CPG-Actor : Reinforcement Learning for Central Pattern Generators
springer
摘要
Central Pattern Generators (CPGs) have several properties desirable for locomotion: they generate smooth trajectories, are robust to perturbations and are simple to implement. However, they are notoriously difficult to tune and commonly operate in an open-loop manner. This paper proposes a new methodology that allows tuning CPG controllers through gradient-based optimisation in a Reinforcement Learning (RL) setting. In particular, we show how CPGs can directly be integrated as the Actor in an Actor-Critic formulation. Additionally, we demonstrate how this change permits us to integrate highly non-linear feedback directly from sensory perception to reshape the oscillators’ dynamics. Our results on a locomotion task using a single-leg hopper demonstrate that explicitly using the CPG as the Actor rather than as part of the environment results in a significant increase in the reward gained over time (20\(\times \) more) compared with previous approaches. Finally, we demonstrate how our closed-loop CPG progressively improves the hopping behaviour for longer training epochs relying only on basic reward functions.
更多查看译文
关键词
Central Pattern Generators,Reinforcement Learning,Feedback control,Legged robots
AI 理解论文
溯源树
样例
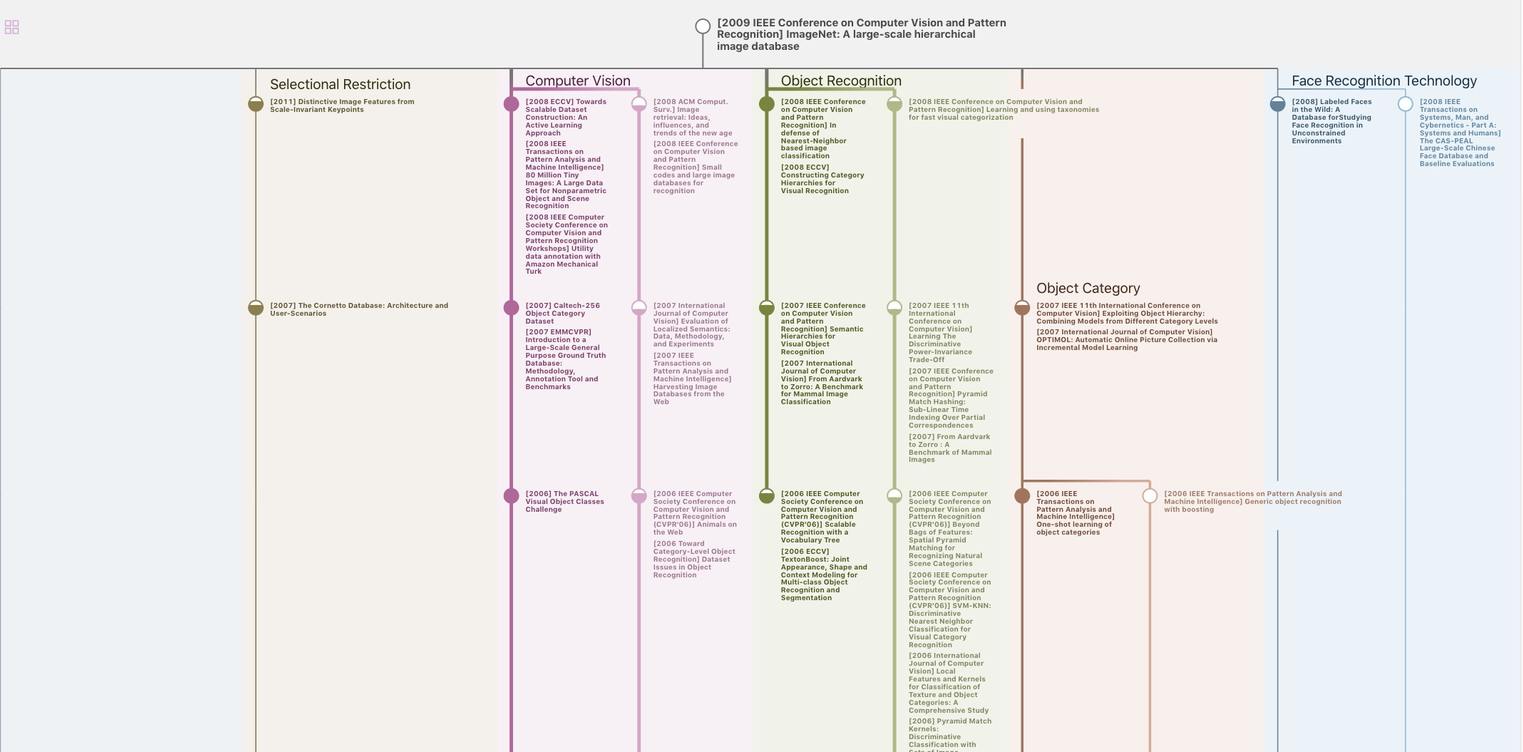
生成溯源树,研究论文发展脉络
Chat Paper
正在生成论文摘要