Computational Code-Based Privacy in Coded Federated Learning.
International Symposium on Information Theory (ISIT)(2022)
摘要
We propose a privacy-preserving federated learning (FL) scheme that is resilient against straggling devices. An adaptive scenario is suggested where the slower devices share their data with the faster ones and do not participate in the learning process. The proposed scheme employs code-based cryptography to ensure \emph{computational} privacy of the private data, i.e., no device with bounded computational power can obtain information about the other devices' data in feasible time. For a scenario with 25 devices, the proposed scheme achieves a speed-up of 4.7 and 4 for 92 and 128 bits security, respectively, for an accuracy of 95\% on the MNIST dataset compared with conventional mini-batch FL.
更多查看译文
关键词
coded federated learning,privacy,computational,code-based
AI 理解论文
溯源树
样例
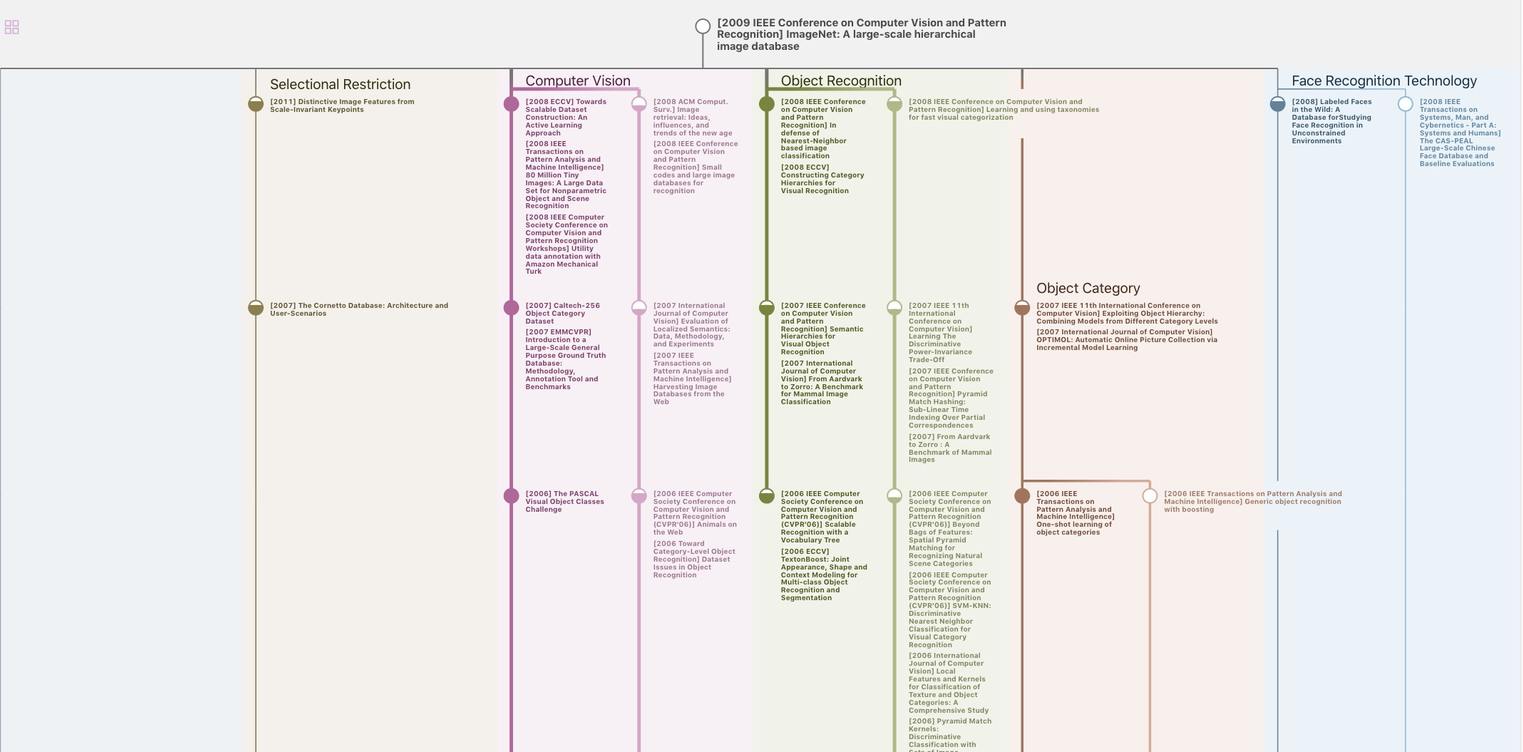
生成溯源树,研究论文发展脉络
Chat Paper
正在生成论文摘要