Improved Position Estimation Algorithm of Agricultural Mobile Robots Based on Multisensor Fusion and Autoencoder Neural Network
SENSORS(2022)
摘要
High-precision position estimations of agricultural mobile robots (AMRs) are crucial for implementing control instructions. Although the global navigation satellite system (GNSS) and real-time kinematic GNSS (RTK-GNSS) provide high-precision positioning, the AMR accuracy decreases when the signals interfere with buildings or trees. An improved position estimation algorithm based on multisensor fusion and autoencoder neural network is proposed. The multisensor, RTK-GNSS, inertial-measurement-unit, and dual-rotary-encoder data are fused with Extended Kalman filter (EKF). To optimize the EKF noise matrix, the autoencoder and radial basis function (ARBF) neural network was used for modeling the state equation noise and EKF measurement equation. A multisensor AMR test platform was constructed for static experiments to estimate the circular error probability and twice-the-distance root-mean-squared criteria. Dynamic experiments were conducted on road, grass, and field environments. To validate the robustness of the proposed algorithm, abnormal working conditions of the sensors were tested on the road. The results showed that the positioning estimation accuracy was improved compared to the RTK-GNSS in all three environments. When the RTK-GNSS signal experienced interference or rotary encoders failed, the system could still improve the position estimation accuracy. The proposed system and optimization algorithm are thus significant for improving AMR position prediction performance.
更多查看译文
关键词
Kalman filter (KF), inertial measurement unit (IMU), global navigation satellite system (GNSS), autoencoder neural network, agricultural mobile robots (AMRs)
AI 理解论文
溯源树
样例
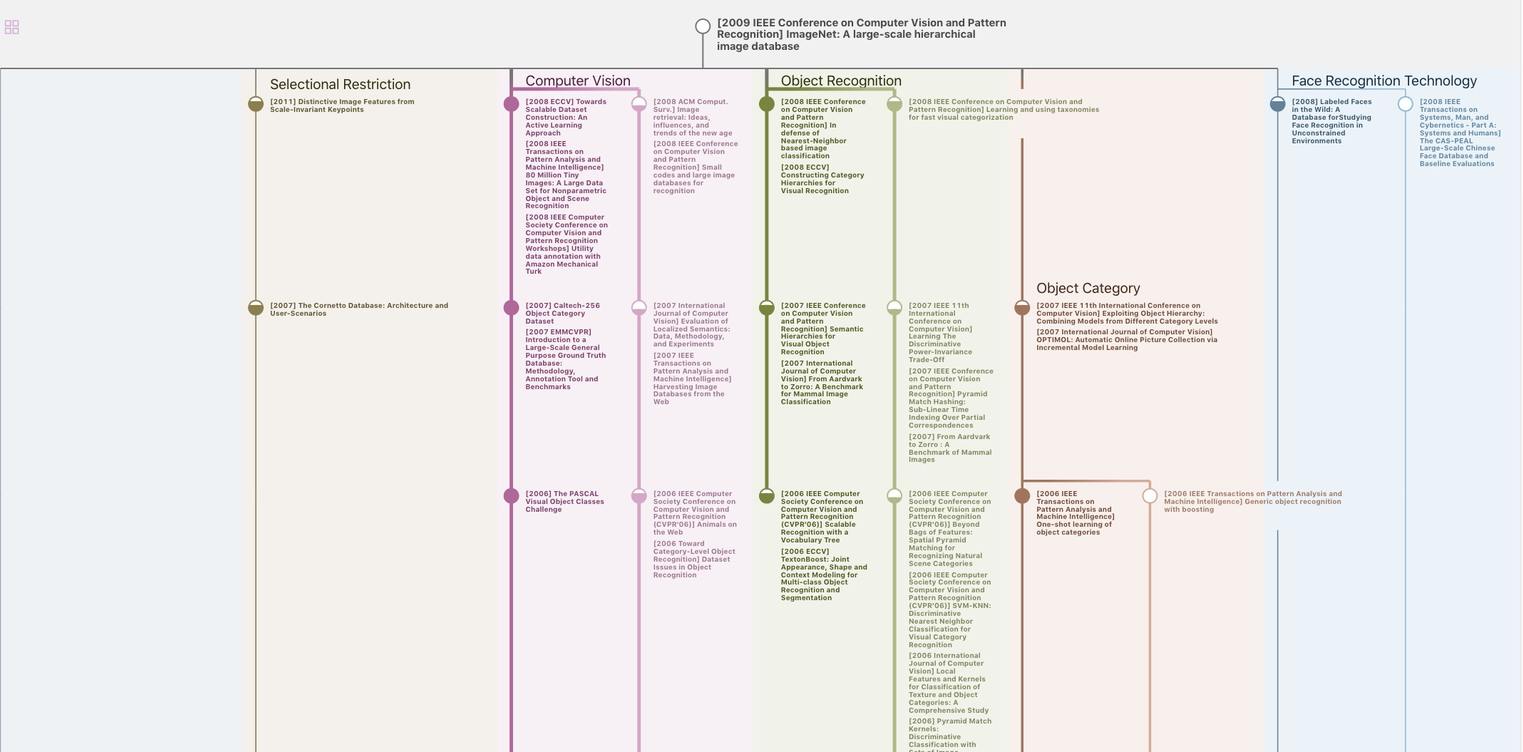
生成溯源树,研究论文发展脉络
Chat Paper
正在生成论文摘要