Unraveling Deep Learning Performance in Cross-Sensor Iris Recognition
Springer eBooks(2021)
摘要
Iris recognition has shown dominant characteristics in the field of biometrics, however, iris acquisition from heterogeneous sensors is still a key barrier in its wide-scale deployment. In the past few years, convolutional neural networks achieved substantially improved performance in the domain of computer vision and outset to employ for iris applications. This paper analyzes the performances of various preeminent deep learning models while realizing cross-sensor iris recognition. Their quantitative performances are analyzed based on the experiments performed over two public iris datasets; ND-CrossSensor-Iris-2013 and ND-iris-0405. The experimental results depict that these models have enough potential to transfer their knowledge to the domain of iris recognition and to recognize the microstructures within the iris region to unveil improved feature perception. Furthermore, a comparative analysis is carried out among these models based on five manually designed experiments regarding cross-sensor iris recognition, where the best performance is reported by the DenseNet model.
更多查看译文
关键词
Cross-sensor iris recognition, CNN customization, Knowledge transfer, Pre-trained models
AI 理解论文
溯源树
样例
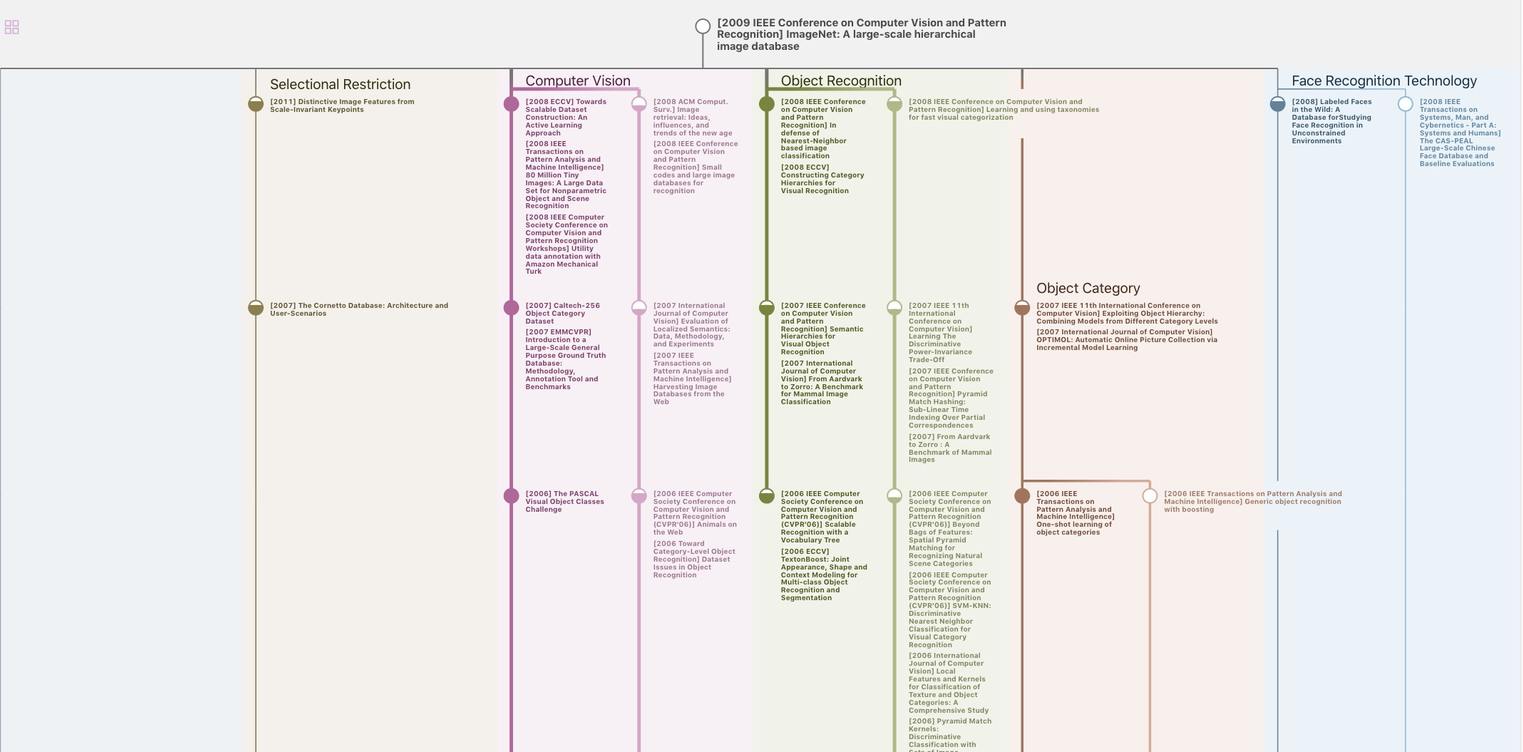
生成溯源树,研究论文发展脉络
Chat Paper
正在生成论文摘要