A Framework of Statistical Methods for Identification of Subgroups with Differential Treatment Effects in Randomized Trials
crossref
摘要
The problem of identifying subgroups of patients with differential treatment effects in randomized trials plays an important role in the effort to tailor therapies to patients who are most likely to get benefit from them. It has attracted active research effort in recent years, and a growing number of statistical methods have been developed. In this chapter, after first examining the major challenges with subgroup identification that these methods are designed to address, we create a structured framework into which many of the methods can be placed. Such a framework provides insight into the subgroup identification problem and methods, and can be utilized to generate additional methods from existing ones. Using a small simulation study, we also demonstrate a recently proposed approach to systematically evaluate the performance of subgroup identification methods. Together, the methodological framework and systematic assessment of performance can help to determine the optimal analyses for various applications.
更多查看译文
关键词
Predictive Marker, Average Treatment Effect, Recursive Partitioning, Random Forest Model, Differential Treatment Effect
AI 理解论文
溯源树
样例
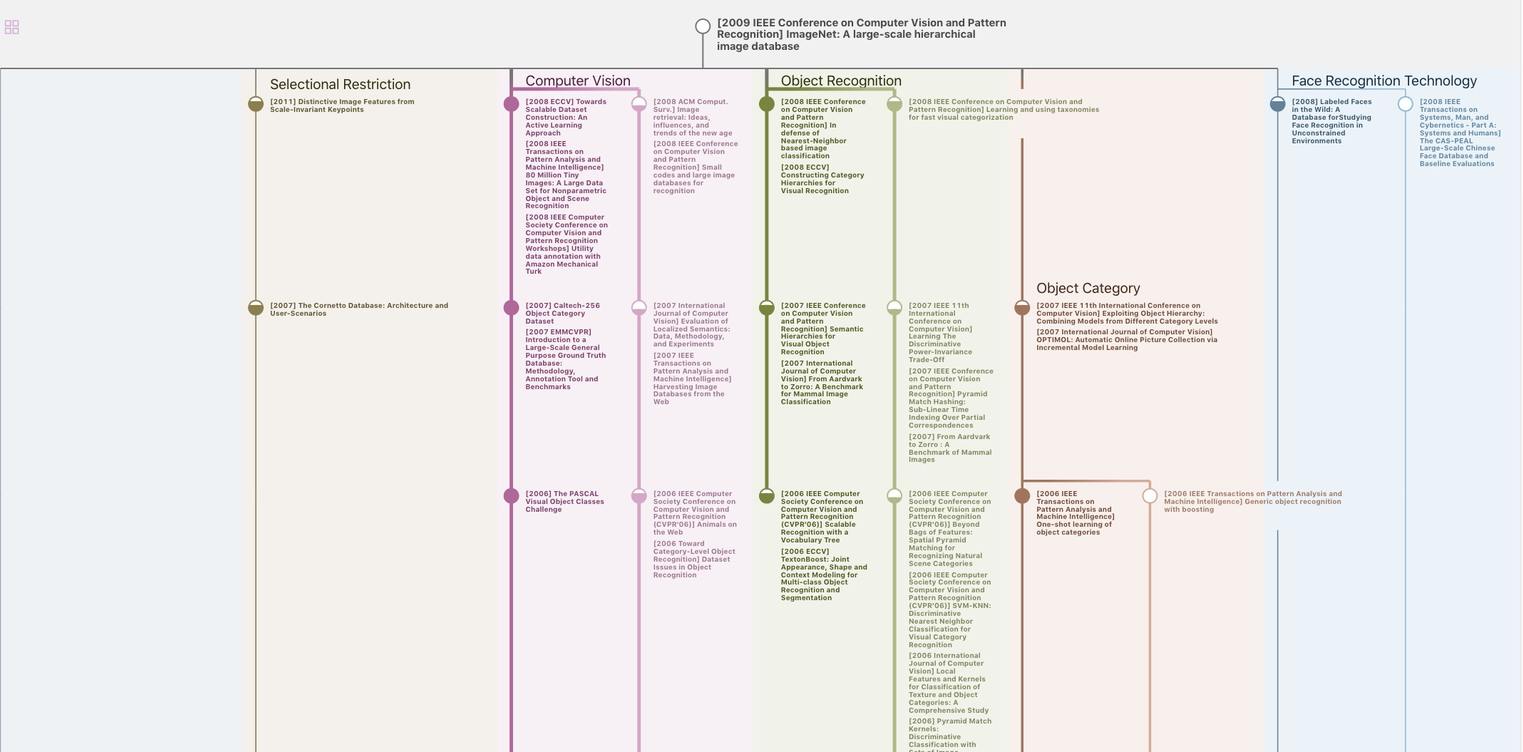
生成溯源树,研究论文发展脉络
Chat Paper
正在生成论文摘要