Evaluating bias control strategies in observational studies using frequentist model averaging
JOURNAL OF BIOPHARMACEUTICAL STATISTICS(2022)
摘要
Estimating a treatment effect from observational data requires modeling treatment and outcome subject to uncertainty/misspecification. A previous research has shown that it is not possible to find a uniformly best strategy. In this article we propose a novel Frequentist Model Averaging (FMA) framework encompassing any estimation strategy and accounting for model uncertainty by computing a cross-validated estimate of Mean Squared Prediction Error (MSPE). We present a simulation study with data mimicking an observational database. Model averaging over 15+ strategies was compared with individual strategies as well as the best strategy selected by minimum MSPE. FMA showed robust performance (Bias, Mean Squared Error (MSE), and Confidence Interval (CI) coverage). Other strategies, such as linear regression, did well in simple scenarios but were inferior to the FMA in a scenario with complex confounding.
更多查看译文
关键词
Model averaging, selection bias, confounding, cross-validation, model uncertainty
AI 理解论文
溯源树
样例
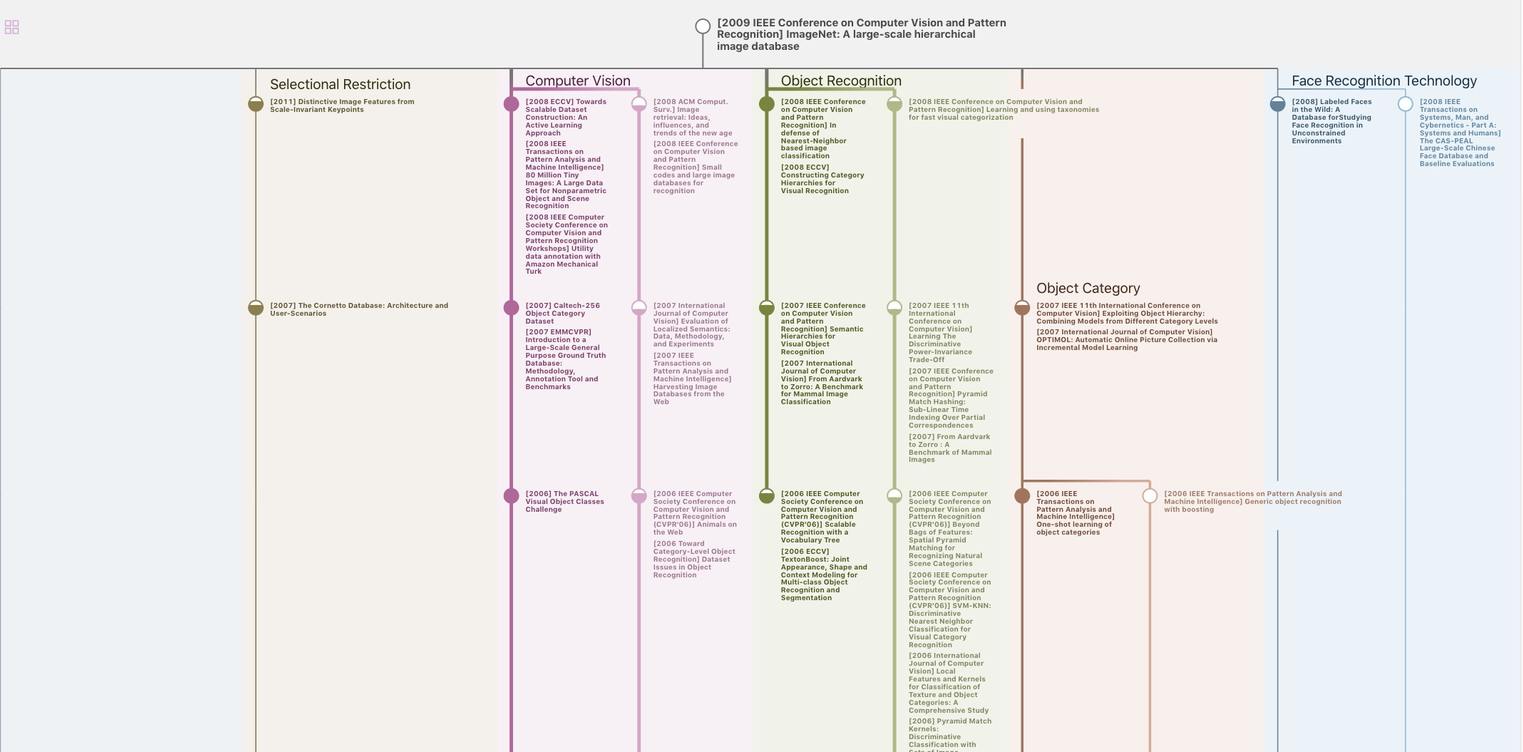
生成溯源树,研究论文发展脉络
Chat Paper
正在生成论文摘要