Assessment of human microbiota stability across longitudinal samples using iteratively growing-partitioned clustering
BRIEFINGS IN BIOINFORMATICS(2022)
摘要
Microbiome research is advancing rapidly, and every new study should definitively be based on updated methods, trends and milestones in this field to avoid the wrong interpretation of results. Most human microbiota surveys rely on data captured from snapshots-single data points from subjects-and have permitted uncovering the recognized interindividual variability and major covariates of such microbial communities. Currently, changes in individualized microbiota profiles are under the spotlight to serve as robust predictors of clinical outcomes (e.g. weight loss via dietary interventions) and disease anticipation. Therefore, novel methods are needed to provide robust evaluation of longitudinal series of microbiota data with the aim of assessing intrapersonally short-term to long-term microbiota changes likely linked to health and disease states. Consequently, we developed microbiota STability ASsessment via Iterative cluStering (mu STASIS)-a multifunction R package to evaluate individual-centered microbiota stability. mu STASIS targets the recognized interindividual variability inherent to microbiota data to stress the tight relationships observed among and characteristic of longitudinal samples derived from a single individual via iteratively growing-partitioned clustering. The algorithms and functions implemented in this framework deal properly with the sparse and compositional nature of microbiota data. Moreover, the resulting metric is intuitive and independent of beta diversity distance methods and correlation coefficients, thus estimating stability for each microbiota sample rather than giving nonconsensus magnitudes that are difficult to interpret within and between datasets. Our method is freely available under GPL-3 licensing. We demonstrate its utility by assessing gut microbiota stability from three independent studies published previously with multiple longitudinal series of multivariate data and respective metadata.
更多查看译文
关键词
microbiota stability, k-means, iterative clustering, individual variability, longitudinal sampling
AI 理解论文
溯源树
样例
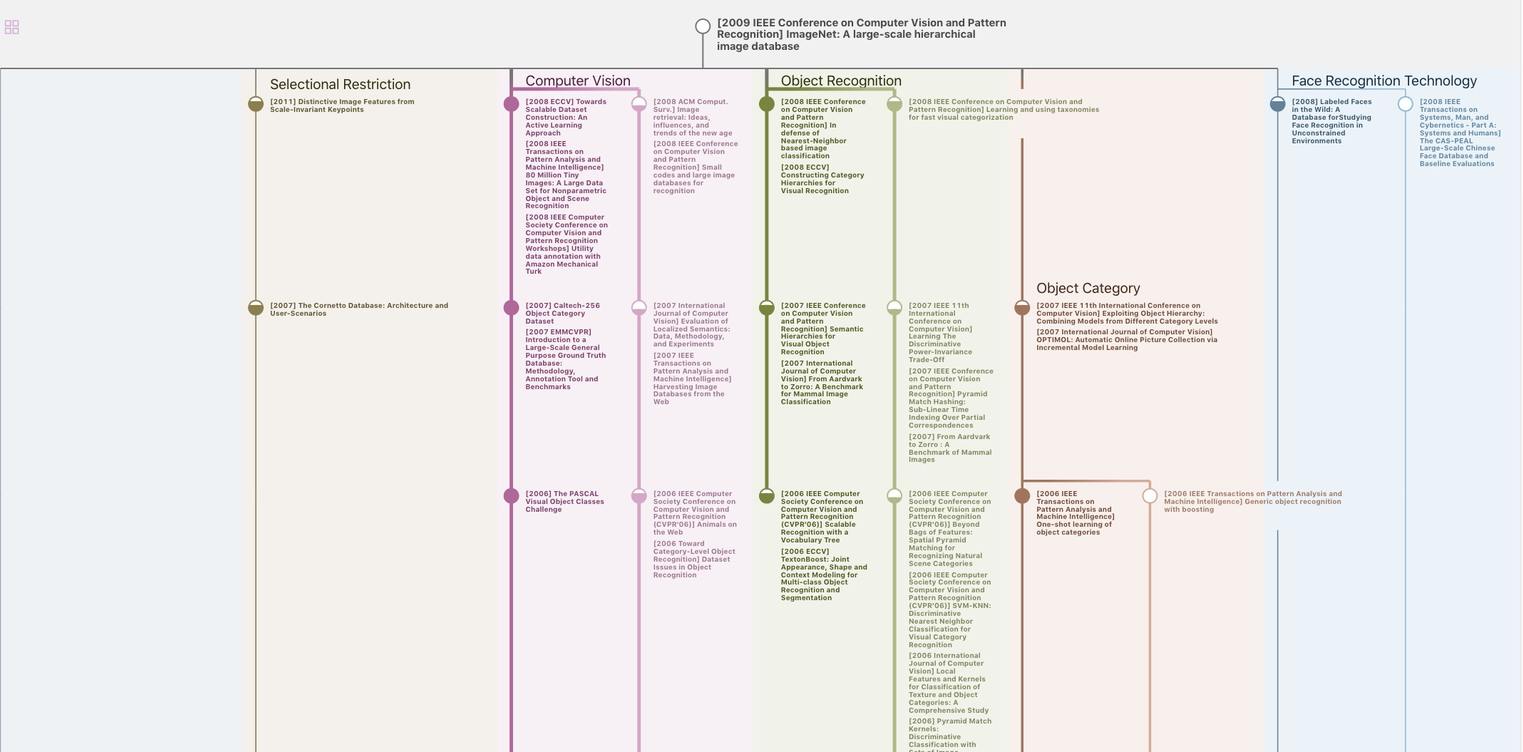
生成溯源树,研究论文发展脉络
Chat Paper
正在生成论文摘要