Privacy-Preserving Personalized Revenue Management
SSRN Electronic Journal(2020)
摘要
This paper examines how data-driven personalized decisions can be made while preserving consumer privacy. Our setting is one in which the firm chooses a personalized price based on each new customer's vector of individual features; the true set of individual demand-generating parameters is unknown to the firm and so must be estimated from historical data. We extend the existing personalized pricing framework by requiring also that the firm's pricing policy preserve consumer privacy, or (formally) that it be differentially private: an industry standard for privacy preservation. We develop privacy-preserving personalized pricing algorithms and show that they achieve near-optimal revenue by deriving theoretical (upper and lower) performance bounds. Our analyses further suggest that, if the firm possesses a sufficient amount of historical data, then it can achieve a certain level of differential privacy almost "for free." That is, the revenue loss due to privacy preservation is of smaller order than that due to estimation. We confirm our theoretical findings in a series of numerical experiments based on synthetically generated and online auto lending (CPRM-12-001) data sets. Finally, motivated by practical considerations, we also extend our algorithms and findings to a variety of alternative settings, including multiproduct pricing with substitution effect, discrete feasible price set, categorical sensitive features, and personalized assortment optimization.
更多查看译文
关键词
privacy, revenue management, data-driven decision making, personalized pricing, assortment optimization
AI 理解论文
溯源树
样例
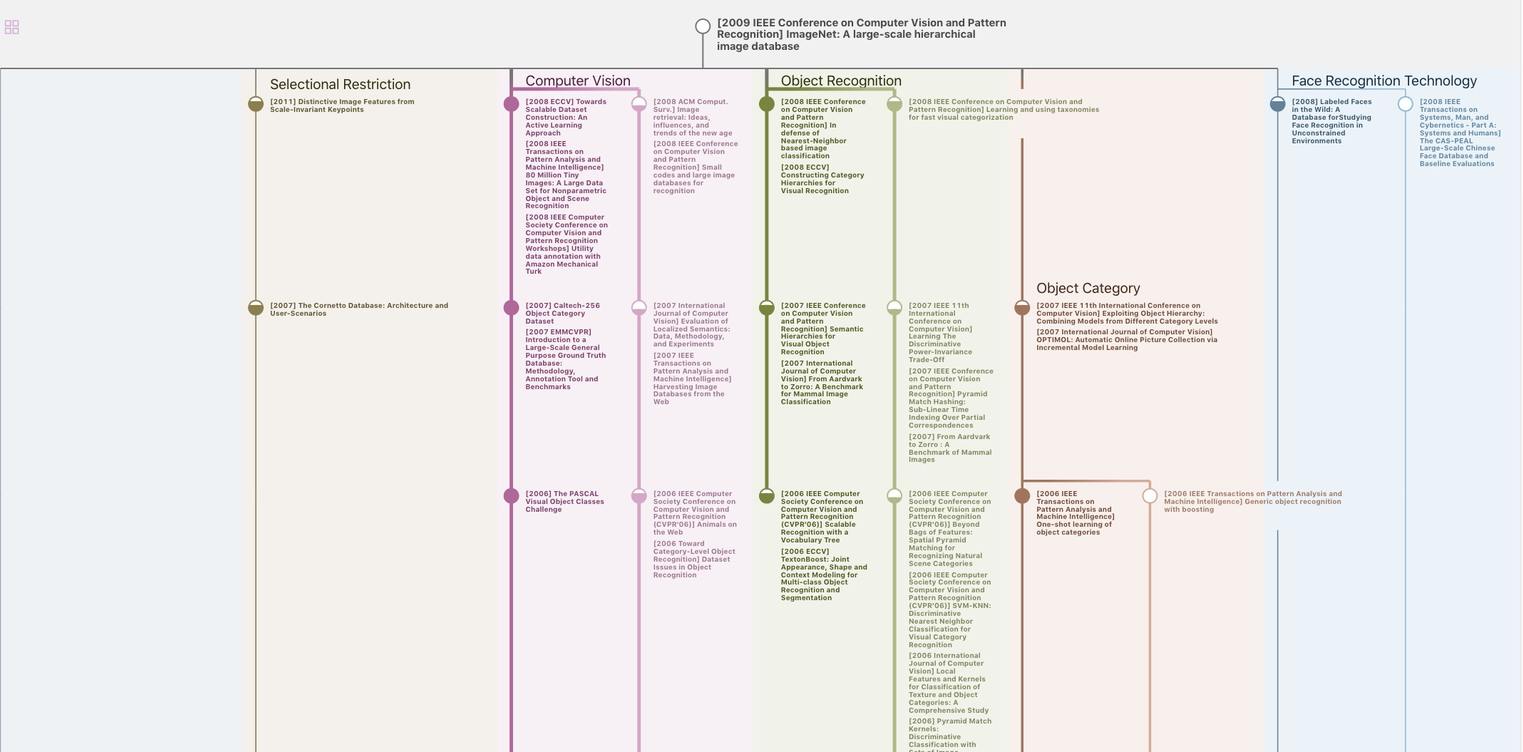
生成溯源树,研究论文发展脉络
Chat Paper
正在生成论文摘要