Local Intensity Order Transformation for Robust Curvilinear Object Segmentation
IEEE TRANSACTIONS ON IMAGE PROCESSING(2022)
摘要
Segmentation of curvilinear structures is important in many applications, such as retinal blood vessel segmentation for early detection of vessel diseases and pavement crack segmentation for road condition evaluation and maintenance. Currently, deep learning-based methods have achieved impressive performance on these tasks. Yet, most of them mainly focus on finding powerful deep architectures but ignore capturing the inherent curvilinear structure feature (e.g., the curvilinear structure is darker than the context) for a more robust representation. In consequence, the performance usually drops a lot on cross-datasets, which poses great challenges in practice. In this paper, we aim to improve the generalizability by introducing a novel local intensity order transformation (LIOT). Specifically, we transfer a gray-scale image into a contrast-invariant four-channel image based on the intensity order between each pixel and its nearby pixels along with the four (horizontal and vertical) directions. This results in a representation that preserves the inherent characteristic of the curvilinear structure while being robust to contrast changes. Cross-dataset evaluation on three retinal blood vessel segmentation datasets demonstrates that LIOT improves the generalizability of some state-of-the-art methods. Additionally, the cross-dataset evaluation between retinal blood vessel segmentation and pavement crack segmentation shows that LIOT is able to preserve the inherent characteristic of curvilinear structure with large appearance gaps. An implementation of the proposed method is available at https://github.com/TY-Shi/LIOT.
更多查看译文
关键词
Image segmentation, Transforms, Object segmentation, Retina, Biomedical imaging, Learning systems, Blood vessels, Curvilinear structure, segmentation, local intensity order, deep learning, generalizability
AI 理解论文
溯源树
样例
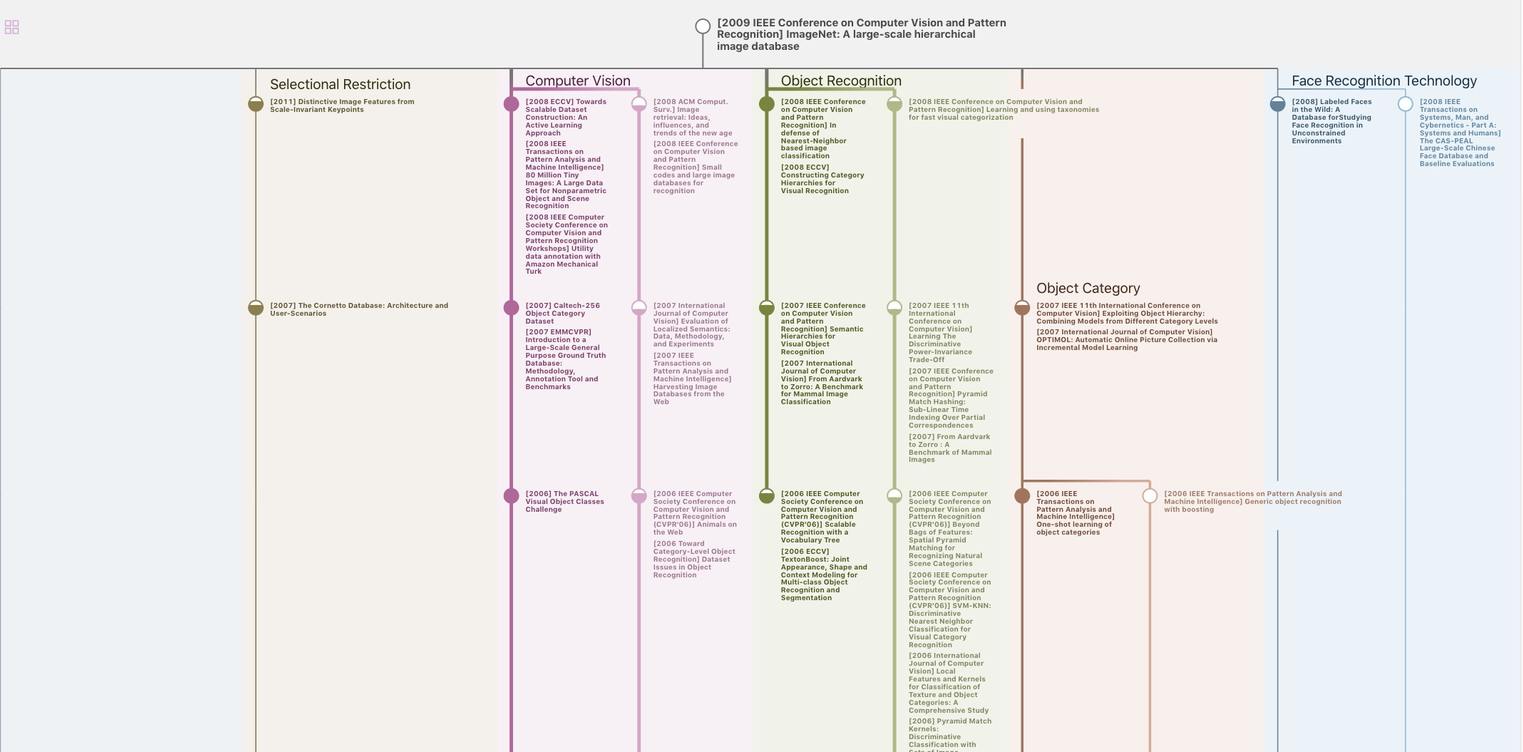
生成溯源树,研究论文发展脉络
Chat Paper
正在生成论文摘要