MUC-driven Feature Importance Measurement and Adversarial Analysis for Random Forest
arxiv(2022)
摘要
The broad adoption of Machine Learning (ML) in security-critical fields demands the explainability of the approach. However, the research on understanding ML models, such as Random Forest (RF), is still in its infant stage. In this work, we leverage formal methods and logical reasoning to develop a novel model-specific method for explaining the prediction of RF. Our approach is centered around Minimal Unsatisfiable Cores (MUC) and provides a comprehensive solution for feature importance, covering local and global aspects, and adversarial sample analysis. Experimental results on several datasets illustrate the high quality of our feature importance measurement. We also demonstrate that our adversarial analysis outperforms the state-of-the-art method. Moreover, our method can produce a user-centered report, which helps provide recommendations in real-life applications.
更多查看译文
关键词
feature importance measurement,adversarial analysis,forest,muc-driven
AI 理解论文
溯源树
样例
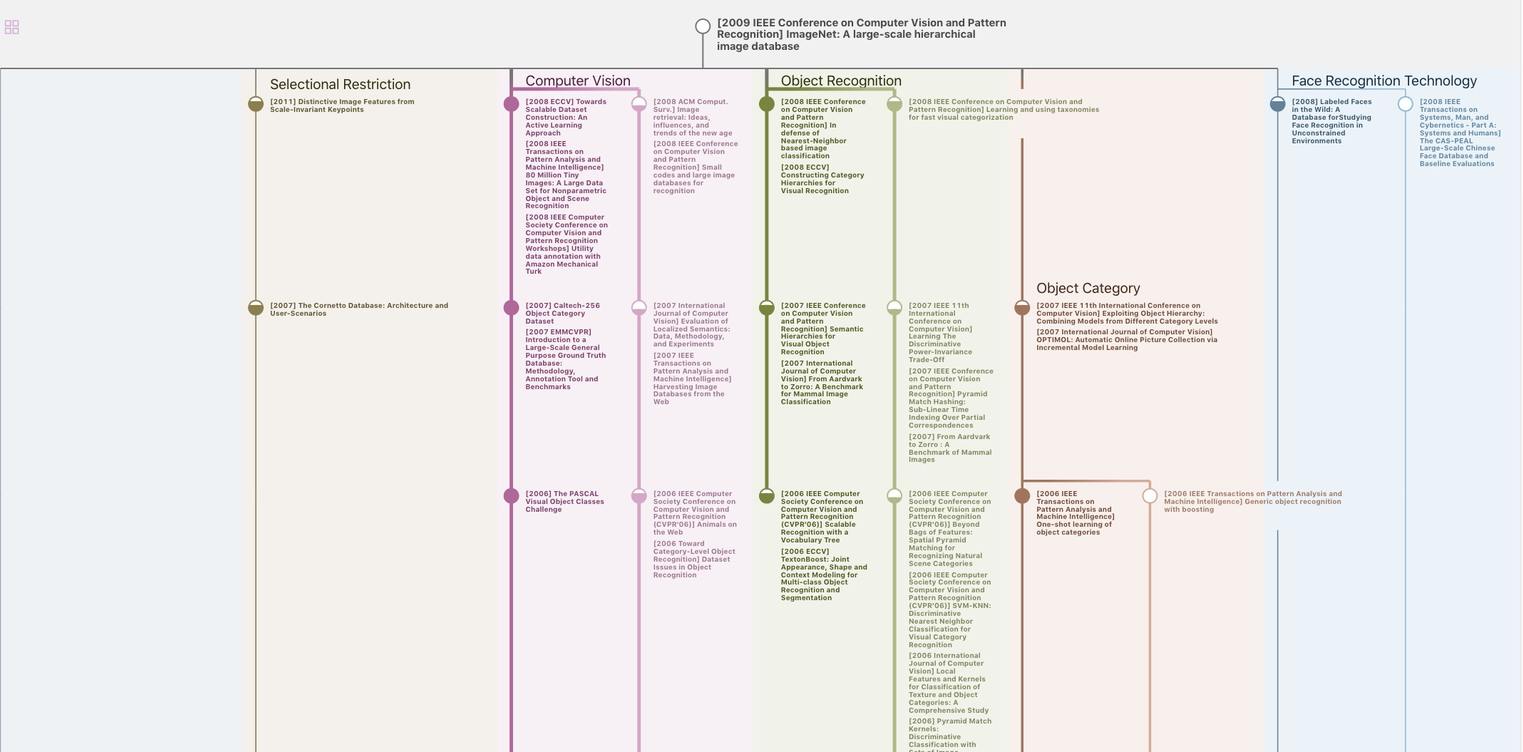
生成溯源树,研究论文发展脉络
Chat Paper
正在生成论文摘要