IGAN: Intrusion Detection Using Anomaly-Based Generative Adversarial Network
springer
摘要
We present an architecture, an Anomaly-based Generative Adversarial Network (IGAN), that detects malicious strings with decent accuracy. The proposed IGAN is comprised of an encoder, a decoder, and a discriminator. The encoder and decoder form the generative unit, trying to reconstruct the input and map that input and output to a latent space variable. IGAN exploits this latent space together with the adversarial training with the discriminator to enhance the learning of the normal distribution. The discriminator used is as a classifier as well as a feature extractor. To identify an anomaly or a normal instance, the anomaly score is calculated after passing it to the trained model. We have performed a detailed analysis of the existing and the proposed architecture using reliable metrics such as AUC score, Precision, Recall, and F_score. The experimental results of the proposed IGAN outperform other existing models in detecting anomalies with high accuracy.
更多查看译文
关键词
Anomaly detection, Autoencoders, Generative adversarial networks, Intrusion detection
AI 理解论文
溯源树
样例
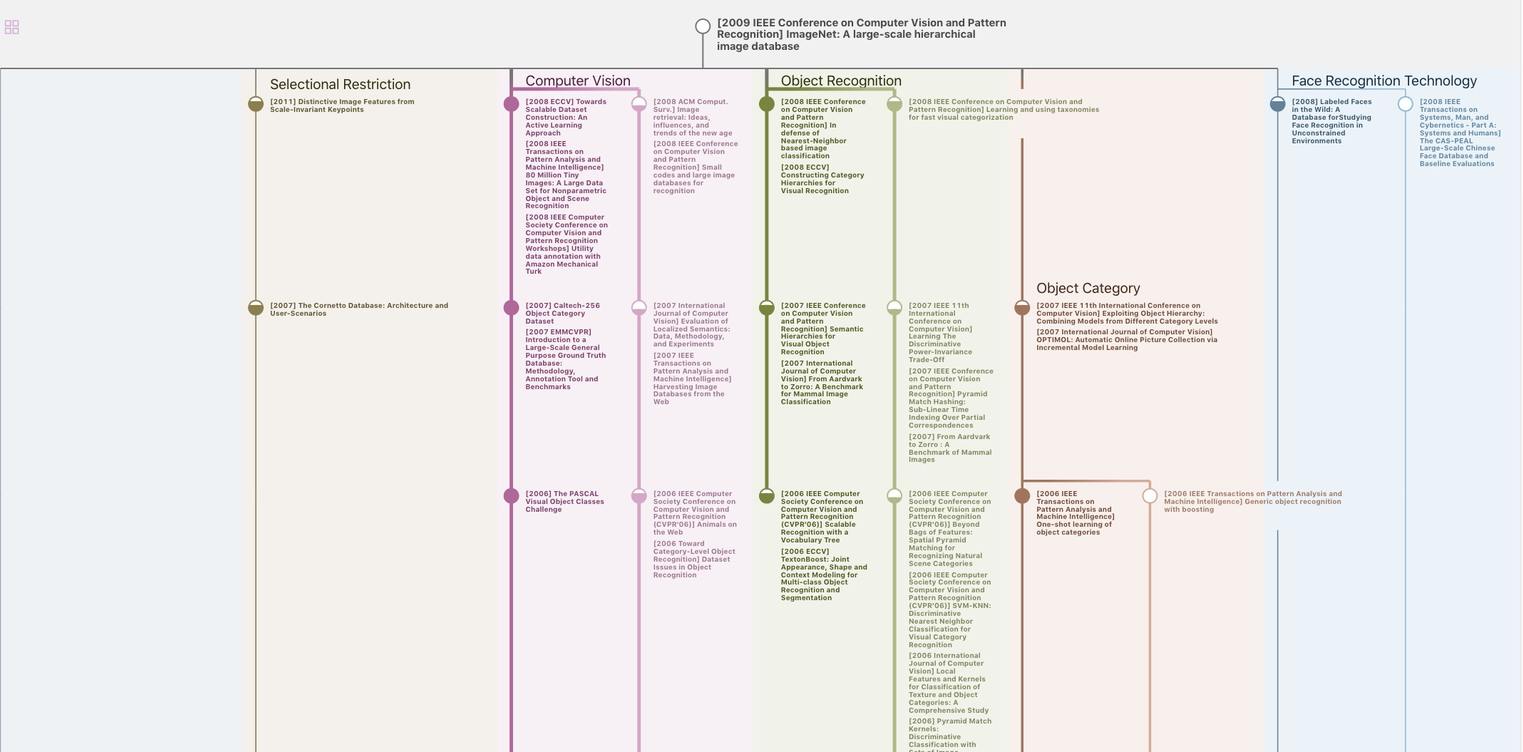
生成溯源树,研究论文发展脉络
Chat Paper
正在生成论文摘要