Differentially-Private Publication of Origin-Destination Matrices with Intermediate Stops
International Conference on Extending Database Technology (EDBT)(2022)
摘要
Conventional origin-destination (OD) matrices record the count of trips between pairs of start and end locations, and have been extensively used in transportation, traffic planning, etc. More recently, due to use case scenarios such as COVID-19 pandemic spread modeling, it is increasingly important to also record intermediate points along an individual's path, rather than only the trip start and end points. This can be achieved by using a multi-dimensional frequency matrix over a data space partitioning at the desired level of granularity. However, serious privacy constraints occur when releasing OD matrix data, and especially when adding multiple intermediate points, which makes individual trajectories more distinguishable to an attacker. To address this threat, we propose a technique for privacy-preserving publication of multi-dimensional OD matrices that achieves differential privacy (DP), the de-facto standard in private data release. We propose a family of approaches that factor in important data properties such as data density and homogeneity in order to build OD matrices that provide provable protection guarantees while preserving query accuracy. Extensive experiments on real and synthetic datasets show that the proposed approaches clearly outperform existing state-of-the-art.
更多查看译文
关键词
matrices,differentially-private,origin-destination
AI 理解论文
溯源树
样例
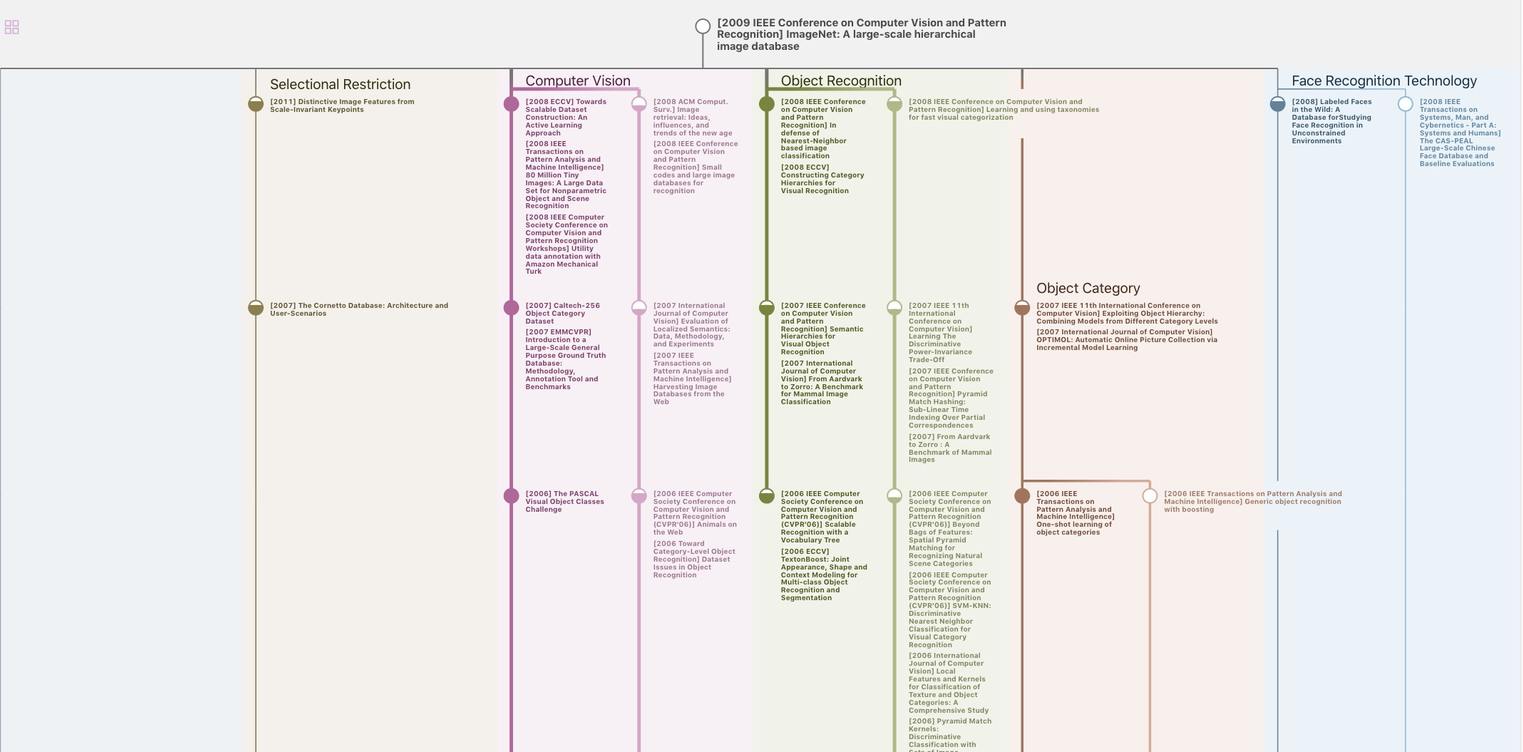
生成溯源树,研究论文发展脉络
Chat Paper
正在生成论文摘要