(Invited) Capturing Biological Behavior in Nanomagnetic Artificial Neurons and Synapses for Energy-Efficient Neuromorphic Computing
ECS Meeting Abstracts(2020)
摘要
Modeling computer architecture after the human brain is a promising approach to the ever-increasing demands for real-time processing of massive amounts of data. But, the building blocks of the brain, i.e. neurons and synapses, have more detailed behavior than simply how they are connected, and this behavior is central to their computing efficiency. If we want to capture the energy efficiency of such a neuromorphic architecture, we need devices themselves that can capture the behavior of the biological elements. Magnetic materials and devices have many properties similar to the brain that make them attractive candidates as artificial neurons and synapses. We will present our recent work on designing and modeling three-terminal domain wall-magnetic tunnel junctions (DW-MTJs) that can act as both leaky, integrate, and fire neurons with inherent lateral inhibition [1-2] and as synapses with online learning and spike-timing dependent plasticity behavior [3]. The DW-MTJ neuron consists of a perpendicularly magnetized wire containing a single DW and an MTJ on top of the wire, shown in Fig. a. When current is applied to the wire, the DW propagates through spin transfer torque (STT) or spin orbit torque (SOT). The MTJ defines the firing point of the neuron: when the DW moves past the junction, the MTJ resistance switches to generate a spiking current at the MTJ output terminal. We will show results of integration, firing, and inherent leaking of the device. When assembled into a crossbar array as shown in Fig. b, 1-10 digit identification is simulated by measuring which of ten neurons reach the firing point first as shown in Fig. c. Lateral inhibition (LI) is an important function in neuromorphic computing, modeled after the biological neuron behavior that a neuron prevents its less active neighbors in the same layer from firing. In most neuromorphic hardware platforms, LI is implemented with external circuitry, thereby decreasing the energy efficiency and increasing the area overhead of such systems. We show that the DW-MTJ neuron has inherent LI via magnetic interactions. We will show results from