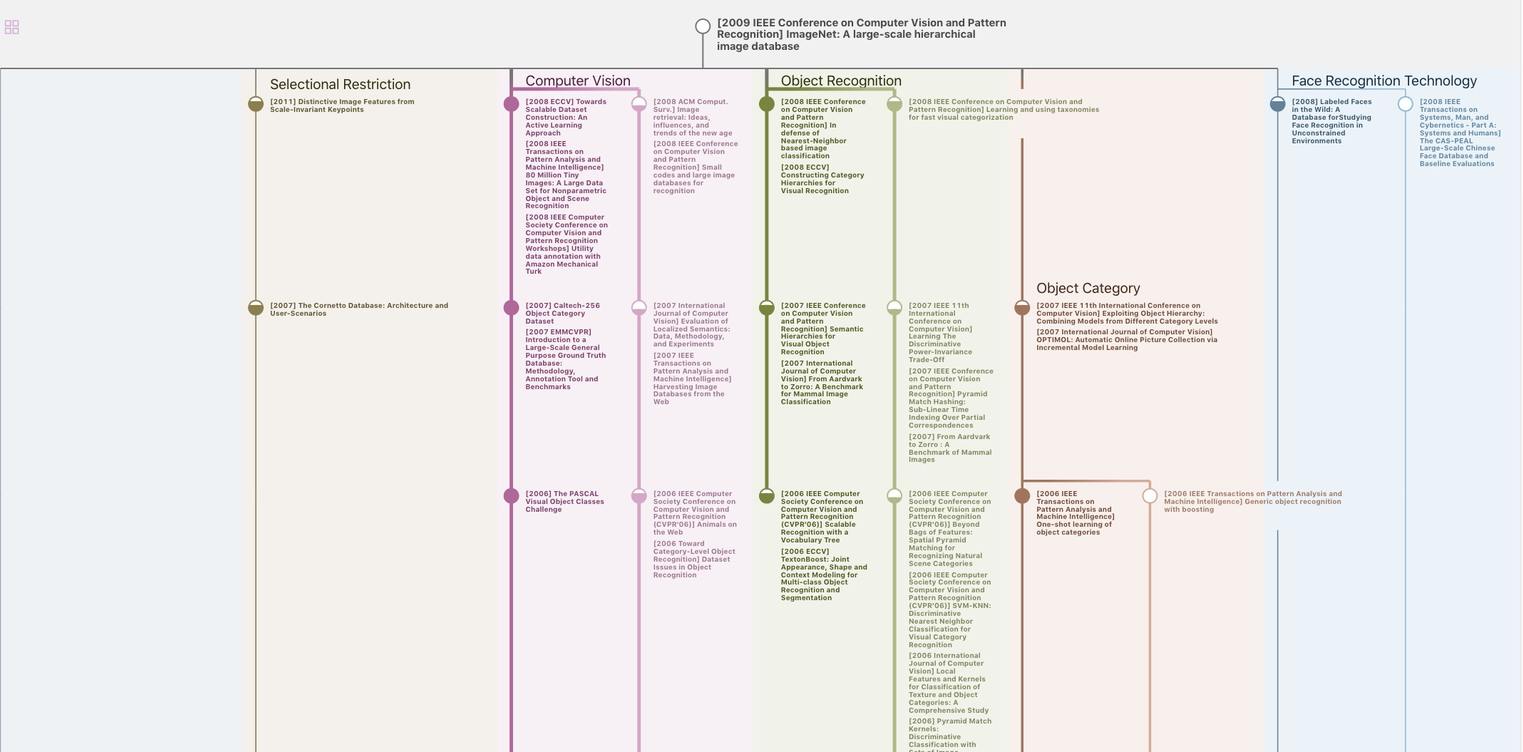
生成溯源树,研究论文发展脉络
Chat Paper
正在生成论文摘要