modeling current- and field-driven DW motion in adjacent DW-MTJ neurons. Figure d shows that with 90 nm spacing between two 50 nm wide DW-MTJs, the slower device’s DW velocity is drastically reduced from 75 m/s to 20 m/s and a LI of 75% is achieved. The results are explained by current-driven DW velocity characteristics in response to external magnetic fields and are quantified by an analytical model based on the Landau-Lifshitz-Gilbert (LLG) equation. We will show results of arrays of DW-MTJs implementing a soft winner-take-all function and application of the effect to image recognition. We will furthermore present simulation results of the DW-MTJ showing synaptic behavior when the MTJ is made long. By constructing a small circuit around the device we implement spike-timing-dependent plasticity (STDP), where the current flowing through the synapse, which will determine its resistive synaptic weight, is determined by the delay between input and output neurons (Fig. e). We will show that the STDP synapses can process time-dependent input data. The results will be compared with other emerging nonvolatile devices for artificial neural networks. The work presented shows that DW-MTJs can provide a monolithic platform for neuromorphic computing circuits, where both synapses and neurons can be made from the same thin film stack, and motivate further study of magnetic devices for neuromorphic computing. SNL is managed and operated by NTESS under DOE NNSA contract DE-NA0003525. References N. Hassan, X. Hu, L. Jiang-Wei, W. H. Brigner, O. G. Akinola, F. Garcia-Sanchez, M. Pasquale, C. H. Bennett, J. A. C. Incorvia & J. S. Friedman. Magnetic domain wall neuron with lateral inhibition. J. Appl. Phys. 124, (2018). C. Cui, O. G. Akinola, N. Hassan, H. Christopher, M. J. Marinella, J. S. Friedman & J. A. C. Incorvia. Maximized Lateral Inhibition in Paired Magnetic Domain Wall Racetracks for Neuromorphic Computing. IOP Nanotechnology (2020). O. G. Akinola, X. Hu, C. H. Bennett, M. Marinella, J. S. Friedman & J. A. C. Incorvia. Three-terminal magnetic tunnel junction synapse circuits showing spike-timing-dependent plasticity. J. Phys. D. Appl. Phys. 52, 49LT01 (2019). Figure 1
更多查看译文
AI 理解论文
溯源树
样例
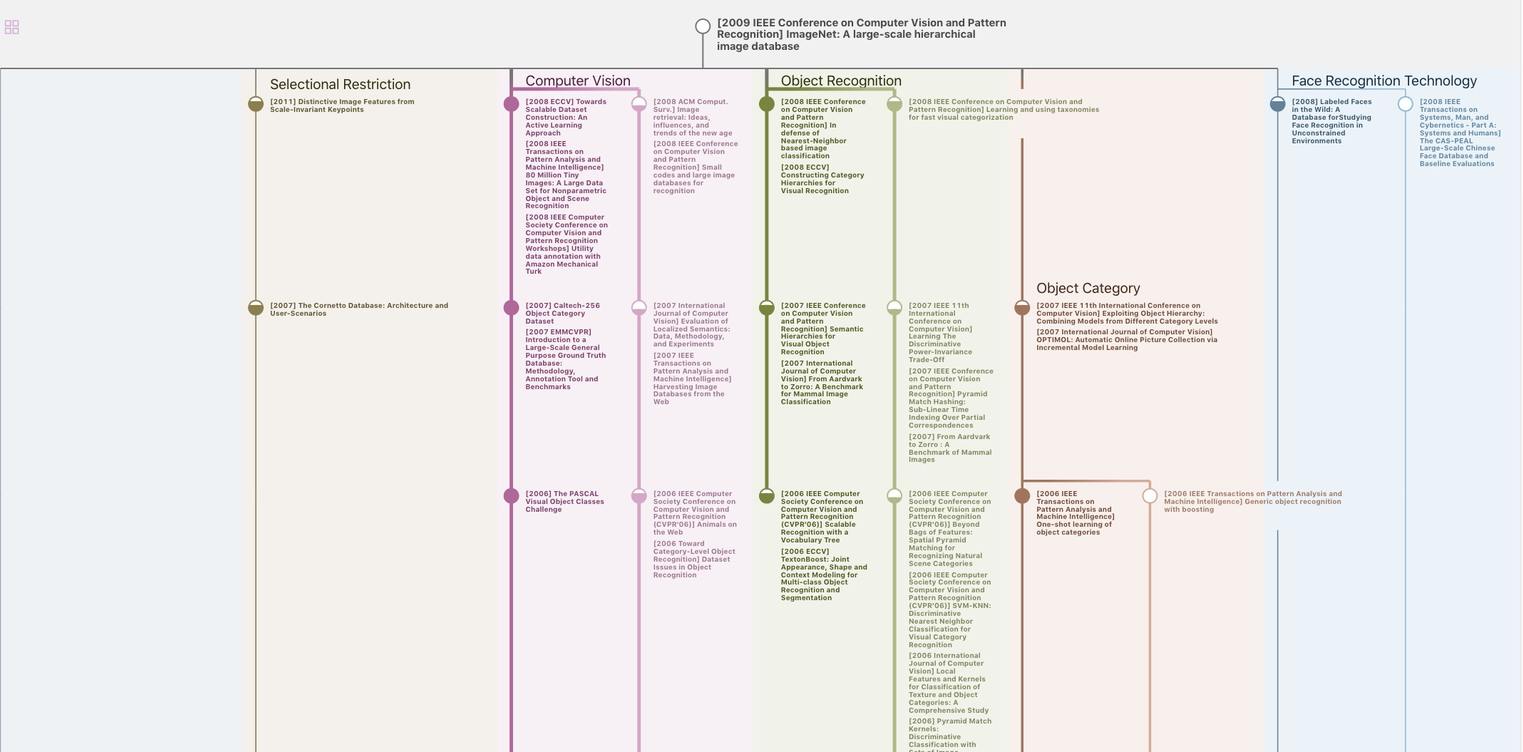
生成溯源树,研究论文发展脉络
Chat Paper
正在生成论文摘要